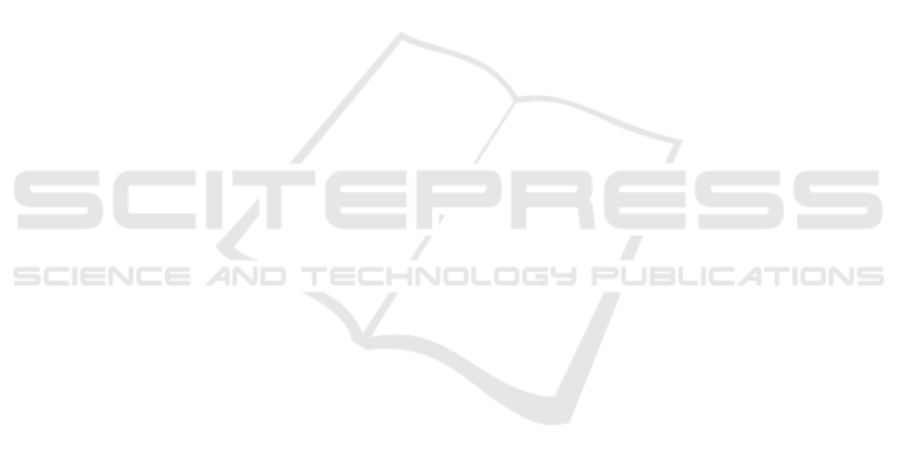
Heuillet, A., Couthouis, F., and D
´
ıaz-Rodr
´
ıguez, N.
(2021). Explainability in deep reinforcement learning.
Knowledge-Based Systems, 214:106685.
Huang, S. H., Bhatia, K., Abbeel, P., and Dragan, A. D.
(2018). Establishing appropriate trust via critical
states. CoRR, abs/1810.08174.
Huang, S. H., Held, D., Abbeel, P., and Dragan, A. D.
(2017). Enabling robots to communicate their objec-
tives. CoRR, abs/1702.03465.
Ishibuchi, H., Tsukamoto, N., and Nojima, Y. (2008). Evo-
lutionary many-objective optimization: A short re-
view. In 2008 IEEE congress on evolutionary com-
putation (IEEE world congress on computational in-
telligence), pages 2419–2426. IEEE.
Khadka, S. and Tumer, K. (2018). Evolutionary reinforce-
ment learning. CoRR, abs/1805.07917.
Koh, P. W. and Liang, P. (2017). Understanding black-box
predictions via influence functions. In International
conference on machine learning, pages 1885–1894.
PMLR.
Lage, I., Lifschitz, D., Doshi-Velez, F., and Amir, O.
(2019). Exploring computational user models for
agent policy summarization. CoRR, abs/1905.13271.
Lehman, J. and Stanley, K. O. (2011). Abandoning objec-
tives: Evolution through the search for novelty alone.
Evolutionary computation, 19(2):189–223.
Li, X., Xiong, H., Li, X., Wu, X., Zhang, X., Liu, J.,
Bian, J., and Dou, D. (2022). Interpretable deep learn-
ing: interpretation, interpretability, trustworthiness,
and beyond. Knowledge and Information Systems.
Lin, B. and Su, J. (2008). One way distance: For shape
based similarity search of moving object trajectories.
GeoInformatica, 12:117–142.
Lundberg, S. M. and Lee, S.-I. (2017). A unified approach
to interpreting model predictions. Advances in neural
information processing systems, 30.
Miller, B. L., Goldberg, D. E., et al. (1995). Genetic algo-
rithms, tournament selection, and the effects of noise.
Complex systems, 9(3):193–212.
Molnar, C. (2020). Interpretable machine learning.
Lulu.com.
Neumann, A., Gao, W., Wagner, M., and Neumann, F.
(2019). Evolutionary diversity optimization using
multi-objective indicators. In Proceedings of the
Genetic and Evolutionary Computation Conference,
pages 837–845.
