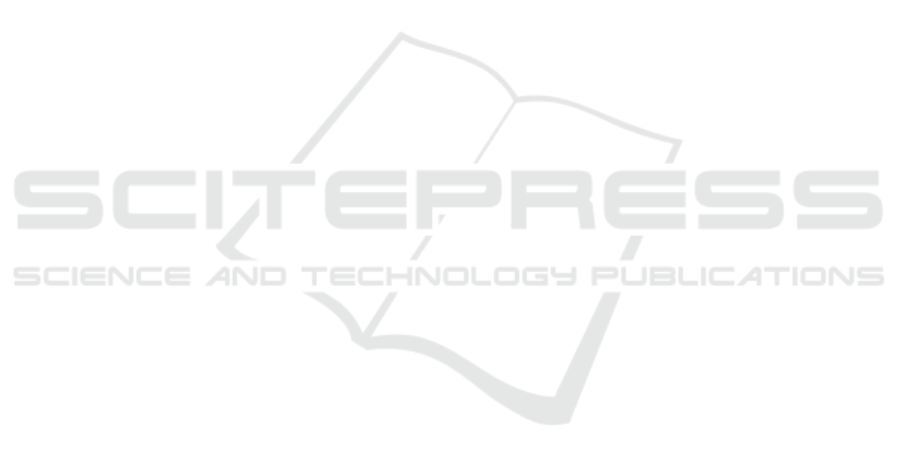
tional farm. This controlled environment will allow us
to rigorously evaluate its performance and fine-tune
its components. Future work will focus on assessing
the scalability of our SDF-based approach for appli-
cation in diverse farming contexts. We will also ex-
plore potential collaborations to refine and implement
the solution on a larger scale and evaluate the social
impact of our technology, particularly its potential to
improve farmer livelihoods and promote sustainable
agricultural practices.
REFERENCES
Cheng, W., andXiaoting Wang, T. M., and Wang,
G. (2022). Anomaly detection for internet of
things time series data using generative adversar-
ial networks with attention mechanism in smart
agriculture. Frontiers in Plant Science, page
https://doi.org/10.3389/fpls.2022.890563.
Feng, Q., Wang, S., Wang, H., Qin, Z., and Wang,
H. (2022a). Circle fitting based image segmen-
tation and multi-scale block local binary pattern
based distinction of ring rot and anthracnose on
apple fruits. Frontiers in Plant Science, 13:doi:
10.3389/fpls.2022.884891.
Feng, Z., Wei, Q., Ye, Z., Yang, B., Gao, Y., Lv, J.,
Dai, Y., Bao, J., and Yao, Q. (2022b). Vibrational
courtship disruption of nilaparvata lugens using arti-
ficial disruptive signals. Frontiers in Plant Science,
13:https://doi.org/10.3389/fpls.2022.897475.
Fu, L., Li, S., Rao, Y., Liang, J., Teng, J., and He, Q. (2022).
A novel heuristic target-dependent neural architecture
search method with small samples. Frontiers in Plant
Science, page 10.3389/fpls.2022.897883.
Maia, R. F., Lurbe, C. B., and Hornbuckle, J. (2022).
Machine learning approach to estimate soil matric
potential in the plant root zone based on remote
sensing data. Frontiers in Plant Science, page
https://doi.org/10.3389/fpls.2022.931491.
MathWorks, I. (2024). Data collection in the cloud with
advanced data analysis using matlab.
Schmidhuber, J. (2015). Deep learning in neural networks:
An overview. neural networks. Official Journal
of the International Neural Network Society, 61,
https://doi.org/10.1016/j.neunet.2014.09.003:pages
85–117.
Sellam, V. and Poovammal, E. (2010). Prediction of crop
yield using regression analysis. IEEE Trans. Knowl.
Data Eng., pages vol. 23, no. 10, pp. 1498–1512.
Sun, G., Liu, S., Luo, H., Feng, Z., Yang, B., Luo, J., Tang,
J., Yao, Q., and Xu, J. (2022a). Intelligent monitor-
ing system of migratory pests based on searchlight
trap and machine vision. Frontiers in Plant Science,
13:https://doi.org/10.3389/fpls.2022.897739.
Sun, M., Xu1, L., Luo, R., Lu, Y., and Jia, W. (2022b).
Fast location and recognition of green apple based
on rgb-d image. Frontiers in Plant Science, 13:doi:
10.3389/fpls.2022.864458.
Umargono, E., Suseno, J. E., and K., V. G. S. (2020).
K-means clustering optimization using the elbow
method and early centroid determination based-on
mean and median. International Conferences on In-
formation System and Technology (CONRIST 2019,
DOI: 10.5220/0009908402340240:pages 234–240.
Uyeh, D. D., Iyiola, O., Mallipeddi, R., Asem-Hiablie,
S., Amaizu, M., Ha, Y., and Park, T. (2022). Grid
search for lowest root mean squared error in pre-
dicting optimal sensor location in protected culti-
vation systems. Frontiers in Plant Science, page
https://doi.org/10.3389/fpls.2022.920284.
Yates, R. D. and Goodman, D. J. (2005). Probability
and Stochastic Processes: A Friendly Introduction for
Electrical and Computer Engineers. John Wiley and
Sons, INC, Rutgers, The State University of New Jer-
sey.
Zhang, L., Lei, L., and Yan, D. (2010). Comparison of
two regression models for predicting crop yield. IEEE
International Symposium on Geoscience and Remote
Sensing (IGARSS).
Zingade, P. D. S., Buchade, O., Mehta, N., Ghodekar, S.,
and Mehta, C. (2018). Machine learning-based crop
prediction system using multi-linear regression. Inter-
national Journal of Emerging Technology and Com-
puter Science (IJETCS), pages Vol 3, Issue 2.
Combating Agricultural Challenges with Secure Digital Farming
383