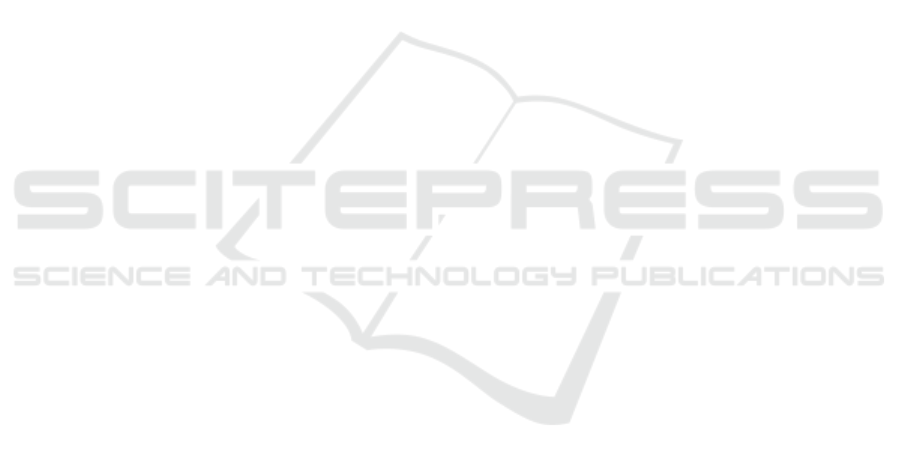
tors, Progress in Pattern Recognition, Image Analysis,
Computer Vision, and Applications, pages 584–593,
Cham. Springer International Publishing.
Alvi, M., Zisserman, A., and Nell
˚
aker, C. (2019). Turning
a blind eye: Explicit removal of biases and variation
from deep neural network embeddings. In Leal-Taix
´
e,
L. and Roth, S., editors, Computer Vision – ECCV
2018 Workshops, pages 556–572, Cham. Springer In-
ternational Publishing.
Angwin, J., Larson, J., Mattu, S., and Kirchner,
L. (2016). Machine bias: There’s software
used across the country to predict future crim-
inals. and it’s biased against blacks. ProP-
ublica. https://www.propublica.org/article/
machine-bias-risk-assessments-in-criminal-sentencing.
Anonymous (2024). Towards Fairness In Machine Learning
- experiments, code and data. https://doi.org/10.5281/
zenodo.12672289.
Balakrishnan, G., Xiong, Y., Xia, W., and Perona, P. (2020).
Towards causal benchmarking of bias in face analy-
sis algorithms. In Vedaldi, A., Bischof, H., Brox, T.,
and Frahm, J.-M., editors, Computer Vision – ECCV
2020, pages 547–563, Cham. Springer International
Publishing.
Benthall, S. and Haynes, B. D. (2019). Racial categories in
machine learning. In Proceedings of the Conference
on Fairness, Accountability, and Transparency, FAT*
’19, page 289–298, New York, NY, USA. Association
for Computing Machinery.
Bogen, M. and Rieke, A. (2018). Help wanted: an exami-
nation of hiring algorithms, equity, and bias.
Cao, Q., Shen, L., Xie, W., Parkhi, O. M., and Zisserman,
A. (2018). Vggface2: A dataset for recognising faces
across pose and age.
Danks, D. and London, A. (2017). Algorithmic bias in au-
tonomous systems. pages 4691–4697.
Goutte, C. and Gaussier, E. (2005). A probabilistic inter-
pretation of precision, recall and f-score, with impli-
cation for evaluation. In Losada, D. E. and Fern
´
andez-
Luna, J. M., editors, Advances in Information Re-
trieval, pages 345–359. Springer Berlin Heidelberg.
Hardt, M., Price, E., Price, E., and Srebro, N. (2016). Equal-
ity of opportunity in supervised learning.
Howard, A. and Borenstein, J. (2018). The ugly truth about
ourselves and our robot creations: The problem of bias
and social inequity. Science and Engineering Ethics,
24.
Islam, A. U. (2023). Gender and Ethnicity Bias in Deep
Learning. PhD thesis.
K
¨
arkk
¨
ainen, K. and Joo, J. (2019). Fairface: Face attribute
dataset for balanced race, gender, and age. ArXiv,
abs/1908.04913.
Karras, T., Laine, S., and Aila, T. (2019). A style-based
generator architecture for generative adversarial net-
works.
Maluleke, V. H., Thakkar, N., Brooks, T., Weber, E., Dar-
rell, T., Efros, A. A., Kanazawa, A., and Guillory, D.
(2022). Studying bias in gans through the lens of race.
Mehrabi, N., Morstatter, F., Saxena, N., Lerman, K., and
Galstyan, A. (2022). A survey on bias and fairness in
machine learning.
Nitzan, Y., Bermano, A., Li, Y., and Cohen-Or, D. (2020).
Face identity disentanglement via latent space map-
ping. ACM Transactions on Graphics (TOG), 39:1 –
14.
Raji, I. D., Smart, A., White, R. N., Mitchell, M., Gebru,
T., Hutchinson, B., Smith-Loud, J., Theron, D., and
Barnes, P. (2020). Closing the ai accountability gap:
Defining an end-to-end framework for internal algo-
rithmic auditing.
Schroff, F., Kalenichenko, D., and Philbin, J. (2015).
Facenet: A unified embedding for face recognition
and clustering. In 2015 IEEE Conference on Com-
puter Vision and Pattern Recognition (CVPR). IEEE.
Serengil, S. I. and Ozpinar, A. (2021). Hyperextended light-
face: A facial attribute analysis framework. In 2021
International Conference on Engineering and Emerg-
ing Technologies (ICEET), pages 1–4. IEEE.
Srinivasan, R. and Chander, A. (2021). Biases in ai systems.
Commun. ACM, 64(8):44–49.
W Flores, A., Bechtel, K., and Lowenkamp, C. (2016).
False positives, false negatives, and false analyses:
A rejoinder to “machine bias: There’s software used
across the country to predict future criminals. and it’s
biased against blacks.”. Federal probation, 80.
Wang, T., Zhao, J., Yatskar, M., Chang, K.-W., and Or-
donez, V. (2019). Balanced datasets are not enough:
Estimating and mitigating gender bias in deep image
representations.
Zhang, Z., Song, Y., and Qi, H. (2017). Age progression
regression by conditional adversarial autoencoder.
NCTA 2024 - 16th International Conference on Neural Computation Theory and Applications
592