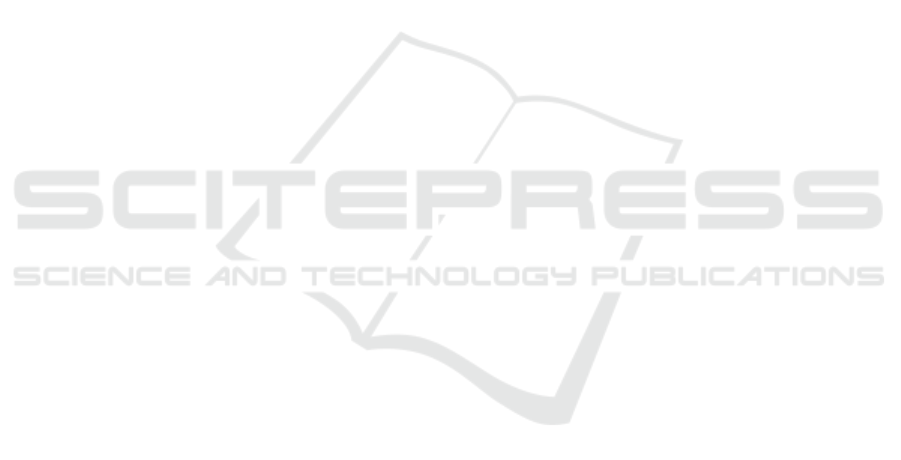
Buchfink, B., Xie, C., and Huson, D. H. (2015). Fast and
sensitive protein alignment using diamond. Nature
methods, 12(1):59–60.
Chowdhury, A. S., Call, D. R., and Broschat, S. L. (2019).
Antimicrobial resistance prediction for gram-negative
bacteria via game theory-based feature evaluation.
Scientific reports, 9(1):14487.
Consortium, U. (2015). Uniprot: a hub for protein informa-
tion. Nucleic acids research, 43(D1):D204–D212.
Davis, J. J., Boisvert, S., Brettin, T., Kenyon, R. W., Mao,
C., Olson, R., Overbeek, R., Santerre, J., Shukla, M.,
Wattam, A. R., et al. (2016). Antimicrobial resis-
tance prediction in patric and rast. Scientific reports,
6(1):27930.
Devlin, J., Chang, M.-W., Lee, K., and Toutanova, K.
(2018). Bert: Pre-training of deep bidirectional trans-
formers for language understanding.
Dietterich, T. G. (2000). Ensemble methods in machine
learning. In International workshop on multiple clas-
sifier systems, pages 1–15. Springer.
Elnaggar, A., Heinzinger, M., Dallago, C., Rihawi, G.,
Wang, Y., Jones, L., Gibbs, T., Feher, T., Angerer, C.,
Steinegger, M., Bhowmik, D., and Rost, B. (2021).
Prottrans: Towards cracking the language of life’s
code through self-supervised deep learning and high
performance computing.
Feldgarden, M., Brover, V., Haft, D. H., Prasad, A. B.,
Slotta, D. J., Tolstoy, I., Tyson, G. H., Zhao, S., Hsu,
C.-H., McDermott, P. F., et al. (2019). Validating the
amrfinder tool and resistance gene database by using
antimicrobial resistance genotype-phenotype correla-
tions in a collection of isolates. Antimicrobial agents
and chemotherapy, 63(11):10–1128.
Gibson, M. K., Forsberg, K. J., and Dantas, G. (2015).
Improved annotation of antibiotic resistance determi-
nants reveals microbial resistomes cluster by ecology.
The ISME journal, 9(1):207–216.
Jia, B., Raphenya, A. R., Alcock, B., Waglechner, N., Guo,
P., Tsang, K. K., Lago, B. A., Dave, B. M., Pereira,
S., Sharma, A. N., et al. (2016). Card 2017: expan-
sion and model-centric curation of the comprehensive
antibiotic resistance database. Nucleic acids research,
page gkw1004.
Kleinheinz, K. A., Joensen, K. G., and Larsen, M. V.
(2014). Applying the resfinder and virulencefinder
web-services for easy identification of acquired antibi-
otic resistance and e. coli virulence genes in bacterio-
phage and prophage nucleotide sequences. Bacterio-
phage, 4(2):e27943.
