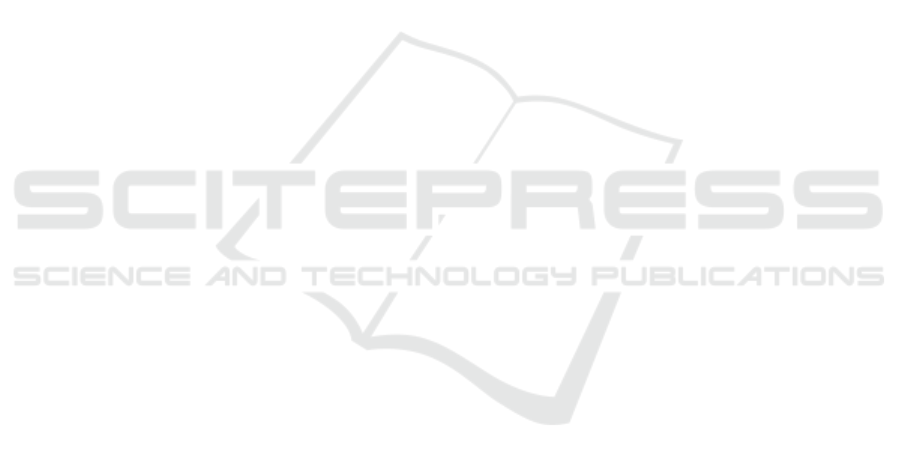
partner implementations in terms of human-likeness
and task performance. Presence, 20:173–189.
Franz, F., Edgar, E., Alebert-Georg, L., and Axel, B. (2007).
G*power 3: A flexible statistical power analysis pro-
gram for the social, behavioral, and biomedical sci-
ences. Behavior Research Methods, pages 175–191.
Ganesh, G., Takagi, A., Osu, R., Yoshioka, T., Kawato,
M., and Burdet, E. (2014). Two is better than one:
Physical interactions improve motor performance in
humans. Scientific Reports, 4.
Goodrich, M. and Schultz, A. (2007). Human-robot inter-
action: A survey. Foundations and Trends in Human-
Computer Interaction, 1:203–275.
Grynszpan, O., Saha
¨
ı, A., Hamidi, N., Pacherie, E., Berbe-
rian, B., Roche, L., and Saint-Bauzel, L. (2019). The
sense of agency in human-human vs human-robot
joint action. Consciousness and Cognition, 75.
Haggard, P. (2005). Conscious intention and motor cogni-
tion. Trends in Cognitive Sciences, 9:290–295.
Haggard, P., Clark, S., and Kalogeras, J. (2002). Volun-
tary action and conscious awareness. Nature Neuro-
science, 5:382–385.
Howard, E. E., Edwards, S. G., and Bayliss, A. P. (2016).
Physical and mental effort disrupts the implicit sense
of agency. Cognition, 157:114–125.
Ivanova, E., Carboni, G., Eden, J., Kr
¨
uger, J., and Burdet,
E. (2020). For motion assistance humans prefer to
rely on a robot rather than on an unpredictable human.
IEEE Open Journal of Engineering in Medicine and
Biology, 1:133–139.
Jarrasse, N., Sanguineti, V., Burdet, E., and Jarrass
´
e, N.
(2013). Slaves no longer: review on role assignment
for human-robot joint motor action. Adaptive Behav-
ior, 22:70–82. ¡br/¿.
Li, Y., Carboni, G., Gonzalez, F., Campolo, D., and Burdet,
E. (2019). Differential game theory for versatile phys-
ical human–robot interaction. Nature Machine Intelli-
gence, 1:36–43.
Madan, C. E., Kucukyilmaz, A., Sezgin, T. M., and Bas-
dogan, C. (2015). Recognition of haptic interaction
patterns in dyadic joint object manipulation. IEEE
Transactions on Haptics, 8:54–66.
Mielke, E. A., Townsend, E. C., and Killpack, M. D. (2017).
Analysis of rigid extended object co-manipulation by
human dyads: Lateral movement characterization.
M
¨
ortl, A., Lawitzky, M., Kucukyilmaz, A., Sezgin, M., Bas-
dogan, C., and Hirche, S. (2012). The role of roles:
Physical cooperation between humans and robots. In-
ternational Journal of Robotics Research, 31:1656–
1674.
Obhi, S. S. and Hall, P. (2011). Sense of agency and in-
tentional binding in joint action. Experimental Brain
Research, 211:655–662.
Parker, C. A. C. and Croft, E. A. (2011). Experimen-
tal investigation of human-robot cooperative carrying.
pages 3361–3366. Institute of Electrical and Electron-
ics Engineers (IEEE).
Peshkin, M., Colgate, J., Wannasuphoprasit, W., Moore, C.,
Gillespie, R., and Akella, P. (2001). Cobot architec-
ture. IEEE Transactions on Robotics and Automation,
17(4):377–390.
Pezzulo, G., Roche, L., and Saint-Bauzel, L. (2021). Haptic
communication optimises joint decisions and affords
implicit confidence sharing. Scientific Reports, 11.
Reed, K. and Peshkin, M. (2008). Physical collaboration
of human-human and human-robot teams. Haptics,
IEEE Transactions on, 1:108–120.
Roche, L., Richer, F., and Saint-Bauzel, L. (2018). The
semaphoro haptic interface: a real-time low-cost
open-source implementation for dyadic teleoperation.
Roche, L. and Saint-Bauzel, L. (2021). Study of kines-
thetic negotiation ability in lightweight comanipula-
tive decision-making tasks: Design and study of a vir-
tual partner based on human-human interaction obser-
vation.
Takagi, A., Ganesh, G., Yoshioka, T., Kawato, M., and Bur-
det, E. (2017). Physically interacting individuals es-
timate the partner’s goal to enhance their movements.
Nature Human Behaviour, 1.
Takagi, A., Usai, F., Ganesh, G., Sanguineti, V., and Burdet,
E. (2018). Haptic communication between humans
is tuned by the hard or soft mechanics of interaction.
PLoS Computational Biology, 14.
van der Wel R. P. R. D., G., K., and Sebanz, N. (2011). Let
the force be with us: Dyads exploit haptic coupling for
coordination. Journal of Experimental Psychology:
Human Perception and Performance, 37(5):1420–
1431.
Study of the Influence of a Force Bias on a Robotic Partner During Kinesthetic Communication
407