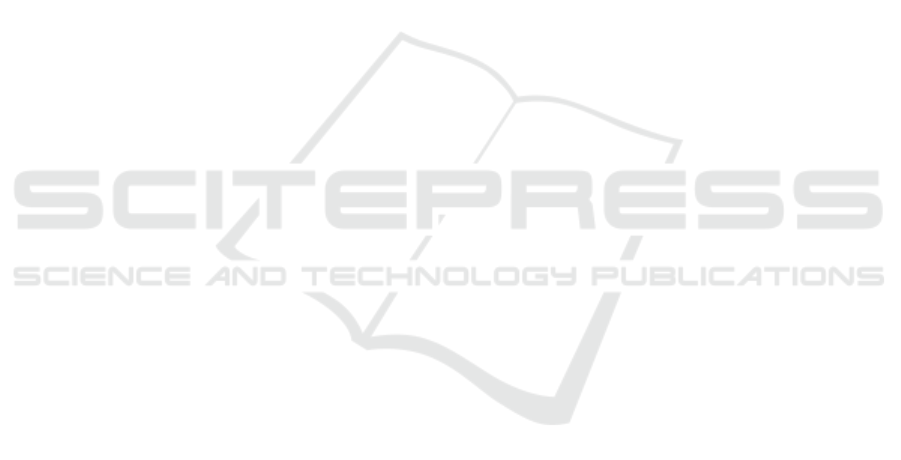
Information Sciences – ISCIS 2006, pages 593–602.
Springer Berlin Heidelberg.
Fernandez-Basso, C., Ruiz, M. D., Delgado, M., and
Martin-Bautista, M. J. (2019). A comparative analysis
of tools for visualizing association rules: A proposal
for visualising fuzzy association rules. In Proceedings
of the 11th Conference of the European Society for
Fuzzy Logic and Technology (EUSFLAT 2019), pages
520–527. Atlantis Press.
Fister, I., Fister, I., Fister, D., Podgorelec, V., Fister,
I., and Salcedo-Sanz, S. (2023). A comprehen-
sive review of visualization methods for association
rule mining: Taxonomy, challenges, open problems
and future ideas. Expert Systems with Applications,
233(June):120901.
Geng, L. and Hamilton, H. J. (2006). Interestingness mea-
sures for data mining: A survey. ACM Comput. Surv.,
38(3):9–es.
Grahne, G. and Zhu, J. (2003). Efficiently using prefix-trees
in mining frequent itemsets. Proc. of the 1st IEEE
ICDM Workshop on Frequent Itemset Mining Imple-
mentations, pages 236–245.
Hahsler, M. (2023). ARULESPY: Exploring Association
Rules and Frequent Itemsets in Python. (Raschka
2018).
Hahsler, M. (2024). A Probabilistic Comparison of Com-
monly Used Interest Measures for Association Rules.
Hahsler, M. and Chelluboina, S. (2011). Visualizing Asso-
ciation Rules: Introduction to the R-extension Pack-
age arulesViz. Technical Report February.
Hahsler, M., Chelluboina, S., and Hornik, D. (2017). Vi-
sualizing association rules: Introduction to the r-
extension package arulesviz. Journal of Statistical
Software, 83(1).
Han, J., Pei, J., and Yin, Y. (2000). Mining frequent patterns
without candidate generation. SIGMOD Record (ACM
Special Interest Group on Management of Data),
29(2):1–12.
Han, J., Pei, J., Yin, Y., and Mao, R. (2004). Min-
ing frequent patterns without candidate generation:
A frequent-pattern tree approach. Data Mining and
Knowledge Discovery, 8(1):53–87.
Henike, T., Kamprath, M., and H
¨
olzle, K. (2020). Effecting,
but effective? How business model visualisations un-
fold cognitive impacts. Long Range Planning, 53(4).
Hofmann, T. and Buhmann, J. M. (2000). Multidimensional
scaling and data clustering. In Advances in Neural
Information Processing Systems, pages 459–466.
