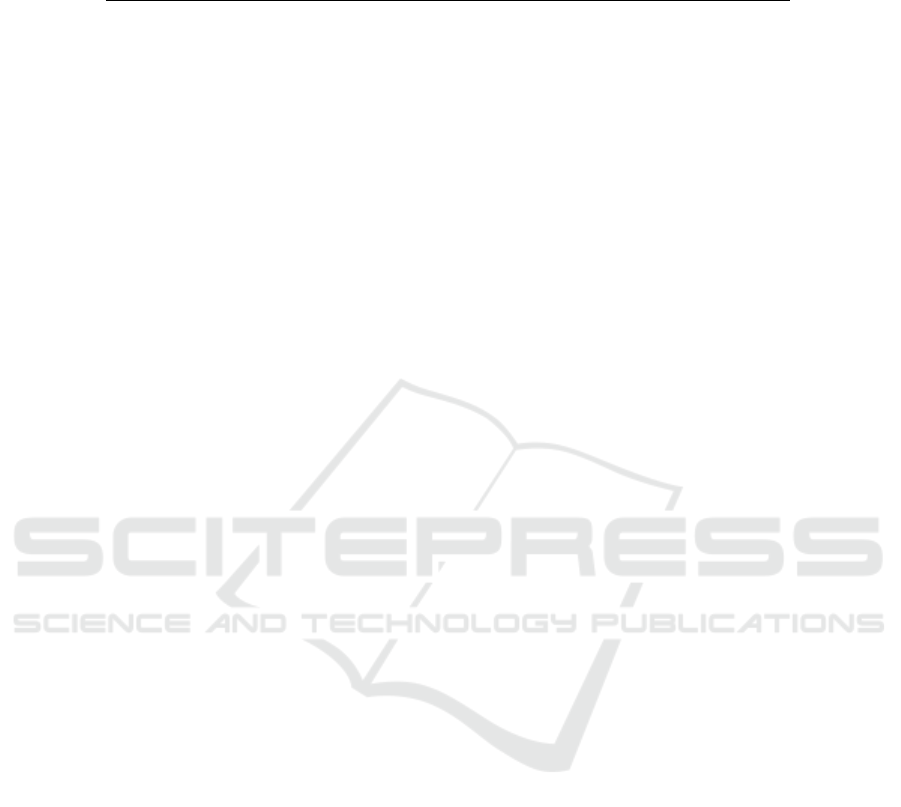
Table 4: Average absolute error, ∆, of valid estimated parameters. Medium’s EM wave propagation velocity, v, and object’s
position, x, depth, y, and radius, r. Calculated considering only first-hyperbola, 1st, and all hyperbolas from an object, group.
∆x
1st
(cm) ∆x
group
(cm) ∆y
1st
(cm) ∆y
group
(cm) ∆r
1st
(cm) ∆r
group
(cm)
2.7 2.9 2.3 1.4 0.4 0.6
The developed system works as expected when
hyperbola segmentation provided well-fitting masks,
such that the parameters were estimated from fitting
points which correctly described the hyperbola.
ACKNOWLEDGEMENTS
This work is co-financed by Component 5 - Capital-
ization and Business Innovation, integrated in the Re-
silience Dimension of the Recovery and Resilience
Plan within the scope of the Recovery and Resilience
Mechanism (MRR) of the European Union (EU),
framed in the Next Generation EU, for the period
2021 - 2026, within project AgendaTransform, with
reference 34.
REFERENCES
Bai, X., Yang, Y., Wei, S., Chen, G., Li, H., Li, Y., Tian, H.,
Zhang, T., and Cui, H. (2023). A comprehensive re-
view of conventional and deep learning approaches for
ground-penetrating radar detection of raw data. Ap-
plied Sciences, 13(13):7992.
Bestagini, P., Lombardi, F., Lualdi, M., Picetti, F., and
Tubaro, S. (2020). Landmine detection using au-
toencoders on multipolarization GPR volumetric data.
IEEE Transactions on Geoscience and Remote Sens-
ing, 59(1):182–195.
Chang, C. W., Lin, C. H., and Lien, H. S. (2009). Measure-
ment radius of reinforcing steel bar in concrete using
digital image gpr. Construction and Building Materi-
als, 23(2):1057–1063.
Chen, G., Fu, L., Chen, K., Boateng, C. D., and Ge, S.
(2019). Adaptive ground clutter reduction in ground-
penetrating radar data based on principal component
analysis. IEEE Transactions on Geoscience and Re-
mote Sensing, 57(6):3271–3282.
Chen, H. and Cohn, A. (2010). Probabilistic robust hyper-
bola mixture model for interpreting ground penetrat-
ing radar data. Proceedings of the International Joint
Conference on Neural Networks, pages 1–8.
Daniels, D. J. (2004). Ground Penetrating Radar, 2nd
Edition. Institution of Electrical Engineers, London,
United Kingdom.
Giannakis, I., Zhou, F., Warren, C., and Giannopoulos, A.
(2022). On the limitations of hyperbola fitting for esti-
mating the radius of cylindrical targets in nondestruc-
tive testing and utility detection. IEEE Geoscience
and Remote Sensing Letters, 19:1–5.
Hart, W. E., Watson, J.-P., and Woodruff, D. L. (2011). Py-
omo: modeling and solving mathematical programs
in python. Mathematical Programming Computation,
3(3):219–260.
Jol, H. M. (2009). Ground Penetrating Radar Theory and
Applications. Elsevier Science, Amsterdam, Nether-
lands.
Mertens, L., Persico, R., Matera, L., and Lambot, S. (2015).
Automated detection of reflection hyperbolas in com-
plex gpr images with no a priori knowledge on the
medium. IEEE Transactions on Geoscience and Re-
mote Sensing, 54(1):580–596.
Ni, Z.-K., Shi, C., Pan, J., Zheng, Z., Ye, S., and Fang,
G. (2022). Declutter-gan: Gpr b-scan data clutter re-
moval using conditional generative adversarial nets.
IEEE Geoscience and Remote Sensing Letters, 19:1–
5.
Persico, R. (2014). Introduction to Ground Penetrating
Radar: Inverse Scattering and Data Processing. John
Wiley and Sons, Inc., Hoboken, New Jersey.
Shorten, C. and Khoshgoftaar, T. M. (2019). A survey on
image data augmentation for deep learning. Journal
of Big Data, 6:1–48.
Utsi, E. C. (2017). Ground Penetrating Radar: Theory and
Practice. Butterworth-Heinemann, Oxford, United
Kingdom.
W
¨
achter, A. and Biegler, L. T. (2006). On the imple-
mentation of a primal-dual interior point filter line
search algorithm for large-scale nonlinear program-
ming. Mathematical Programming, 106(1):25–57.
Zheng, X., Fang, S., Chen, H., Peng, L., and Ye, Z. (2023).
Internal detection of ground-penetrating radar images
using yolox-s with modified backbone. Electronics,
12(16):3520.
Zhou, X., Chen, H., and Li, J. (2018). An automatic gpr b-
scan image interpreting model. IEEE Transactions on
Geoscience and Remote Sensing, 56(6):3398–3412.
Subsurface Metallic Object Detection Using GPR Data and YOLOv8 Based Image Segmentation
699