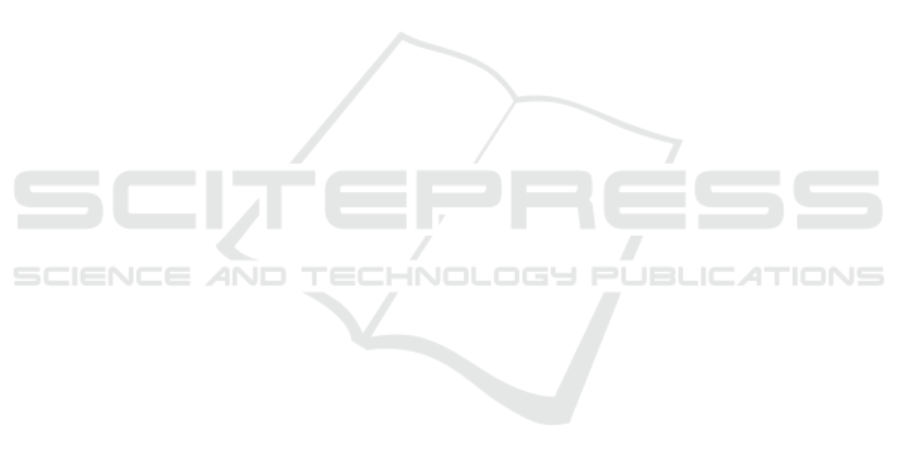
ent Boosting Survival Analysis (GBSA), and Survival
Support Vector Machines (FS-SVM), on datasets
from both Gorenje and Microsoft Azure.
The results reveal that survival methods can be
highly effective for predicting maintenance needs in
an industrial setting. As it was seen, the Cox model
showed strong performance on test data, although it
exhibited overfitting, which necessitates further inves-
tigation and validation. The RSF model displayed
good generalization in some components but also
signs of overfitting in others, indicating the need for
model adjustments. The GBSA model consistently
performed well across different datasets, suggesting
it is a robust choice for generalizable predictive main-
tenance tasks. Meanwhile, FS-SVM showed poten-
tial, especially in specific test scenarios, though it may
benefit from further refinement.
A key advantage of using these survival analy-
sis models is the ability to generate Remaining Use-
ful Life (RUL) estimates, which are strongly impor-
tant for planning maintenance activities. The simula-
tion and dashboard interface developed using Stream-
lit provided a practical tool for visualizing these pre-
dictions, allowing maintenance schedules to be opti-
mized to minimize costs and downtime. The inter-
face facilitated real-time decision-making by enabling
users to simulate various scenarios and view detailed
reports on component health and predicted failures.
Despite the promising results, there are several ar-
eas for future work to enhance the application of pre-
dictive maintenance, which can be highlighted:
• Real-Time Data Integration: Implementing mod-
els that can handle real-time data streams will im-
prove the timeliness and accuracy of maintenance
predictions. This integration will also allow for
dynamic updating of RUL estimates and more re-
sponsive maintenance planning.
• Enhanced User Interface: Developing a more
complex and detailed user interface could provide
additional insights and functionality, such as more
granular control over simulation parameters and
detailed visualizations of component health trends
over time.
• Advanced Model Refinement: Further refinement
of models, particularly those showing signs of
overfitting or underperformance, could involve
hybrid approaches that combine the strengths of
multiple models or the integration of additional
data sources to improve prediction accuracy. The
use of other performance measures, such as the
Brier Score mentioned in section 2.4, can help to
compare the results obtained in the future.
• Scalability Testing: Testing the models and simu-
lation framework in larger, more diverse industrial
settings will ensure the scalability and robustness
of the solutions proposed.
In conclusion, this study emphasises the potential
of survival analysis methods in improving predictive
maintenance within Industry 4.0 environments. By
using advanced predictive models and user-friendly
interfaces, industries can move towards more effi-
cient, cost-effective, and reliable maintenance strate-
gies. Continued research and development in this
field will further solidify these methods’ role in the
next generation of intelligent and efficient mainte-
nance.
ACKNOWLEDGEMENTS
This work was partially supported by the
HORIZONCL4-2021-TWIN-TRANSITION-01
openZDM project, under Grant Agreement No.
101058673.
REFERENCES
Achouch, M., Dimitrova, M., Ziane, K., Sattarpanah Kar-
ganroudi, S., Dhouib, R., Ibrahim, H., and Adda, M.
(2022). On Predictive Maintenance in Industry 4.0:
Overview, Models, and Challenges. Applied Sciences,
12(16):8081.
Arena, F., Collotta, M., Luca, L., Ruggieri, M., and Ter-
mine, F. G. (2021). Predictive Maintenance in the Au-
tomotive Sector: A Literature Review. Mathematical
and Computational Applications, 27(1):2.
De Santis, R. B., Gontijo, T. S., and Costa, M. A. (2022).
A Data-Driven Framework for Small Hydroelectric
Plant Prognosis Using Tsfresh and Machine Learning
Survival Models. Sensors, 23(1):12.
Ferreira, C. and Gonc¸alves, G. (2022). Remaining Use-
ful Life prediction and challenges: A literature review
on the use of Machine Learning Methods. Journal of
Manufacturing Systems, 63:550–562.
Frumosu, F. D., Rønsch, G. Ø., and Kulahci, M. (2020).
Mould wear-out prediction in the plastic injec-
tion moulding industry: a case study. Interna-
tional Journal of Computer Integrated Manufactur-
ing, 33(12):1245–1258.
Gupta, S., Kumar, A., and Maiti, J. (2024). A critical review
on system architecture, techniques, trends and chal-
lenges in intelligent predictive maintenance. Safety
Science, 177:106590.
Madasamy, S., Shankar, B. P., Yadav, R. K., and P, J. K.
(2023). A Machine Learning Approach in Predic-
tive Maintenance in the IoT Enabled Industry 4.0. In
2023 4th International Conference on Smart Electron-
ics and Communication (ICOSEC), pages 418–423,
Trichy, India. IEEE.
Data-Driven Predictive Maintenance for Component Life-Cycle Extension
135