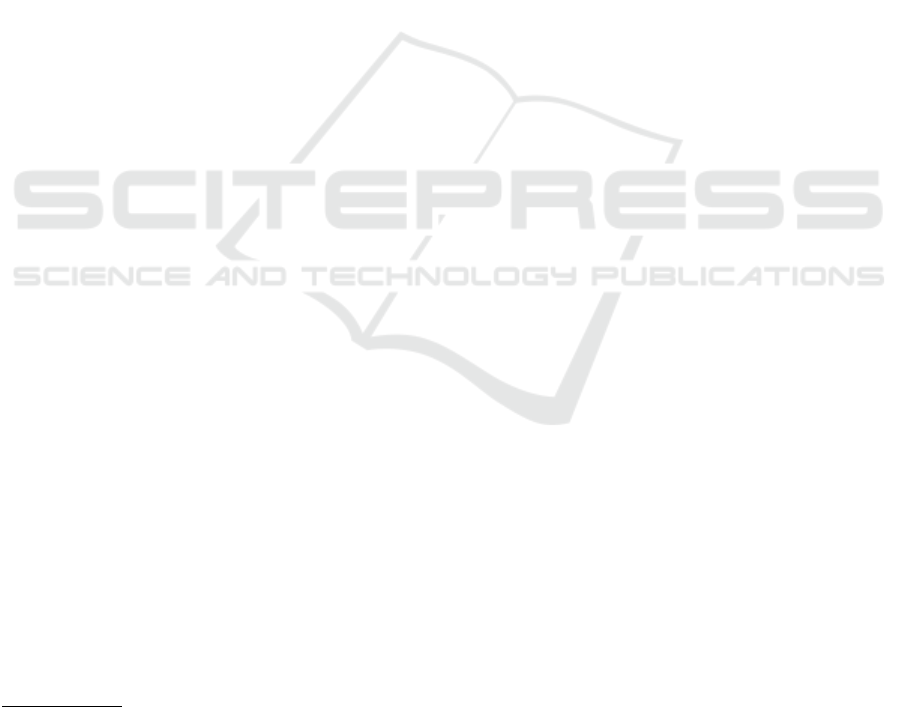
phase.
We use RAND U.S. Health and Retirement Study
(HRS) data
4
. The used dataset comprises health sta-
tus and risk factor details from 42,406 survey partic-
ipants born between the years 1890 and 1995. The
features in the HRS dataset that were used in this re-
search are described in Table 1.
Table 2 presents the performance of our methods
for the data random samples from the test dataset.
The performance metrics considered include True
Positives (TP), True Negatives (TN), False Positives
(FP), False Negatives (FN)
5
, the False Positive Rate
(FPR), the False Negative Rate (FNR), and the over-
all accuracy in percentage. The FPR and FNR pro-
vide insights into the model’s tendency to categorize
negative and positive cases erroneously, which are re-
spectively calculated as: FPR = FP/(FP + T N), and
FNR = FN/(T P + FN). Finally, accuracy quantifies
the percentage of actual findings in the dataset that
match the ground truth.
In Table 2, experiment 10, which has the highest
accuracy at 68.69%, shows a balance between iden-
tifying true positives and true negatives while min-
imizing both false positives and false negatives. In
contrast, Experiment 5 shows the lowest accuracy,
indicating a higher misclassification rate. On aver-
age, these experiments have the accuracy 62.48%, and
across the 10 experiments, the model achieved a TP
rate of 27, a TN rate of 34, with FP and FN averag-
ing at 16 and 21, respectively. The average FPR was
observed at 0.32, with the FNR at 0.44.
In Table 2, a notable pattern across all experiments
is the higher number of TN compared to TP, and FN
compare to FP. This trend shows that the model has
a tendency to classify individuals as ‘not-disabled’.
In particular, the methods has better performances in
correctly identifying individuals who are not disabled
than it is at identifying those who are disabled.
5 CONCLUSION
This preliminary study explores feature weight opti-
mization for disability classification and shows how
learning and network approaches can be integrated
into healthcare frameworks in a potentially fruitful
way. We plain to compare the results of our method
with other prediction methods. Another possible fu-
4
https://hrs.isr.umich.edu.
5
The TP refers to when an individual’s ground truth is
‘not disabled’, but they are incorrectly classified as ‘dis-
abled’, and the FN refers to when an individual’s ground
truth is ‘disabled’, but they are incorrectly classified as ‘not
disabled’.
ture direction is to improve the ability to classify ‘dis-
abled’ individuals. Extending our dataset to include
a wider variety of demographic and geographic char-
acteristics is expected to enhance the generalizability
and relevance of our findings.
REFERENCES
Bondy, J. A. and Murty, U. S. R. (2008). Graph theory.
Springer Publishing Company, Incorporated.
Cui, H., Lu, J., Wang, S., Xu, R., Ma, W., Yu, S.,
Yu, Y., Kan, X., Ling, C., Ho, J., et al. (2023).
A survey on knowledge graphs for healthcare: Re-
sources, applications, and promises. arXiv preprint
arXiv:2306.04802.
Health and Study, R. (2008). Public use dataset. produced
and distributed by the university of michigan with
funding from the national institute on aging (grant
number nia u01ag009740).
Hosseinzadeh, M. M. (2020). Dense subgraphs in biologi-
cal networks. In International conference on current
trends in theory and practice of informatics, pages
711–719. Springer.
Hosseinzadeh, M. M., Cannataro, M., Guzzi, P. H., and
Dondi, R. (2022). Temporal networks in biology and
medicine: a survey on models, algorithms, and tools.
Network Modeling Analysis in Health Informatics and
Bioinformatics, 12(1):10.
Li, Z., Shao, A. W., and Sherris, M. (2017). The impact of
systematic trend and uncertainty on mortality and dis-
ability in a multistate latent factor model for transition
rates. North American Actuarial Journal, 21(4):594–
610.
Pham, T., Tao, X., Zhanag, J., Yong, J., Zhang, W., and
Cai, Y. (2018). Mining heterogeneous information
graph for health status classification. In 2018 5th
International Conference on Behavioral, Economic,
and Socio-Cultural Computing (BESC), pages 73–78.
IEEE.
Pham, T., Tao, X., Zhang, J., Yong, J., Li, Y., and Xie,
H. (2022). Graph-based multi-label disease prediction
model learning from medical data and domain knowl-
edge. Knowledge-based systems, 235:107662.
Rossetti, G. and Cazabet, R. (2018). Community discov-
ery in dynamic networks: a survey. ACM computing
surveys (CSUR), 51(2):1–37.
Stuck, A. E., Walthert, J. M., Nikolaus, T., B
¨
ula, C. J.,
Hohmann, C., and Beck, J. C. (1999). Risk factors for
functional status decline in community-living elderly
people: a systematic literature review. Social science
& medicine, 48(4):445–469.
Tao, X., Pham, T., Zhang, J., Yong, J., Goh, W. P., Zhang,
W., and Cai, Y. (2020). Mining health knowledge
graph for health risk prediction. World Wide Web,
23:2341–2362.
Wang, T., Qiu, R. G., Yu, M., and Zhang, R. (2020). Di-
rected disease networks to facilitate multiple-disease
risk assessment modeling. Decision Support Systems,
129:113171.
KDIR 2024 - 16th International Conference on Knowledge Discovery and Information Retrieval
362