Lakin, S. M., Dean, C., Noyes, N. R., Dettenwanger, A.,
Ross, A. S., Doster, E., Rovira, P., Abdo, Z., Jones,
K. L., Ruiz, J., et al. (2017). Megares: an antimicro-
bial resistance database for high throughput sequenc-
ing. Nucleic acids research, 45(D1):D574–D580.
L
´
az
´
ar, V. and Kishony, R. (2019). Transient antibiotic
resistance calls for attention. Nature microbiology,
4(10):1606–1607.
Li, H. and Durbin, R. (2009). Fast and accurate short read
alignment with burrows–wheeler transform. bioinfor-
matics, 25(14):1754–1760.
Li, Y., Wang, S., Umarov, R., Xie, B., Fan, M., Li, L., and
Gao, X. (2018). Deepre: sequence-based enzyme ec
number prediction by deep learning. Bioinformatics,
34(5):760–769.
Li, Y., Xu, Z., Han, W., Cao, H., Umarov, R., Yan, A.,
Fan, M., Chen, H., Duarte, C. M., Li, L., et al. (2021).
Hmd-arg: hierarchical multi-task deep learning for an-
notating antibiotic resistance genes. Microbiome, 9:1–
12.
Mao, D., Yu, S., Rysz, M., Luo, Y., Yang, F., Li, F., Hou,
J., Mu, Q., and Alvarez, P. (2015). Prevalence and
proliferation of antibiotic resistance genes in two mu-
nicipal wastewater treatment plants. Water research,
85:458–466.
McArthur, A. G. and Tsang, K. K. (2017). Antimicrobial
resistance surveillance in the genomic age. Annals of
the New York Academy of Sciences, 1388(1):78–91.
McArthur, A. G., Waglechner, N., Nizam, F., Yan, A., Azad,
M. A., Baylay, A. J., Bhullar, K., Canova, M. J.,
De Pascale, G., Ejim, L., et al. (2013). The compre-
hensive antibiotic resistance database. Antimicrobial
agents and chemotherapy, 57(7):3348–3357.
Mendelson M, M. M. (2015). The world health organization
global action plan for antimicrobial resistance. S Afr
Med J, 105(5):325.
Miah, M. S. U., Kabir, M. M., Sarwar, T. B., Safran, M.,
Alfarhood, S., and Mridha, M. (2024). A multimodal
approach to cross-lingual sentiment analysis with en-
semble of transformer and llm. Scientific Reports,
14(1):9603.
Murray, C. J., Ikuta, K. S., Sharara, F., Swetschinski, L.,
Aguilar, G. R., Gray, A., Han, C., Bisignano, C., Rao,
P., Wool, E., et al. (2022). Global burden of bacterial
antimicrobial resistance in 2019: a systematic analy-
sis. The lancet, 399(10325):629–655.
Pehrsson, E. C., Tsukayama, P., Patel, S., Mej
´
ıa-Bautista,
M., Sosa-Soto, G., Navarrete, K. M., Calderon,
M., Cabrera, L., Hoyos-Arango, W., Bertoli, M. T.,
et al. (2016). Interconnected microbiomes and re-
sistomes in low-income human habitats. Nature,
533(7602):212–216.
Pham, V. H. and Kim, J. (2012). Cultivation of unculturable
soil bacteria. Trends in biotechnology, 30(9):475–484.
Rao, R. M., Liu, J., Verkuil, R., Meier, J., Canny, J. F.,
Abbeel, P., Sercu, T., and Rives, A. (2021). Trans-
former protein language models are unsupervised
structure learners. bioRxiv.
Rupp
´
e, E., Ghozlane, A., Tap, J., Pons, N., Alvarez, A.-S.,
Maziers, N., Cuesta, T., Hernando-Amado, S., Clares,
I., Mart
´
ınez, J. L., et al. (2019). Prediction of the
intestinal resistome by a three-dimensional structure-
based method. Nature microbiology, 4(1):112–123.
Steinegger, M. and S
¨
oding, J. (2018). Clustering huge pro-
tein sequence sets in linear time. Nature communica-
tions, 9(1):2542.
Suzek, B. E., Wang, Y., Huang, H., McGarvey, P. B.,
Wu, C. H., and Consortium, U. (2015). Uniref clus-
ters: a comprehensive and scalable alternative for im-
proving sequence similarity searches. Bioinformatics,
31(6):926–932.
Antibiotic Resistance Gene Identification from Metagenomic Data Using Ensemble of Finetuned Large Language Models
111