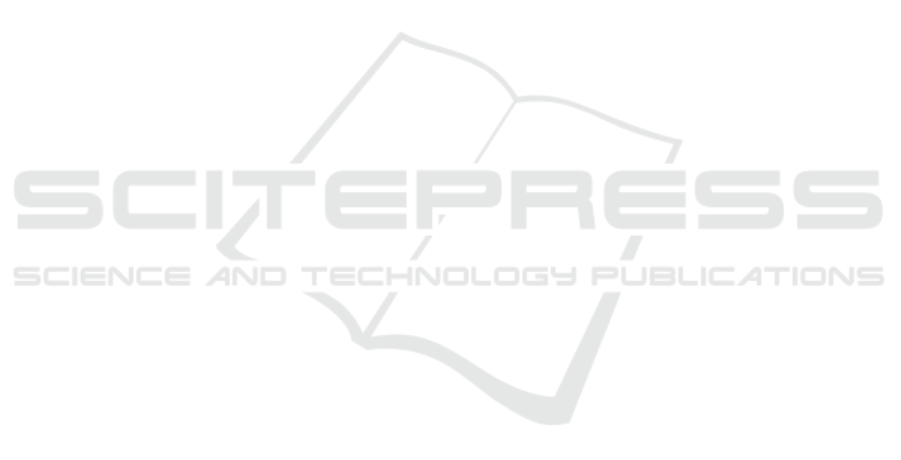
6 CONCLUDING REMARKS
Here we have investigated a Hopfield-type model
and, in particular, the model proposed in (Grinstein
and Linsker, 2005), being a simple prototype of bio-
inspired neural model. This includes bio-inspired
features, such as the refractory time and the maxi-
mum firing time that can be tuned and interpreted,
in the context of AI, as hyper-parameters. A partic-
ularly interesting bio-inspired feature is also encoded
in the learning mechanism of Hopfield-type networks,
which is exactly the Hebbian bio-inspired, unsuper-
vised, learning. The network topology is recognized
to also play a central role in both global network dy-
namics and learning efficiency. This last feature is of
particular interest in the AI field.
In particular, some authors focused on the effect
of topological structure on learning features (Kaviani
and Sohn, 2021), in some cases finding a better learn-
ing performance associated with specific topologies,
e.g., scale-free and/or small-world (Lu et al., 2023).
The present work represents a preliminary investiga-
tion of the relationships among connectivity features
and temporal complexity of a simple spiking neural
network without learning algorithms. Interestingly,
different topological structures can give similar dy-
namical behaviours and complexity features (see, e.g,
Fig. 4b, panel (9), with Fig. 5b, panel (8), and Fig.
3a, panels (1) and (4)).
Regarding the relationship between connectivity
and temporal complexity, further investigations are
needed to better understand the reason why very dif-
ferent topologies can give similar complexity. We ex-
pect these further investigations to deepen the under-
standing of the relationship between dynamical fea-
tures of the network, e.g., temporal complexity, con-
nectivity structure and learning features, such as stor-
age capacity.
We also plan future investigations regarding the re-
lationship between connectivity structure and learn-
ing algorithms, to study how the performance mea-
sures, jointly with the evaluation of complexity in-
dices, change with the number of stored patterns (e.g.,
in the Hopfield model).
7 FUNDING
This work was supported by the Next-Generation-
EU programme under the funding schemes PNRR-
PE-AI scheme (M4C2, investment 1.3, line on AI)
FAIR “Future Artificial Intelligence Research”, grant
id PE00000013, Spoke-8: Pervasive AI.
REFERENCES
Adjodah, D., Calacci, D., Dubey, A., Goyal, A., Krafft,
P., Moro, E., and Pentland, A. (2020). Leveraging
communication topologies between learning agents in
deep reinforcement learning. volume 2020-May, page
1738 – 1740.
Akin, O., Paradisi, P., and Grigolini, P. (2009).
Perturbation-induced emergence of poisson-like be-
havior in non-poisson systems. J. Stat. Mech.: Theory
Exp., page P01013.
Albert, R. and Barab
´
asi, A.-L. (2002). Statistical mechanics
of complex networks. Reviews of Modern Physics,
74(1):47 – 97.
Allegrini, P., Menicucci, D., Bedini, R., Gemignani, A., and
Paradisi, P. (2010). Complex intermittency blurred
by noise: theory and application to neural dynamics.
Phys. Rev. E, 82(1 Pt 2):015103.
Allegrini, P., Paradisi, P., Menicucci, D., Laurino, M., Be-
dini, R., Piarulli, A., and Gemignani, A. (2013). Sleep
unconsciousness and breakdown of serial critical in-
termittency: New vistas on the global workspace.
Chaos Solitons Fract., 55:32–43.
Allegrini, P., Paradisi, P., Menicucci, D., Laurino, M., Pi-
arulli, A., and Gemignani, A. (2015). Self-organized
dynamical complexity in human wakefulness and
sleep: Different critical brain-activity feedback for
conscious and unconscious states. Phys. Rev. E Stat.
Nonlin. Soft Matter Phys, 92(3).
Barab
´
asi, A.-L. and Albert, R. (1999). Emergence of scal-
ing in random networks. Science, 286(5439):509 –
512.
Barab
´
asi, A.-L. and Oltvai, Z. N. (2004). Network biology:
Understanding the cell’s functional organization. Na-
ture Reviews Genetics, 5(2):101 – 113.
Beggs, J. M. and Plenz, D. (2003). Neuronal avalanches
in neocortical circuits. Journal of neuroscience,
23(35):11167–11177.
Bianco, S., Grigolini, P., and Paradisi, P. (2007). A fluctu-
ating environment as a source of periodic modulation.
Chem. Phys. Lett., 438(4-6):336–340.
Boccaletti, S., Latora, V., Moreno, Y., Chavez, M., and
Hwang, D.-U. (2006). Complex networks: Structure
and dynamics. Phys. Rep., 424(4-5):175–308. DOI:
10.1016/j.physrep.2005.10.009.
Cox, D. (1970). Renewal Processes. Methuen & Co., Lon-
don. ISBN: 0-412-20570-X; first edition 1962.
Davies, M., Srinivasa, N., Lin, T.-H., Chinya, G., Cao, Y.,
Choday, S. H., Dimou, G., Joshi, P., Imam, N., Jain,
S., Liao, Y., Lin, C.-K., Lines, A., Liu, R., Math-
aikutty, D., McCoy, S., Paul, A., Tse, J., Venkatara-
manan, G., Weng, Y.-H., Wild, A., Yang, Y., and
Wang, H. (2018). Loihi: A neuromorphic many-
core processor with on-chip learning. IEEE Micro,
38(1):82 – 99.
Erd ¨os, P. and R
´
enyi, A. (1959). On random graphs i. Pub-
licationes Mathematcae, 6(3-4):290–297.
Ernst, I. (1925). Beitrag zur theorie des ferromag-
netismus. Zeitschrift f
¨
ur Physik A Hadrons and Nu-
clei, 31(1):253–258.
Temporal Complexity of a Hopfield-Type Neural Model in Random and Scale-Free Graphs
447