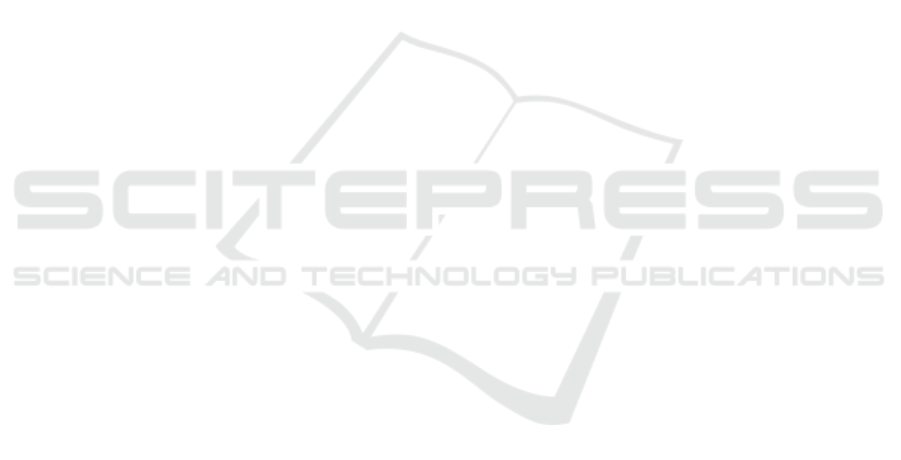
REFERENCES
Cai, X., Ning, H., Dhelim, S., Zhou, R., Zhang, T., Xu, Y.,
and Wan, Y. (2021). Robot and its living space: A
roadmap for robot development based on the view of
living space. Digital Communications and Networks,
7(4):505–517.
Camarinha-Matos, L. M. and Afsarmanesh, H. (2018).
Roots of collaboration: nature-inspired solutions for
collaborative networks. IEEE Access, 6:30829–
30843.
Camarinha-Matos, L. M. and Afsarmanesh, H. (2021). The
evolution path to collaborative networks 4.0. Ad-
vancing Research in Information and Communication
Technology: IFIP’s Exciting First 60+ Years, Views
from the Technical Committees and Working Groups,
pages 170–193.
Cresswell, K., Cunningham-Burley, S., and Sheikh, A.
(2018). Health care robotics: qualitative exploration
of key challenges and future directions. Journal of
medical Internet research, 20(7):e10410.
Fragapane, G., Ivanov, D., Peron, M., Sgarbossa, F., and
Strandhagen, J. O. (2022). Increasing flexibility and
productivity in industry 4.0 production networks with
autonomous mobile robots and smart intralogistics.
Annals of operations research, 308(1):125–143.
Guerrini, F. M. and Yamanari, J. S. (2019). A systematic re-
view of collaborative networks: implications for sens-
ing, smart and sustainable enterprises. In Collabora-
tive Networks and Digital Transformation: 20th IFIP
WG 5.5 Working Conference on Virtual Enterprises,
PRO-VE 2019, Turin, Italy, September 23–25, 2019,
Proceedings 20, pages 69–80. Springer.
Hooman, S. (2023). Creative Robotics. Colour Edition.
Hor
´
akov
´
a, J. and Kelemen, J. (2008). The robot story: why
robots were born and how they grew up.
Javaid, M., Haleem, A., Singh, R. P., and Suman, R. (2021).
Substantial capabilities of robotics in enhancing i4.0
implementation. Cognitive Robotics, 1:58–75.
Kalaitzidou, M. and Pachidis, T. P. (2023). Recent robots in
steam education. Education Sciences, 13(3):272.
Kappas, A. and Gratch, J. (2023). These aren’t the droids
you are looking for: Promises and challenges for the
intersection of affective science and robotics/ai. Affec-
tive Science, 4(3):580–585.
Kattel, R., Lember, V., and T
˜
onurist, P. (2020). Collabora-
tive innovation and human-machine networks. Public
management review, 22(11):1652–1673.
