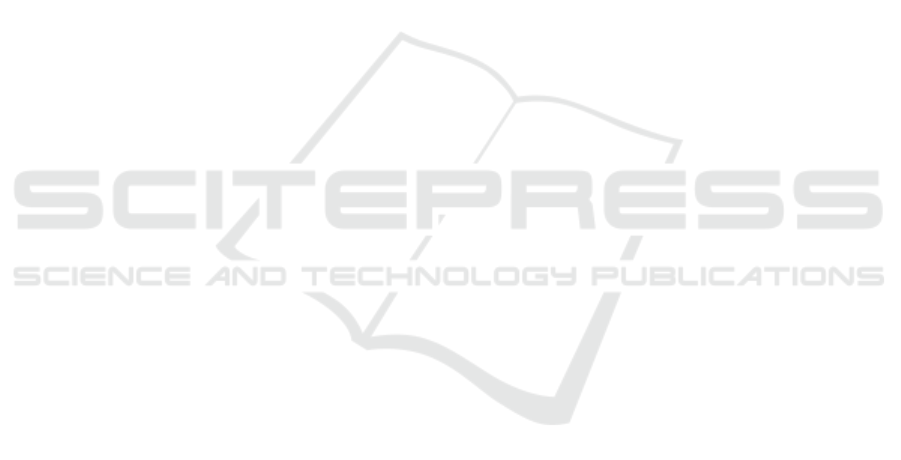
In future research, we aim to expand the analy-
sis to datasets that include a broader range of physi-
ological signals, enhancing the robustness and gener-
alizability of the engagement prediction models. Ad-
ditionally, exploring the real-time implementation of
these models within e-learning platforms will be a
crucial step towards creating more personalized and
responsive learning environments.
REFERENCES
Weka. https://www.weka.io/. (accessed on 17 July 2024).
Aggarwal, S., Lamba, M., Verma, K., Khuttan, S., and Gau-
tam, H. (2021). A preliminary investigation for assess-
ing attention levels for massive online open courses
learning environment using eeg signals: An experi-
mental study. Human Behavior and emerging tech-
nologies, 3(5):933–941.
Al-Nafjan, A. and Aldayel, M. (2022). Predict students’
attention in online learning using eeg data. Sustain-
ability, 14(11):6553.
Ayyadevara, V. K. and Ayyadevara, V. K. (2018). Gradient
boosting machine. Pro machine learning algorithms:
A hands-on approach to implementing algorithms in
python and R, pages 117–134.
Chrysanthakopoulou, A., Dritsas, E., Trigka, M., and My-
lonas, P. (2023). An eeg-based application for real-
time mental state recognition in adaptive e-learning
environment. In 2023 18th International Workshop
on Semantic and Social Media Adaptation & Person-
alization (SMAP) 18th International Workshop on Se-
mantic and Social Media Adaptation & Personaliza-
tion (SMAP 2023), pages 1–6. IEEE.
Dadebayev, D., Goh, W. W., and Tan, E. X. (2022). Eeg-
based emotion recognition: Review of commercial
eeg devices and machine learning techniques. Jour-
nal of King Saud University-Computer and Informa-
tion Sciences, 34(7):4385–4401.
Daghriri, T., Rustam, F., Aljedaani, W., Bashiri, A. H., and
Ashraf, I. (2022). Electroencephalogram signals for
detecting confused students in online education plat-
forms with probability-based features. Electronics,
11(18):2855.
Genuer, R., Poggi, J.-M., Genuer, R., and Poggi, J.-M.
(2020). Random forests. Springer.
Gurney, K. (2018). An introduction to neural networks.
CRC press.
Herbig, N., D
¨
uwel, T., Helali, M., Eckhart, L., Schuck, P.,
Choudhury, S., and Kr
¨
uger, A. (2020). Investigating
multi-modal measures for cognitive load detection in
e-learning. In Proceedings of the 28th ACM confer-
ence on user modeling, adaptation and personaliza-
tion, pages 88–97.
Lu, W. and Wang, Y. (2024). Logistic regression. In
Textbook of Medical Statistics: For Medical Students,
pages 181–189. Springer.
Maimaiti, B., Meng, H., Lv, Y., Qiu, J., Zhu, Z., Xie, Y.,
Li, Y., Zhao, W., Liu, J., Li, M., et al. (2022). An
overview of eeg-based machine learning methods in
seizure prediction and opportunities for neurologists
in this field. Neuroscience, 481:197–218.
Mejbri, N., Essalmi, F., Jemni, M., and Alyoubi, B. A.
(2022). Trends in the use of affective computing in
e-learning environments. Education and Information
Technologies, pages 1–23.
Naidu, G., Zuva, T., and Sibanda, E. M. (2023). A review of
evaluation metrics in machine learning algorithms. In
Computer Science On-line Conference, pages 15–25.
Springer.
Nandi, A., Xhafa, F., Subirats, L., and Fort, S. (2021). Real-
time emotion classification using eeg data stream in
e-learning contexts. Sensors, 21(5):1589.
Pathak, D. and Kashyap, R. (2022). Electroencephalogram-
based deep learning framework for the proposed so-
lution of e-learning challenges and limitations. In-
ternational Journal of Intelligent Information and
Database Systems, 15(3):295–310.
Pathak, D. and Kashyap, R. (2023). Evaluating e-learning
engagement through eeg signal analysis with convo-
lutional neural networks. In International Conference
on Computer & Communication Technologies, pages
225–239. Springer.
Pisner, D. A. and Schnyer, D. M. (2020). Support vector
machine. In Machine learning, pages 101–121. Else-
vier.
Trigka, M., Dritsas, E., and Fidas, C. (2022). A survey on
signal processing methods for eeg-based brain com-
puter interface systems. In Proceedings of the 26th
Pan-Hellenic Conference on Informatics, pages 213–
218.
Trigka, M., Dritsas, E., and Mylonas, P. (2023a). Mental
confusion prediction in e-learning contexts with eeg
and machine learning. In Novel & Intelligent Digital
Systems Conferences, pages 195–200. Springer.
Trigka, M., Dritsas, E., and Mylonas, P. (2024). New per-
spectives in e-learning: Eeg-based modelling of hu-
man cognition individual differences. In IFIP Inter-
national Conference on Artificial Intelligence Appli-
cations and Innovations, pages 290–299. Springer.
Trigka, M., Papadoulis, G., Dritsas, E., and Fidas, C.
(2023b). Influences of cognitive styles on eeg-based
activity: An empirical study on visual content com-
prehension. In IFIP Conference on Human-Computer
Interaction, pages 496–500. Springer.
WEBIST 2024 - 20th International Conference on Web Information Systems and Technologies
330