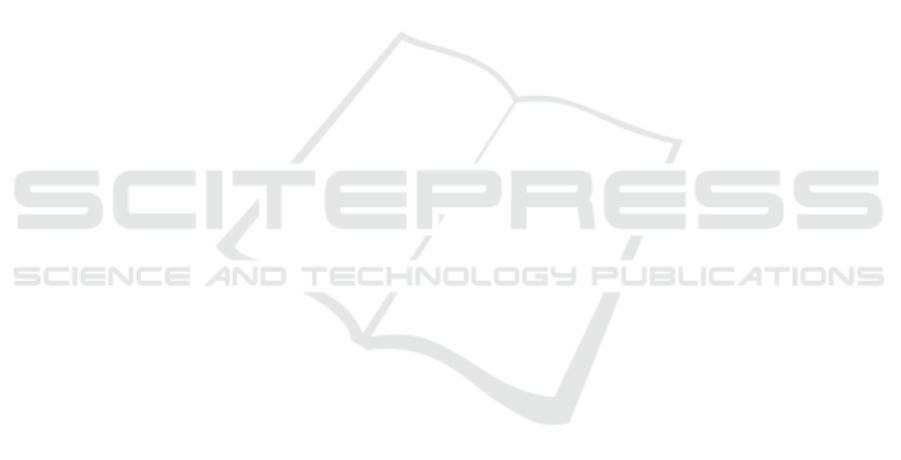
REFERENCES
Azzali, I., Cilia, N. D., De Stefano, C., Fontanella, F., Gi-
acobini, M., and Vanneschi, L. (2024). Automatic
feature extraction with vectorial genetic programming
for Alzheimer’s disease prediction through handwrit-
ing analysis. Swarm and Evolutionary Computation,
87:101571.
Bastani, O., Kim, C., and Bastani, H. (2017). Interpret-
ing blackbox models via model extraction. ArXiv,
abs/1705.08504.
Bazarbekov, I., Razaque, A., Ipalakova, M., Yoo, J., As-
sipova, Z., and Almisreb, A. (2024). A review of arti-
ficial intelligence methods for alzheimer’s disease di-
agnosis: Insights from neuroimaging to sensor data
analysis. Biomedical Signal Processing and Control,
92:106023.
Breiman, L. (2001). Random forests. Machine Learning,
45(1):5–32.
Cappiello, G. and Caruso, F. (2024). Quantum ai for
alzheimer’s disease early screening.
Cilia, N. D., De Gregorio, G., De Stefano, C., Fontanella,
F., Marcelli, A., and Parziale, A. (2022). Diag-
nosing Alzheimer’s disease from on-line handwrit-
ing: A novel dataset and performance benchmark-
ing. Engineering Applications of Artificial Intelli-
gence, 111:104822.
Cilia, N. D., De Stefano, C., Fontanella, F., and Siniscalchi,
S. M. (2024). How word semantics and phonology
affect handwriting of alzheimer’s patients: A machine
learning based analysis. Computers in Biology and
Medicine, 169:107891.
Cover, T. and Thomas, J. (2006). Elements of information
theory. John Wiley & Sons, second edition.
David W. Hosmer Jr., Rodney X. Sturdivant, S. L. (2013).
Applied Logistic Regression. 3rd edition.
Erdogmus, P. and Kabakus, A. T. (2023). The promise of
convolutional neural networks for the early diagnosis
of the alzheimer’s disease. Engineering Applications
of Artificial Intelligence, 123:106254.
Ferreira, A. and Figueiredo, M. (2023). Leveraging explain-
ability with k-fold feature selection. In 12th Inter-
national Conference on Pattern Recognition Applica-
tions and Methods (ICPRAM), pages 458–465.
Fisher, R. (1936). The use of multiple measurements in
taxonomic problems. Annals of Eugenics, 7:179–188.
Kim, B., Wattenberg, M., Gilmer, J., Cai, C., Wexler, J.,
Vi
´
egas, F., and Sayres, R. (2018). Interpretability
beyond feature attribution: Quantitative testing with
concept activation vectors (tcav). In Dy, J. G. and
Krause, A., editors, ICML, volume 80 of Proceedings
of Machine Learning Research, pages 2673–2682.