Kluger, L. C., Gorris, P., Kochalski, S., Mueller, M. S., and
Romagnoni, G. (2020). Studying human–nature re-
lationships through a network lens: A systematic re-
view. People and Nature, 2(4):1100–1116.
Leigh, N. G., Kraft, B., and Lee, H. (2020). Robots, skill
demand and manufacturing in us regional labour mar-
kets. Cambridge Journal of Regions, Economy and
Society, 13(1):77–97.
Liu, J., Wang, Z., Qian, L., Luo, R., and Luo, X. (2023).
Task-oriented systematic design of a heavy-duty elec-
trically actuated quadruped robot with high perfor-
mance. Sensors, 23(15):6696.
Marchetti, E., Nikghadam-Hojjati, S., and Barata, J. (2023).
Collaborative network 5.0: By design human values
and human-centred based extended collaborative net-
works. In Working Conference on Virtual Enterprises,
pages 415–430. Springer.
Maroto-G
´
omez, M., Castro-Gonz
´
alez,
´
A., Malfaz, M.,
and Salichs, M.
´
A. (2023). A biologically inspired
decision-making system for the autonomous adaptive
behavior of social robots. Complex & Intelligent Sys-
tems, 9(6):6661–6679.
Mor, R. S., Kumar, D., Singh, A., and Neethu, K. (2022).
Robotics and automation for agri-food 4.0: Innovation
and challenges. In Agri-food 4.0: Innovations, chal-
lenges and strategies, pages 189–199. Emerald Pub-
lishing Limited.
Narayan, S., Aquif, M., Kalim, A. R., Chagarlamudi, D.,
and Harshith Vignesh, M. (2022). Search and re-
connaissance robot for disaster management. In Ma-
chines, Mechanism and Robotics: Proceedings of iN-
aCoMM 2019, pages 187–201. Springer.
Nikghadam-Hojjati, S. and Barata, J. (2019). Computa-
tional creativity to design cyber-physical systems in
industry 4.0. In Collaborative Networks and Digi-
tal Transformation: 20th IFIP WG 5.5 Working Con-
ference on Virtual Enterprises, PRO-VE 2019, Turin,
Italy, September 23–25, 2019, Proceedings 20, pages
29–40. Springer.
N
´
obrega, L., Marchhausen, L., Martinez, L. F., Lima, Y.,
Almeida, M., Lyra, A., Barbosa, C. E., and Moreira de
Souza, J. (2023). Ai delphi: Machine-machine collab-
oration for exploring the future of work. Available at
SSRN 4660589.
Pawlicka, A., Pawlicki, M., Kozik, R., and Chora
´
s, M.
(2022). Human-driven and human-centred cybersecu-
rity: policy-making implications. Transforming Gov-
ernment: People, Process and Policy, 16(4):478–487.
Santos, N. B., Bavaresco, R. S., Tavares, J. E., Ramos, G.
d. O., and Barbosa, J. L. (2021). A systematic map-
ping study of robotics in human care. Robotics and
Autonomous Systems, 144:103833.
Thuruthel, T. G., Shih, B., Laschi, C., and Tolley, M. T.
(2019). Soft robot perception using embedded soft
sensors and recurrent neural networks. Science
Robotics, 4(26):eaav1488.
Ventura, D. (2019). Autonomous intentionality in compu-
tationally creative systems. Computational creativity:
The philosophy and engineering of autonomously cre-
ative systems, pages 49–69.
Wong, C., Yang, E., Yan, X.-T., and Gu, D. (2018). Au-
tonomous robots for harsh environments: a holistic
overview of current solutions and ongoing challenges.
Systems Science & Control Engineering, 6(1):213–
219.
Yao, B. (2021). International research collaboration: chal-
lenges and opportunities. Journal of Diagnostic Med-
ical Sonography, 37(2):107–108.
Zhuge, H. (2020). Cyber-physical-social intelligence.
Cyber-Physical-Social Intelligence.
WEBIST 2024 - 20th International Conference on Web Information Systems and Technologies
314