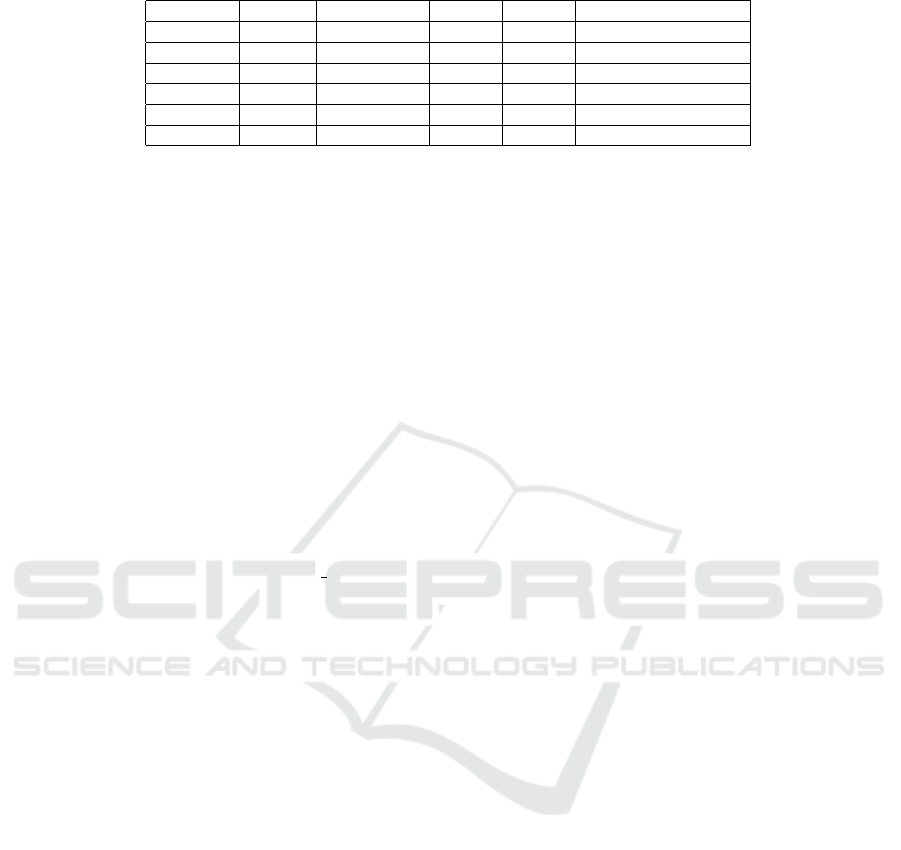
Table 3: Percentage error of each one of the metrics used to evaluate the spray quality of a water-sensitive paper.
Method Dataset No Droplets VMD RSF Coverage Percentage
YOLOv8 RD 0.5561 0.2233 2.7338 0.6095
YOLOv8 SD 0.2621 0.1423 0.2729 0.4593
CCV RD 0.4189 0.1952 1.9945 0.1533
CCV SD 0.3144 2.5103 0.5108 0.0842
DropLeaf RD 0.6096 0.7362 0.0916 0.5223
DropLeaf SD 0.9667 0.3738 8.5897 0.2639
findings emphasize the potential of using synthetic
datasets to train machine learning models to enhance
accuracy and efficiency. Future research could fo-
cus on refining the process of generating synthetic
datasets of WSP and developing more advanced
machine-learning models to further advance its anal-
ysis.
ACKNOWLEDGEMENTS
This work is co-financed by Component 5 - Capital-
ization and Business Innovation, integrated in the Re-
silience Dimension of the Recovery and Resilience
Plan within the scope of the Recovery and Resilience
Mechanism (MRR) of the European Union (EU),
framed in the Next Generation EU, for the period
2021 - 2026, within project Vine&Wine PT, with ref-
erence 67.
REFERENCES
Cerruto, E., Manetto, G., Longo, D., Failla, S., and Papa, R.
(2019). A model to estimate the spray deposit by sim-
ulated water sensitive papers. Crop Protection, 124.
D
´
echelette, A., Babinsky, E., and Sojka, P. (2011). Hand-
book of atomization and sprays.
Hoffmann, W. and Hewitt, A. (2005). Comparison of three
imaging systems for water-sensitive papers. Applied
Engineering in Agriculture, 21:961–964. USDA-
ARS.
Lefebvre, A. and McDonell, V. (2017). Atomization and
sprays. CRC Press.
Lipi
´
nski, A. and Lipi
´
nski, S. (2020). Binarizing water sen-
sitive papers – how to assess the coverage area prop-
erly? Crop Protection, 127.
Liu, J., Yu, S., Liu, X., Wang, Q., Cui, H., Zhu, Y., and
Yuan, J. (2024). A novel optical shadow edge imaging
method based fast in-situ measuring portable device
for droplet deposition. Computers and Electronics in
Agriculture, 217:108632.
Machado, B. B., Spadon, G., Arruda, M. S., Goncalves,
W. N., Carvalho, A. C., and Rodrigues-Jr, J. F. (2018).
A smartphone application to measure the quality of
pest control spraying machines via image analysis.
Proceedings of the ACM Symposium on Applied Com-
puting.
Marc¸al, A. and Cunha, M. (2008). Image processing of arti-
ficial targets for automatic evaluation of spray quality.
Transactions of the ASABE, 51:811–821.
Mugele, R. A. and Evans, H. D. (1951). Droplet size distri-
bution in sprays. Industrial & Engineering Chemistry,
43:1317–1324.
Nansen, C., Ferguson, J., Moore, J., Groves, L., Emery, R.,
Garel, N., and Hewitt, A. (2015). Optimizing pes-
ticide spray coverage using a novel web and smart-
phone tool, snapcard. Agronomy for Sustainable De-
velopment, 35:1075–1085. SnapCard.
Nansen, C., Villar, G., Recalde, A., Alvarado, E., and Chen-
napragada, K. (2021). Phone app to perform quality
control of pesticide spray applications in field crops.
Agriculture (Switzerland), 11. SmartSpray.
Privitera, S., Manetto, G., Pascuzzi, S., Pessina, D., and
Cerruto, E. (2023). Drop size measurement tech-
niques for agricultural sprays:a state-of-the-art review.
Agronomy.
Schick, R. J. (2008). Spray technology reference guide:
Understanding drop size. Spray Systems Co.
Wen, T., Tong, B., Liu, Y., Pan, T., Du, Y., Chen, Y., and
Zhang, S. (2022). Review of research on the instance
segmentation of cell images. Computer Methods and
Programs in Biomedicine, 227:107211.
Xun, L. and Gil, E. (2024). A novel methodology for water-
sensitive papers analysis focusing on the segmentation
of overlapping droplets to better characterize deposi-
tion pattern. Crop Protection, 176.
Zhu, H., Salyani, M., and Fox, R. (2011). A portable
scanning system for evaluation of spray deposit dis-
tribution. Computers and Electronics in Agriculture,
76:38–43. DepositScan.
¨
Omer Barıs¸
¨
Ozl
¨
uoymak and Bolat, A. (2020). Develop-
ment and assessment of a novel imaging software for
optimizing the spray parameters on water-sensitive
papers. Computers and Electronics in Agriculture,
168:105104.
Spray Quality Assessment on Water-Sensitive Paper Comparing AI and Classical Computer Vision Methods
307