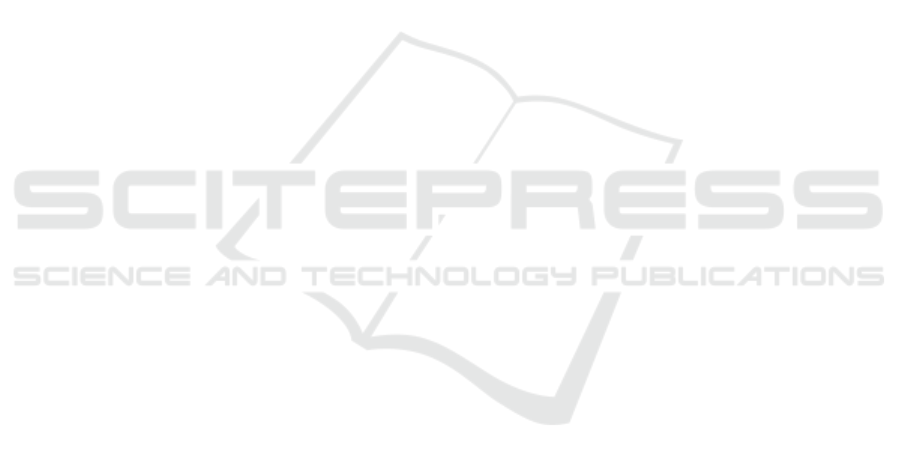
mentations of MH. Second, applications with previ-
ously unsolved challenges can be identified as such
and targeted specifically in future research. Imple-
mentation can focus on core sources of complexity
for tackling future problems.
REFERENCES
Ahmed, U., Carpitella, S., and Certa, A. (2021). An inte-
grated methodological approach for optimising com-
plex systems subjected to predictive maintenance. Re-
liability Engineering & System Safety, 216:108022.
Blancke, O., Combette, A., Amyot, N., and Komljenovic,
D. (2019). A predictive maintenance approach for
complex equipment based on a failure mechanism
propagation model. International Journal of Prognos-
tics and Health Management, 10.
Dai, J., Chen, C. L. P., Xu, X.-Y., and Hu, P. (2008). Condi-
tion monitoring on complex machinery for predictive
maintenance and process control. In 2008 IEEE Inter-
national Conference on Systems, Man and Cybernet-
ics, pages 3595–3600.
Dom
´
ınguez-Monferrer, C., Fern
´
andez-P
´
erez, J., De Santos,
R., Migu
´
elez, M., and Cantero, J. (2022). Machine
learning approach in non-intrusive monitoring of tool
wear evolution in massive cfrp automatic drilling pro-
cesses in the aircraft industry. Journal of Manufactur-
ing Systems, 65:622–639.
Elahi, M., Afolaranmi, S. O., Mohammed, W. M., and Mar-
tinez Lastra, J. L. (2022). Energy-based prognostics
for gradual loss of conveyor belt tension in discrete
manufacturing systems. Energies, 15(13).
Farooq, U., Ademola, M., and Shaalan, A. (2024). Compar-
ative analysis of machine learning models for predic-
tive maintenance of ball bearing systems. Electronics,
13(2).
Fossier, S. and Robic, P.-O. (2017). Maintenance of com-
plex systems — from preventive to predictive. In 2017
12th International Conference on Live Maintenance
(ICOLIM), pages 1–6.
Gashi, M. and Thalmann, S. (2020). Taking complex-
ity into account: A structured literature review on
multi-component systems in the context of predictive
maintenance. In Themistocleous, M. and Papadaki,
M., editors, Information Systems, pages 31–44, Cham.
Springer International Publishing.
Hesser, D. F. and Markert, B. (2019). Tool wear monitor-
ing of a retrofitted cnc milling machine using artificial
neural networks. Manufacturing Letters, 19:1–4.
Izagirre, U., Andonegui, I., Egea, A., and Zurutuza, U.
(2020). A methodology and experimental implemen-
tation for industrial robot health assessment via torque
signature analysis. Applied Sciences, 10(21).
Klein, P. and Bergmann, R. (2019). Generation of complex
data for ai-based predictive maintenance research with
a physical factory model. In ICINCO (1), pages 40–
50. Scitepress.
Lee, D. and Pan, R. (2017). Predictive maintenance
of complex system with multi-level reliability struc-
ture. International Journal of Production Research,
55(16):4785–4801.
Lis, A., Dworakowski, Z., and Czubak, P. (2021). An
anomaly detection method for rotating machinery
monitoring based on the most representative data.
Journal of Vibroengineering.
Mallioris, P., Aivazidou, E., and Bechtsis, D. (2024). Pre-
dictive maintenance in industry 4.0: A systematic
multi-sector mapping. CIRP Journal of Manufactur-
ing Science and Technology, 50:80–103.
Miller, K. and Dubrawski, A. (2020). System-level predic-
tive maintenance: Review of research literature and
gap analysis.
Naeem, M., Jamal, T., Diaz-Martinez, J., Butt, S. A., Mon-
tesano, N., Tariq, M. I., De-la Hoz-Franco, E., and
De-La-Hoz-Valdiris, E. (2022). Trends and future per-
spective challenges in big data. In Pan, J.-S., Balas,
V. E., and Chen, C.-M., editors, Advances in Intelli-
gent Data Analysis and Applications, pages 309–325,
Singapore. Springer Singapore.
Nguyen, K.-A., Do, P., and Grall, A. (2015). Multi-level
predictive maintenance for multi-component systems.
Reliability Engineering & System Safety, 144:83–94.
Nunes, P., Santos, J., and Rocha, E. (2023). Challenges in
predictive maintenance – a review. CIRP Journal of
Manufacturing Science and Technology, 40:53–67.
Roosefert Mohan, T., Preetha Roselyn, J., and Annie Uthra,
R. (2023). Lstm based predictive maintenance ap-
proach for zero breakdown in foundry line through in-
dustry 4.0. In Kumar, H., Jain, P. K., and Goel, S., ed-
itors, Recent Advances in Intelligent Manufacturing,
pages 29–51, Singapore. Springer Nature Singapore.
Serradilla, O., Zugasti, E., Ramirez de Okariz, J., Ro-
driguez, J., and Zurutuza, U. (2021). Adaptable and
explainable predictive maintenance: Semi-supervised
deep learning for anomaly detection and diagnosis in
press machine data. Applied Sciences, 11(16).
Serradilla, O., Zugasti, E., Rodriguez, J., and Zurutuza,
U. (2022). Deep learning models for predictive
maintenance: a survey, comparison, challenges and
prospects. Applied Intelligence, 52.
Van Horenbeek, A. and Pintelon, L. (2013). A dynamic
predictive maintenance policy for complex multi-
component systems. Reliability Engineering & Sys-
tem Safety, 120:39–50.
Wang, Y. and Zhao, Y. (2022). Multi-scale remaining useful
life prediction using long short-term memory. Sustain-
ability, 14(23).
Wu, R. and Keogh, E. J. (2022). Current time series
anomaly detection benchmarks are flawed and are cre-
ating the illusion of progress (extended abstract). In
2022 IEEE 38th International Conference on Data
Engineering (ICDE), pages 1479–1480.
Z
¨
ufle, M., Moog, F., Lesch, V., Krupitzer, C., and Kounev,
S. (2021). A machine learning-based workflow for au-
tomatic detection of anomalies in machine tools. ISA
Transactions, 125.
ICINCO 2024 - 21st International Conference on Informatics in Control, Automation and Robotics
350