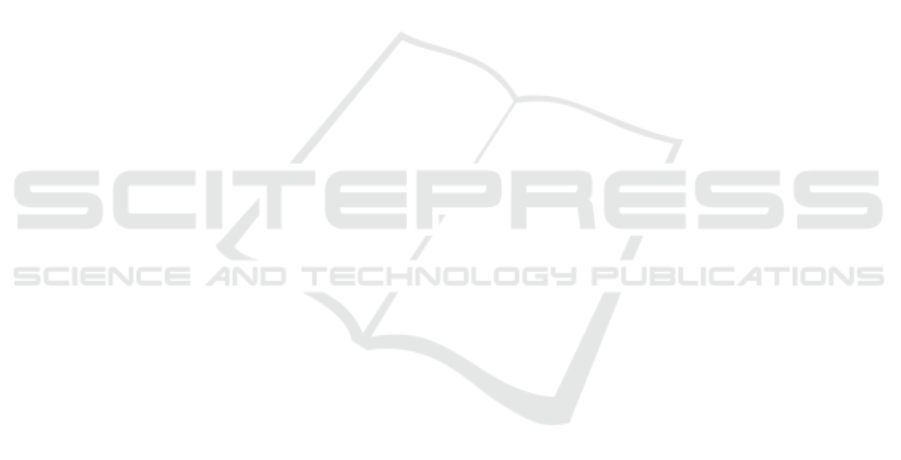
(2019). Residual Reinforcement Learning for Robot
Control. In Proc. IEEE Int. Conf. Robot. Automat.,
pages 6023–6029.
Khader, S. A., Yin, H., Falco, P., and Kragic, D.
(2021). Stability-Guaranteed Reinforcement Learn-
ing for Contact-Rich Manipulation. IEEE Robot. Au-
tomat. Lett., 6(1):1–8.
Khansari, M., Kronander, K., and Billard, A. (2014). Mod-
eling robot discrete movements with state-varying
stiffness and damping: A framework for integrated
motion generation and impedance control. In Proc.
Robot. Sci. Syst.
Khatib, O. (1987). A unified approach for motion and force
control of robot manipulators: The operational space
formulation. IEEE J. Robot. Automat., 3(1):43–53.
Lillicrap, T. P., Hunt, J. J., Pritzel, A., Heess, N., Erez, T.,
Tassa, Y., Silver, D., and Wierstra, D. (2016). Con-
tinuous control with deep reinforcement learning. In
Proc. Int. Conf. Learn. Represent. Art. no. 149803.
Liu, X., Ge, S. S., Zhao, F., and Mei, X. (2021). Optimized
Interaction Control for Robot Manipulator Interact-
ing With Flexible Environment. IEEE/ASME Trans.
Mechatron., 26(6):2888–2898.
Lloyd, S., Irani, R. A., and Ahmadi, M. (2024). Precision
robotic deburring with Simultaneous Registration and
Machining for improved accuracy, quality, and effi-
ciency. Robot. Computer-Integr. Manufact., 88. Art.
no. 102733.
Luo, J., Solowjow, E., Wen, C., Ojea, J. A., Agogino, A. M.,
Tamar, A., and Abbeel, P. (2019). Reinforcement
Learning on Variable Impedance Controller for High-
Precision Robotic Assembly. In Proc. IEEE Int. Conf.
Robot. Automat., pages 3080–3087.
Makoviychuk, V., Wawrzyniak, L., Guo, Y., Lu, M., Storey,
K., Macklin, M., Hoeller, D., Rudin, N., Allshire,
A., Handa, A., and State, G. (2021). Isaac Gym:
High Performance GPU-Based Physics Simulation
For Robot Learning. In Proc. Adv. Neural Inform. Pro-
cess. Syst., volume 1.
Matschek, J., Bethge, J., and Findeisen, R. (2023). Safe
Machine-Learning-Supported Model Predictive Force
and Motion Control in Robotics. IEEE Trans. Contr.
Syst. Technol., 31(6):2380–2392.
Merhi, M. I. and Harfouche, A. (2023). Enablers of artificial
intelligence adoption and implementation in produc-
tion systems. Int. J. Prod. Res., 62(15):5457–5471.
Mnih, V., Kavukcuoglu, K., Silver, D., Graves, A.,
Antonoglou, I., Wierstra, D., and Riedmiller, M.
