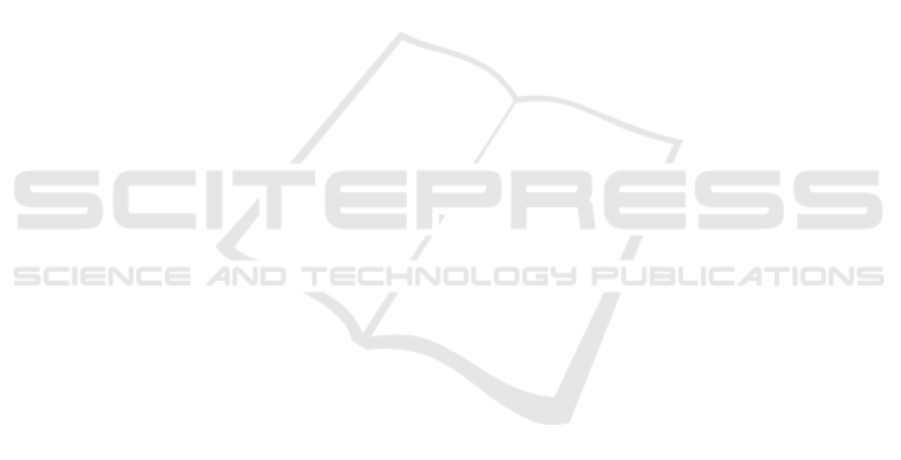
will serve to develop performance forecasting models
integrating data from multiple sensors, including the
combination of heart rate data and performance met-
rics acquired from the camera sensors. By addressing
these areas, the autoWT system can further contribute
to performance optimization in Olympic weightlifting
and serve as an example for long-term performance
research of other complex sports movements.
In conclusion, the autoWT system offers a promis-
ing approach to objective, repeatable, long-term per-
formance tracking. The system has the potential to
transform research and coaching practice, opening
new avenues for future performance optimization in
Olympic weightlifting.
ACKNOWLEDGEMENTS
This research was funded and supported by the EP-
SRC’s DTP, Grant EP/T518128/1 and the industrial
partner - Gymshark. Additional thanks to Gian Singh
Cheema and Warley Weightlifting Club.
REFERENCES
Three-dimensional monitoring of weightlifting for com-
puter assisted training. In VRIC, Laval France.
AN, V. (1978). Weightlifting. International Weightlifting
Federation, Budapest.
Arandjelovi
´
c, O. (2017). Computer-Aided Parameter Selec-
tion for Resistance Exercise Using Machine Vision-
Based Capability Profile Estimation. Augmented Hu-
man Research, 2(1):4.
Bazarevsky, V., Grishchenko, I., Raveendran, K., Zhu, T.,
Zhang, F., and Grundmann, M. (2020). BlazePose:
On-device Real-time Body Pose tracking. Number:
arXiv:2006.10204 arXiv:2006.10204 [cs].
Bolarinwa, D., Qazi, N., and Ghazanfar, M. (2023). Shifting
the Weight: Applications of AI in Olympic Weightlift-
ing. In 2023 IEEE 28th(PRDC), pages 319–326.
ISSN: 2473-3105.
Destro, M. (2024). materight/RepNet-pytorch. original-
date: 2023-02-28T15:28:43Z.
Garhammer, J. and Newton, H. (2013). Applied Video
Analysis for Coaches: Weightlifting Examples. In-
ternational Journal of Sports Science & Coaching,
8(3):581–594.
He, Q., Li, W., Tang, W., and Xu, B. (2023). Recognition to
weightlifting postures using convolutional neural net-
works with evaluation mechanism. Measurement and
Control, page 00202940231215378. Publisher: SAGE
Publications Ltd.
Host, K. and Ivaši
´
c-Kos, M. (2022). An overview of Hu-
man Action Recognition in sports based on Computer
Vision. Heliyon, 8(6):e09633.
Hsu, C.-T., Ho, W.-H., and Chen, J.-S. (2019). High Effi-
cient Weightlifting Barbell Tracking Algorithm Based
on Diamond Search Strategy. In Arkusz, K., B˛edz-
i
´
nski, R., Klekiel, T., and Piszczatowski, S., editors,
Biomechanics in Medicine and Biology, pages 252–
262, Cham. Springer International Publishing.
Hsu, C.-T., Ho, W.-H., Tsai, W.-I., and Lin, Y.-C. (2018).
Realtime weightlifting barbell trajectory extraction
and performance analysis. | Physical Education Jour-
nal | EBSCOhost. ISSN: 1024-7297 Issue: 1 Pages:
73 Volume: 51.
Jian, M., Zhang, S., Wu, L., Zhang, S., Wang, X., and He, Y.
(2019). Deep key frame extraction for sport training.
Neurocomputing, 328:147–156.
Lin, C.-Y. and Jian, K.-C. (2022). A real-time algorithm for
weight training detection and correction. Soft Com-
puting, 26(10):4727–4739.
Mroz, S., Baddour, N., McGuirk, C., Juneau, P., Tu, A.,
Cheung, K., and Lemaire, E. (2021). Comparing the
Quality of Human Pose Estimation with BlazePose
or OpenPose. In 2021 4th International Conference
on Bio-Engineering for Smart Technologies (BioS-
MART), pages 1–4.
Needham, L., Evans, M., Cosker, D. P., Wade, L.,
McGuigan, P. M., Bilzon, J. L., and Colyer, S. L.
(2021). The accuracy of several pose estimation meth-
ods for 3D joint centre localisation. Scientific Reports,
11(1):20673. Number: 1 Publisher: Nature Publish-
ing Group.
Pan, S. (2022). A Method of Key Posture Detection and
Motion Recognition in Sports Based on Deep Learn-
ing. Mobile Information Systems, 2022:e5168898.
Publisher: Hindawi.
Rethinam, P., Manoharan, S., Kirupakaran, A. M.,
Srinivasan, R., Hegde, R. S., and Srinivasan, B.
(2023). Olympic Weightlifters’ Performance Assess-
ment Module Using Computer Vision. In 2023 IEEE
International Workshop on Sport, Technology and Re-
search (STAR), pages 8–12.
Yoshikawa, F., Kobayashi, T., Watanabe, K., Katsuyoshi,
S., and Otsu, N. (2010). Start and End Point Detection
of Weightlifting Motion using CHLAC and MRA:.
In B-Interface 2011, pages 44–50, Valencia, Spain.
SciTePress.
autoWT: A Semi-Automated ML-Based Movement Tracking System for Performance Tracking and Analysis in Olympic Weightlifting
71