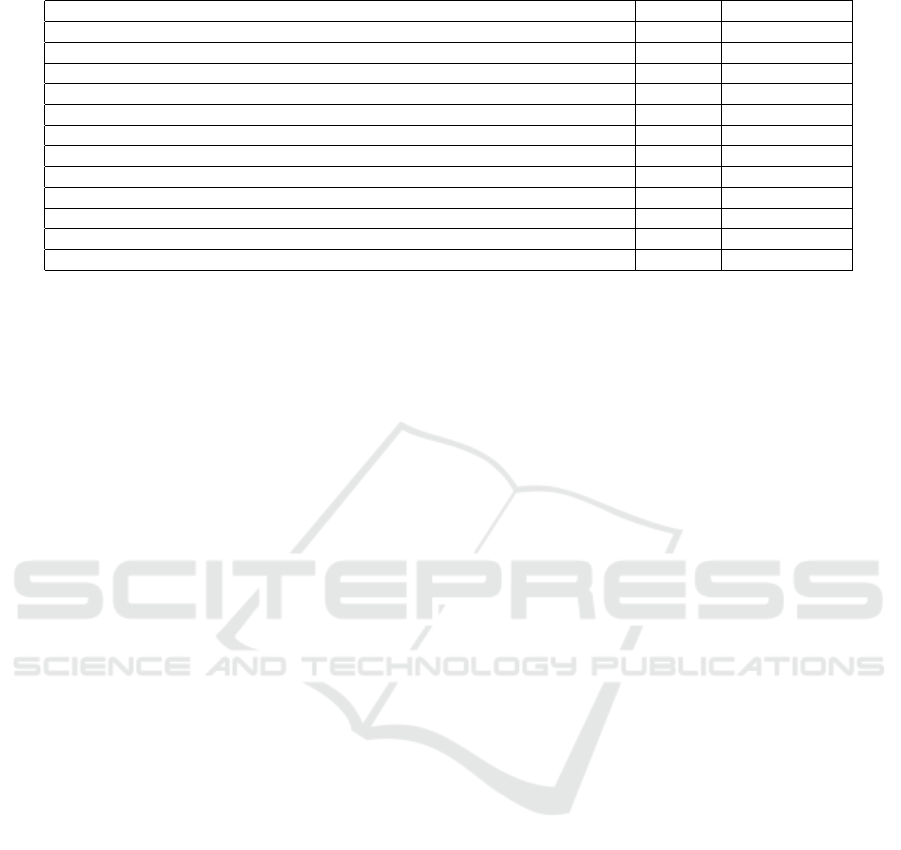
Table 7: ADA SEP – Mechatronic with High AI Impact and Corresponding Digital Skills Rate.
Area of activity AI Impact Digital Skills Rate
Programming Electronic Systems for Automation Control 4.53 17%
Installation of Electrical/Electronic Systems on Boats 3.82 7%
Manual and Automated Machine Forming 3.75 0%
Installation and Repair of TV Reception and Signal Systems 3.75 0%
Designing Renewable Energy Source (RES) Systems 3.73 27%
System Integration for Optimizing Aerospace Components and Vehicles Production 3.73 25%
Customer Installation, Commissioning, and Testing 3.71 12%
Design of Thermohydraulic Systems (e.g., civil, industrial, HVAC) 3.69 27%
Installation/Maintenance of Industrial Electrical Systems 3.61 14%
Management and Improvement of Aerospace Production Processes and Logistics 3.61 21%
Building Automation Systems Setup and Management 3.59 26%
Installation/Maintenance of Civil and Commercial Electrical Systems 3.57 11%
REFERENCES
Acemoglu, D., Autor, D., Hazell, J., and Restrepo, P.
(2022). Artificial intelligence and jobs: Evidence
from online vacancies. Journal of Labor Economics,
40(S1):S293–S340.
Alekseeva, L., Azar, J., Gin
´
e, M., Samila, S., and Taska, B.
