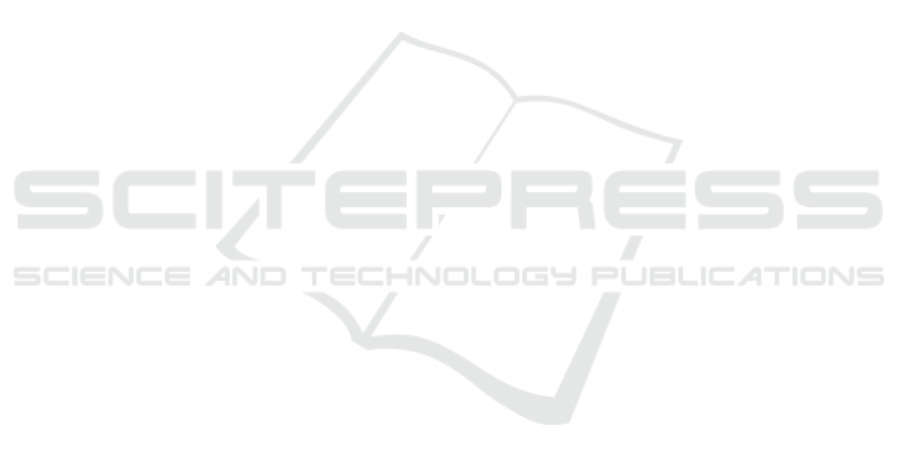
of multi-objective evolutionary algorithms, volume 1.
World Scientific.
Deb, K., Bhaskara Rao N., U., and Karthik, S. (2007).
Dynamic multi-objective optimization and decision-
making using modified nsga-ii: A case study on
hydro-thermal power scheduling. In International
Conference on Evolutionary Multi-Criterion Opti-
mization, pages 803–817. Springer.
Deb, K. and Jain, H. (2014). An evolutionary many-
objective optimization algorithm using reference-
point-based nondominated sorting approach, part i:
Solving problems with box constraints. IEEE Trans-
actions on Evolutionary Computation, 18(4):577–
601.
Farina, M. and Amato, P. (2002). On the optimal solution
definition for many-criteria optimization problems. In
2002 Annual Meeting of the North American Fuzzy In-
formation Processing Society Proceedings. NAFIPS-
FLINT 2002 (Cat. No. 02TH8622), pages 233–238.
Farina, M., Deb, K., and Amato, P. (2004). Dynamic multi-
objective optimization problems: Test cases, approxi-
mations, and applications. Evolutionary Computation,
IEEE Transactions on, 8:425 – 442.
Geladi, P. and Linderholm, J. (2020). 2.03 - principal com-
ponent analysis. In Brown, S., Tauler, R., and Wal-
czak, B., editors, Comprehensive Chemometrics (Sec-
ond Edition), pages 17–37. Elsevier, Oxford, second
edition edition.
Goh, C.-K. and Tan, K. C. (2009). A competitive-
cooperative coevolutionary paradigm for dynamic
multiobjective optimization. IEEE Transactions on
Evolutionary Computation, 13(1):103–127.
Gupta, R. and Nanda, S. J. (2021). Solving dynamic many-
objective tsp using nsga-iii equipped with svr-rbf ker-
nel predictor. In 2021 IEEE Congress on Evolutionary
Computation (CEC), pages 95–102.
Jiang, M., Huang, Z., Qiu, L., Huang, W., and Yen, G. G.
(2017). Transfer learning-based dynamic multiobjec-
tive optimization algorithms. IEEE Transactions on
Evolutionary Computation, 22(4):501–514.
Jiang, S., Wang, Y., Hu, Y., Zhang, Q., and Yang, S. (2023).
Vector autoregressive evolution for dynamic multi-
objective optimisation.
Jiang, S. and Yang, S. (2014). A framework of scalable dy-
namic test problems for dynamic multi-objective opti-
mization. In 2014 IEEE Symposium on Computational
Intelligence in Dynamic and Uncertain Environments
(CIDUE), pages 32–39.
Jin, J. and Chen, Y. (2013). Var-based research on energy
consumption in china. In 2013 International Con-
ference on Computational and Information Sciences,
pages 1746–1749.
Jin, Y. and Branke, J. (2005). Evolutionary optimization in
uncertain environments-a survey. Trans. Evol. Comp,
9(3):303–317.
Jonathan D. Cryer, K.-S. C. (2008). Introduction, pages 1–
10. Springer New York, New York, NY.
Karkazan, K., Topcuoglu, H. R., and Sahmoud, S. (2023).
A new prediction-based algorithm for dynamic multi-
objective optimization problems. In International
Conference on the Applications of Evolutionary Com-
putation (Part of EvoStar), pages 194–209. Springer.
Li, H. and Zhang, Q. (2009). Multiobjective optimization
problems with complicated pareto sets, moea/d and
nsga-ii. IEEE Transactions on Evolutionary Compu-
tation, 13(2):284–302.
Li, L. D., Li, X., and Yu, X. (2008). Power generation load-
ing optimization using a multi-objective constraint-
handling method via pso algorithm. In 2008 6th IEEE
International Conference on Industrial Informatics,
pages 1632–1637.
S. Huband, P. Hingston, L. B. and While, L. (2006). A
review of multiobjective test problems and a scalable
test problem toolkit. IEEE Transactions on Evolution-
ary Computation, 10(5):477–506.
Sahmoud, S. and Topcuoglu, H. R. (2018). A type detec-
tion based dynamic multi-objective evolutionary algo-
rithm. In International Conference on the Applica-
tions of Evolutionary Computation, pages 879–893.
Springer.
Sahmoud, S. and Topcuoglu, H. R. (2019). Exploiting
characterization of dynamism for enhancing dynamic
multi-objective evolutionary algorithms. Applied Soft
Computing, 85:105783.
Sahmoud, S. and Topcuoglu, H. R. (2020). Memory-
assisted dynamic multi-objective evolutionary algo-
rithm for feature drift problem. In 2020 IEEE
Congress on Evolutionary Computation (CEC), pages
1–8. IEEE.
Tian, Y., Cheng, R., Zhang, X., and Jin, Y. (2017).
PlatEMO: A MATLAB platform for evolutionary
multi-objective optimization. IEEE Computational
Intelligence Magazine, 12(4):73–87.
Weizhen, H., Jiang, M., Gao, X., Tan, K. C., and Cheung,
Y.-m. (2019). Solving dynamic multi-objective opti-
mization problems using incremental support vector
machine. In 2019 IEEE Congress on Evolutionary
Computation (CEC), pages 2794–2799. IEEE.
Zhang, Q. and Li, H. (2007). Moea/d: A multiob-
jective evolutionary algorithm based on decomposi-
tion. IEEE Transactions on Evolutionary Computa-
tion, 11(6):712–731.
Zhu, X., Zhang, J., and Wu, Z. (2019). Many-objective
power flow optimization problems based on an im-
proved moea/d with dynamical resource allocation
strategy. In 2019 Chinese Automation Congress
(CAC), pages 3680–3685.
A Vector Autoregression-Based Algorithm for Dynamic Many-Objective Optimization Problems
287