PMLR.
Koenig, L. N., Day, G. S., Salter, A., Keefe, S., Marple,
L. M., Long, J., LaMontagne, P., Massoumzadeh, P.,
Snider, B. J., Kanthamneni, M., Raji, C. A., Ghoshal,
N., Gordon, B. A., Miller-Thomas, M., Morris, J. C.,
Shimony, J. S., and Benzinger, T. L. (2020). Select
atrophied regions in Alzheimer disease (sara): An im-
proved volumetric model for identifying Alzheimer
disease dementia. NeuroImage: Clinical, 26:102248.
Kumar, S. and Shastri, S. (2022). Alzheimer MRI prepro-
cessed dataset.
Lakkaraju, H. and Bastani, O. (2020). How do I fool you?
manipulating user trust via misleading black box ex-
planations. Proceedings of the AAAI/ACM Conference
on AI, Ethics, and Society, pages 79–85.
Lou, Y., Caruana, R., Gehrke, J., and Hooker, G. (2013a).
Accurate intelligible models with pairwise interac-
tions. In Dhillon, I. S., Koren, Y., Ghani, R., Sen-
ator, T. E., Bradley, P., Parekh, R., He, J., Gross-
man, R. L., and Uthurusamy, R., editors, The 19th
ACM SIGKDD International Conference on Knowl-
edge Discovery and Data Mining, KDD, Chicago, IL,
USA, pages 623–631. ACM.
Lou, Y., Caruana, R., Gehrke, J., and Hooker, G. (2013b).
Accurate intelligible models with pairwise interac-
tions. In Proceedings of the 19th ACM SIGKDD In-
ternational Conference on Knowledge Discovery and
Data Mining, KDD ’13, page 623–631, New York,
NY, USA. Association for Computing Machinery.
Mitra, U. and Rehman, S. U. (2024). Ml-powered handwrit-
ing analysis for early detection of alzheimer’s disease.
IEEE Access, 12:69031–69050.
Mothilal, R., Sharma, A., and Tan, C. (2020). Explain-
ing machine learning classifiers through diverse coun-
terfactual explanations. In Proceedings of the 2020
Conference on Fairness, Accountability, and Trans-
parency, pages 607–617. ACM.
Rainio, O., Teuho, J., and Kl
´
en, R. (2024). Evaluation met-
rics and statistical tests for machine learning. Scien-
tific Reports, 14(1):6086.
Ribeiro, M. T., Singh, S., and Guestrin, C. (2016a). ”why
should i trust you?”: Explaining the predictions of any
classifier. In Proceedings of the 22nd ACM SIGKDD
International Conference on Knowledge Discovery
and Data Mining, KDD ’16, page 1135–1144, New
York, NY, USA. Association for Computing Machin-
ery.
Ribeiro, M. T., Singh, S., and Guestrin, C. (2016b). Why
should I trust you? explaining the predictions of any
classifier. In HLT-NAACL Demos, pages 97–101. The
Association for Computational Linguistics.
Scheda, R. and Diciotti, S. (2022). Explanations of machine
learning models in repeated nested cross-validation:
An application in age prediction using brain complex-
ity features. Applied Sciences, 12(13).
Szepannek, G. and L
¨
ubke, K. (2022). Explaining artificial
intelligence with care. KI - K
¨
unstliche Intelligenz.
Vapnik, V. (1999). The nature of statistical learning theory.
Springer-Verlag.
Yu, L. and Liu, H. (2003). Feature selection for high-
dimensional data: a fast correlation-based filter solu-
tion. In Proceedings of the International Conference
on Machine Learning (ICML), pages 856–863.
Yu, L. and Liu, H. (2004). Efficient feature selection via
analysis of relevance and redundancy. Journal of Ma-
chine Learning Research (JMLR), 5:1205–1224.
Prediction of Alzheimer Disease on the DARWIN Dataset with Dimensionality Reduction and Explainability Techniques
49