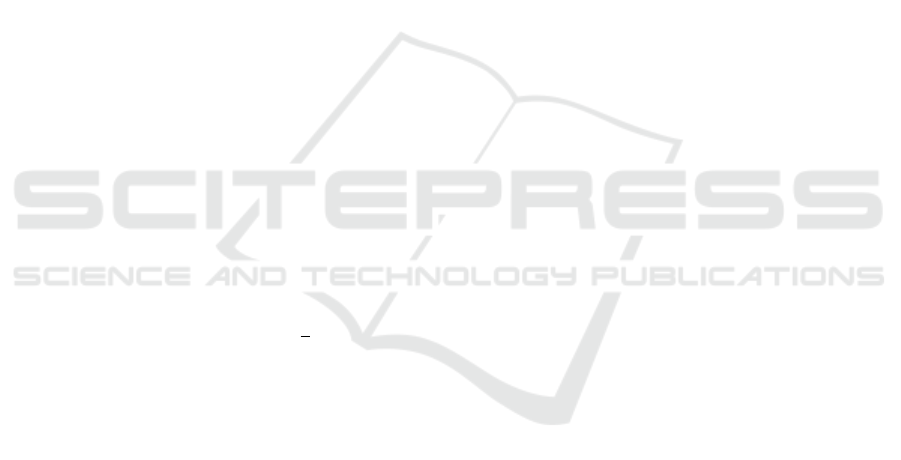
REFERENCES
Abraham, I., Torre, G. D. L., and Murphey, T. D.
(2017). Model-based control using koopman opera-
tors. CoRR, abs/1709.01568.
Bongiovanni, N., Mavkov, B., Martins, R., and Allibert,
G. (2024). Data-Driven Nonlinear System Identifica-
tion of a Throttle Valve Using Koopman Representa-
tion. In American Control Conference (ACC 2024),
Toronto, Canada.
Brunton, S. L., Budi
ˇ
si
´
c, M., Kaiser, E., and Kutz, J. N.
(2021). Modern koopman theory for dynamical sys-
tems.
Brunton, S. L. and Kutz, J. N. (2019). Data-Driven Science
and Engineering: Machine Learning, Dynamical Sys-
tems, and Control. Cambridge University Press, USA,
1st edition.
Buzhardt, J. and Tallapragada, P. (2022). A koopman op-
erator approach for the vertical stabilization of an off-
road vehicle. IFAC-PapersOnLine, 55(37):675–680.
2nd Modeling, Estimation and Control Conference
MECC 2022.
Chen, H., He, X., Cheng, S., and Lv, C. (2024a). Deep
koopman operator-informed safety command gover-
nor for autonomous vehicles. IEEE/ASME Transac-
tions on Mechatronics, pages 1–11.
Chen, Z., Chen, X., Liu, J., Cen, L., and Gui, W. (2024b).
Learning model predictive control of nonlinear sys-
tems with time-varying parameters using koopman
operator. Applied Mathematics and Computation,
470:128577.
Cibulka, V., Hanis, T., and Hromcik, M. (2019). Data-
driven identification of vehicle dynamics using koop-
man operator. 2019 22nd International Conference on
Process Control (PC19), pages 167–172.
Gavish, M. and Donoho, D. L. (2014). The optimal hard
threshold for singular values is 4/
√
3. IEEE Transac-
tions on Information Theory, 60(8):5040–5053.
Guo, W., Zhao, S., Cao, H., Yi, B., and Song, X.
(2023). Koopman operator-based driver-vehicle dy-
namic model for shared control systems. Applied
Mathematical Modelling, 114:423–446.
Gupta, S., Shen, D., Karbowski, D., and Rousseau, A.
(2022). Koopman model predictive control for eco-
driving of automated vehicles. In 2022 American Con-
trol Conference (ACC), pages 2443–2448.
Han, Y., Hao, W., and Vaidya, U. (2020). Deep learning
of koopman representation for control. In 2020 59th
IEEE Conference on Decision and Control (CDC),
pages 1890–1895.
Joglekar, A., Samak, C., Samak, T., Kosaraju, K. C.,
Smereka, J., Brudnak, M., Gorsich, D., Krovi, V., and
Vaidya, U. (2023). Analytical construction of koop-
man edmd candidate functions for optimal control
of ackermann-steered vehicles. IFAC-PapersOnLine,
56(3):619–624. 3rd Modeling, Estimation and Con-
trol Conference MECC 2023.
Kim, J. S., Quan, Y. S., and Chung, C. C. (2022). Data-
driven modeling and control for lane keeping sys-
tem of automated driving vehicles: Koopman opera-
tor approach. In 2022 22nd International Conference
on Control, Automation and Systems (ICCAS), pages
1049–1055.
Kim, J. S., Quan, Y. S., and Chung, C. C. (2023). Koop-
man operator-based model identification and control
for automated driving vehicle. International Jour-
nal of Control, Automation and Systems 21, page
2431–2443.
Koopman, B. O. (1931). Hamiltonian systems and transfor-
mation in hilbert space. Proceedings of the National
Academy of Sciences, 17(5):315–318.
Manzoor, W. A., Rawashdeh, S., and Mohammadi, A.
(2023). Vehicular applications of koopman operator
theory—a survey. IEEE Access, 11:25917–25931.
Mauroy, A. and Goncalves, J. (2020). Koopman-based
lifting techniques for nonlinear systems identifica-
tion. IEEE Transactions on Automatic Control,
65(6):2550–2565.
Schreier, M. (2017). Bayesian environment representa-
tion, prediction, and criticality assessment for driver
assistance systems. at - Automatisierungstechnik,
65(2):151–152.
Wilson, D. (2023). Koopman operator inspired nonlinear
system identification. SIAM Journal on Applied Dy-
namical Systems, 22(2):1445–1471.
Xiao, Y., Zhang, X., Xu, X., Liu, X., and Liu, J. (2023).
Deep neural networks with koopman operators for
modeling and control of autonomous vehicles. IEEE
Transactions on Intelligent Vehicles, 8(1):135–146.
Yu, S., Shen, C., and Ersal, T. (2022a). Autonomous driv-
ing using linear model predictive control with a koop-
man operator based bilinear vehicle model. IFAC-
PapersOnLine, 55(24):254–259. 10th IFAC Sympo-
sium on Advances in Automotive Control AAC 2022.
Yu, S., Sheng, E., Zhang, Y., Li, Y., Chen, H., and Hao, Y.
(2022b). Efficient nonlinear model predictive control
of automated vehicles. Mathematics, 10(21).
Analysis of Truncated Singular Value Decomposition for Koopman Operator-Based Lane Change Model
683