(2021). The demand for ai skills in the labor market.
Labour economics, 71:102002.
Autor, D. H. (2015). Why are there still so many jobs? the
history and future of workplace automation. Journal
of economic perspectives, 29(3):3–30.
Brynjolfsson, E. and McAfee, A. (2014). The second ma-
chine age: Work, progress, and prosperity in a time of
brilliant technologies. WW Norton & Company.
Chen, T. and Guestrin, C. (2016). Xgboost: A scalable
tree boosting system. In Proceedings of the 22nd acm
sigkdd international conference on knowledge discov-
ery and data mining, pages 785–794.
Eloundou, T., Manning, S., Mishkin, P., and Rock, D.
(2023). Gpts are gpts: An early look at the labor mar-
ket impact potential of large language models. arXiv
preprint arXiv:2303.10130.
Enrique, F. M. and Matteo, S. (2024). Skewed signals?
confronting biases in online job ads data. Technical
report, Joint Research Centre.
Frey, C. B. and Osborne, M. A. (2017). The future of em-
ployment: How susceptible are jobs to computerisa-
tion? Technological forecasting and social change,
114:254–280.
Gatti, A. C., Colombo, E., Magrini, E., Perego, S., and
Pelucchi, M. (2022). Understanding talent attraction
using online job ads: the impact of artificial intelli-
gence and green jobs. In The Relevance of Artifi-
cial Intelligence in the Digital and Green Transfor-
mation of Regional and Local Labour Markets Across
Europe, pages 129–164. Nomos Verlagsgesellschaft
mbH & Co. KG.
Lane, M., Williams, M., and Broecke, S. (2023). The im-
pact of ai on the workplace: Main findings from the
oecd ai surveys of employers and workers.
Lovaglio, P. G. (2022). Do job vacancies variations antici-
pate employment variations by sector? some prelimi-
nary evidence from italy. Labour, 36(1):71–93.
Manca, F. (2023). Six questions about the demand for arti-
ficial intelligence skills in labour markets.
Mazzarella, R., Mallardi, F., and Porcelli, R. (2017). At-
lante lavoro: un modello a supporto delle politiche
dell’occupazione e dell’apprendimento permanente.
Sinappsi, 7:2–3.
Mezzanzanica, M., Mercorio, F., and Colombo, E. (2018).
Digitalisation and automation: Insights from the on-
line labour market. In Developing Skills in a Changing
World of Work, pages 259–282. Rainer Hampp Verlag.
Reimers, N. and Gurevych, I. (2019). Sentence-bert: Sen-
tence embeddings using siamese bert-networks. arXiv
preprint arXiv:1908.10084.
Squicciarini, M. and Nachtigall, H. (2021). Demand for ai
skills in jobs: Evidence from online job postings.
Vermeulen, W. and Amaros, F. G. (2024). How well do on-
line job postings match national sources in european
countries?: Benchmarking lightcast data against sta-
tistical and labour agency sources across regions, sec-
tors and occupation.
Vrolijk, J., Mol, S. T., Weber, C., Tavakoli, M., Kismih
´
ok,
G., and Pelucchi, M. (2022). Ontojob: Automated on-
tology learning from labor market data. In 2022 IEEE
16th International Conference on Semantic Comput-
ing (ICSC), pages 195–200. IEEE.
Weichselbraun, A., S
¨
usstrunk, N., Waldvogel, R., Glatzl,
A., Bras¸oveanu, A. M., and Scharl, A. (2024). An-
ticipating job market demands—a deep learning ap-
proach to determining the future readiness of profes-
sional skills. Future Internet, 16(5):144.
Whelan, A., M. S. S. E. and Redmond, P. (2024). Skill re-
quirements for emerging technologies in ireland. ESRI
Research Series 191.
KDIR 2024 - 16th International Conference on Knowledge Discovery and Information Retrieval
432