(2013). Playing Atari with Deep Reinforcement
Learning. arXiv preprint: 1312.5602.
Narang, Y., Storey, K., Akinola, I., Macklin, M., Reist, P.,
Wawrzyniak, L., Guo, Y., Moravanszky, A., State, G.,
Lu, M., Handa, A., and Fox, D. (2022). Factory: Fast
Contact for Robotic Assembly. In Proc. Robot. Sci.
Syst.
Newman, W. S. (1992). Stability and Performance Limits
of Interaction Controllers. J. Dyn. Syst. Meas. Contr.,
114(4):563–570.
Petrone, V., Puricelli, L., Pozzi, A., Ferrentino, E., Chi-
acchio, P., Braghin, F., and Roveda, L. (2024).
Optimized Residual Action for Interaction Control
with Learned Environments. TechRxiv Preprint:
21905433.v2.
Pozzi, A., Puricelli, L., Petrone, V., Ferrentino, E., Chiac-
chio, P., Braghin, F., and Roveda, L. (2023). Exper-
imental Validation of an Actor-Critic Model Predic-
tive Force Controller for Robot-Environment Interac-
tion Tasks. In Proc. Int. Conf. Inform. Contr. Automat.
Robot., volume 1, pages 394–404.
Schulman, J., Wolski, F., Dhariwal, P., Radford, A., and
Klimov, O. (2017). Proximal Policy Optimization Al-
gorithms. arXiv preprint: 1707.06347.
Sørensen, L. C., Buch, J. P., Petersen, H. G., and Kraft, D.
(2016). Online Action Learning using Kernel Density
Estimation for Quick Discovery of Good Parameters
for Peg-in-Hole Insertion. In Proc. Int. Conf. Inform.
Contr. Automat. Robot., volume 2, pages 166–177.
Tang, B., Lin, M. A., Akinola, I. A., Handa, A., Sukhatme,
G. S., Ramos, F., Fox, D., and Narang, Y. S.
(2023a). IndustReal: Transferring Contact-Rich As-
sembly Tasks from Simulation to Reality. In Proc.
Robot. Sci. Syst.
Tang, Z., Wang, P., Xin, W., Xie, Z., Kan, L., Mohanakr-
ishnan, M., and Laschi, C. (2023b). Meta-Learning-
Based Optimal Control for Soft Robotic Manipula-
tors to Interact with Unknown Environments. In Proc.
IEEE Int. Conf. Robot. Automat., pages 982–988.
Todorov, E., Erez, T., and Tassa, Y. (2012). MuJoCo:
A physics engine for model-based control. In Proc.
IEEE Int. Conf. Intell. Robots Syst., pages 5026–5033.
Todorov, E. and Li, W. (2005). A generalized iterative LQG
method for locally-optimal feedback control of con-
strained nonlinear stochastic systems. In Proc. Am.
Contr. Conf., volume 1, pages 300–306.
Unten, H., Sakaino, S., and Tsuji, T. (2023). Peg-in-
Hole Using Transient Information of Force Response.
IEEE/ASME Trans. Mechatron., 28(3):1674–1682.
Xu, L. D., Xu, E. L., and Li, L. (2018). Industry 4.0:
state of the art and future trends. Int. J. Prod. Res.,
56(8):2941–2962.
Yang, F. and Gu, S. (2021). Industry 4.0, a revolution that
requires technology and national strategies. Compl.
Intell. Syst., 7(3):1311–1325.
Zhang, H., Solak, G., Lahr, G. J. G., and Ajoudani, A.
(2024). SRL-VIC: A Variable Stiffness-Based Safe
Reinforcement Learning for Contact-Rich Robotic
Tasks. IEEE Robot. Automat. Lett., 9(6):5631–5638.
Zhang, K., Wang, C., Chen, H., Pan, J., Wang, M. Y.,
and Zhang, W. (2023). Vision-based Six-Dimensional
Peg-in-Hole for Practical Connector Insertion. In
Proc. IEEE Int. Conf. Robot. Automat., pages 1771–
1777.
Zhang, X., Sun, L., Kuang, Z., and Tomizuka, M. (2021).
Learning Variable Impedance Control via Inverse Re-
inforcement Learning for Force-Related Tasks. IEEE
Robot. Automat. Lett., 6(2):2225–2232.
On the Role of Artificial Intelligence Methods in Modern Force-Controlled Manufacturing Robotic Tasks
399