Pang, Q., Yuan, Y., and Wang, S. (2022). Mdpfuzz: test-
ing models solving markov decision processes. In
Proceedings of the 31st ACM SIGSOFT International
Symposium on Software Testing and Analysis, pages
378–390.
Parker-Holder, J., Jiang, M., Dennis, M., Samvelyan, M.,
Foerster, J., Grefenstette, E., and Rockt
¨
aschel, T.
(2022). Evolving curricula with regret-based environ-
ment design. In International Conference on Machine
Learning, pages 17473–17498. PMLR.
Parker-Holder, J., Pacchiano, A., Choromanski, K., and
Roberts, S. (2020). Effective diversity in population-
based reinforcement learning. CoRR, abs/2002.00632.
Pleiss, G., Zhang, T., Elenberg, E., and Weinberger, K. Q.
(2020). Identifying mislabeled data using the area un-
der the margin ranking. Advances in Neural Informa-
tion Processing Systems, 33:17044–17056.
Puterman, M. L. (1990). Markov decision processes. Hand-
books in operations research and management sci-
ence, 2:331–434.
Raffin, A., Hill, A., Gleave, A., Kanervisto, A., Ernestus,
M., and Dormann, N. (2021). Stable-baselines3: Reli-
able reinforcement learning implementations. Journal
of Machine Learning Research, 22(268):1–8.
Ribeiro, M. T., Singh, S., and Guestrin, C. (2016). ” why
should i trust you?” explaining the predictions of any
classifier. In Proceedings of the 22nd ACM SIGKDD
international conference on knowledge discovery and
data mining, pages 1135–1144.
Richard S. Sutton, A. G. B. (2014, 2015). Reinforcement
Learning: An Introduction. The MIT Press, Cam-
bridge, Massachusetts, London, England, 2 edition.
Rolf, B., Jackson, I., M
¨
uller, M., Lang, S., Reggelin, T., and
Ivanov, D. (2023). A review on reinforcement learning
algorithms and applications in supply chain manage-
ment. International Journal of Production Research,
61(20):7151–7179.
Schulman, J., Wolski, F., Dhariwal, P., Radford, A., and
Klimov, O. (2017). Proximal policy optimization al-
gorithms.
Sequeira, P. and Gervasio, M. (2020). Interestingness ele-
ments for explainable reinforcement learning: Under-
standing agents’ capabilities and limitations. Artificial
Intelligence, 288:103367.
Shapley, L. S. and Shubik, M. (1954). A method for evalu-
ating the distribution of power in a committee system.
American political science review, 48(3):787–792.
Tappler, M., C
´
ordoba, F. C., Aichernig, B. K., and
K
¨
onighofer, B. (2022). Search-based testing of rein-
forcement learning. arXiv preprint arXiv:2205.04887.
Vartiainen, P. (2002). On the principles of comparative eval-
uation. Evaluation, 8(3):359–371.
Wineberg, M. and Oppacher, F. (2003). The underlying
similarity of diversity measures used in evolutionary
computation. In Genetic and evolutionary computa-
tion conference, pages 1493–1504. Springer.
Wu, S., Yao, J., Fu, H., Tian, Y., Qian, C., Yang, Y.,
FU, Q., and Wei, Y. (2023). Quality-similar diversity
via population based reinforcement learning. In The
Eleventh International Conference on Learning Rep-
resentations.
Wurman, P. R., Barrett, S., Kawamoto, K., MacGlashan, J.,
Subramanian, K., Walsh, T. J., Capobianco, R., De-
vlic, A., Eckert, F., Fuchs, F., et al. (2022). Outracing
champion gran turismo drivers with deep reinforce-
ment learning. Nature, 602(7896):223–228.
Zolfagharian, A., Abdellatif, M., Briand, L. C.,
Bagherzadeh, M., and Ramesh, S. (2023). A
search-based testing approach for deep reinforcement
learning agents. IEEE Transactions on Software
Engineering, 49(7):3715–3735.
ECTA 2024 - 16th International Conference on Evolutionary Computation Theory and Applications
138