Hu, Y. (2006). The Mathematica ® Journal Efficient, High-
Quality Force-Directed Graph Drawing. Methematica
Journal, 10:37–71.
Huang, W., Eades, P., and Hong, S. H. (2009). Measuring
effectiveness of graph visualizations: A cognitive load
perspective. Information Visualization, 8(3):139–152.
Jentner, J., Heitmann, B., and Nagel, W. E. (2019). A sur-
vey on visualization for mining association rules and
frequent item sets. Wiley Interdisciplinary Reviews:
Data Mining and Knowledge Discovery, 9(2).
Jr., R. J. B., Agrawal, R., and Gunopulos, D.
(1999). Constraint-based rule mining in large, dense
databases. In Proceedings of the 15th International
Conference on Data Engineering, pages 188–197.
Klemettinen, M., Mannila, H., Ronkainen, P., Toivonen, H.,
and Verkamo, A. I. (1994). Finding interesting rules
from large sets of discovered association rules. In Pro-
ceedings of the Third International Conference on In-
formation and Knowledge Management, pages 401–
407.
Leung, C. K. S. and Carmichael, C. L. (2009). FpViz: A
visualizer for frequent pattern mining. Proceedings of
the ACM SIGKDD Workshop on Visual Analytics and
Knowledge Discovery, VAKD ’09, (January 2009):30–
39.
Luna, J. M., Ondra, M., Fardoun, H. M., and Ventura,
S. (2018). Optimization of quality measures in as-
sociation rule mining: an empirical study. Interna-
tional Journal of Computational Intelligence Systems,
12:59–78.
Menin, A., Cadorel, L., Tettamanzi, A., Giboin, A., Gan-
don, F., and Winckler, M. (2021). ARViz: Interactive
Visualization of Association Rules for RDF Data Ex-
ploration. In Proceedings of the International Confer-
ence on Information Visualisation, volume 2021-July,
pages 13–20. IEEE.
Ong, K.-h., Ong, K.-l., Ng, W.-k., and Lim, E.-p. (2002).
CrystalClear: Active Visualization of Association
Rules. ICDM’02 International Workshop on Active
Mining AM2002, (February):1–6.
Rainsford, C. P. and Roddick, J. F. (2000). Visualisa-
tion of temporal interval association rules. In Lecture
Notes in Computer Science (including subseries Lec-
ture Notes in Artificial Intelligence and Lecture Notes
in Bioinformatics), volume 1983, pages 91–96.
Shabtay, L., Fournier-Viger, P., Yaari, R., and Dattner,
I. (2021). A guided fp-growth algorithm for min-
ing multitude-targeted item-sets and class associa-
tion rules in imbalanced data. Information Sciences,
553:353–375.
Shahbazi, N. and Gryz, J. (2022). Upper bounds for can-
tree and FP-tree. Journal of Intelligent Information
Systems, 58(1):197–222.
Shaukat Dar, K., Zaheer, S., and Nawaz, I. (2015). Associa-
tion rule mining: An application perspective. Interna-
tional Journal of Computer Science and Innovation,
1:29–38.
Varu, R., Christino, L., and Paulovich, F. V. (2022). AR-
Matrix: An Interactive Item-to-Rule Matrix for Asso-
ciation Rules Visual Analytics. Electronics (Switzer-
land), 11(9).
Wu, T., Chen, Y., and Han, J. (2010). Re-examination of
interestingness measures in pattern mining: A unified
framework. Data Mining and Knowledge Discovery,
21(3):371–397.
Yoghourdjian, V., Yang, Y., Dwyer, T., Lawrence, L.,
Wybrow, M., and Marriott, K. (2021). Scalability
of Network Visualisation from a Cognitive Load Per-
spective. IEEE Transactions on Visualization and
Computer Graphics, 27(2):1677–1687.
KDIR 2024 - 16th International Conference on Knowledge Discovery and Information Retrieval
80