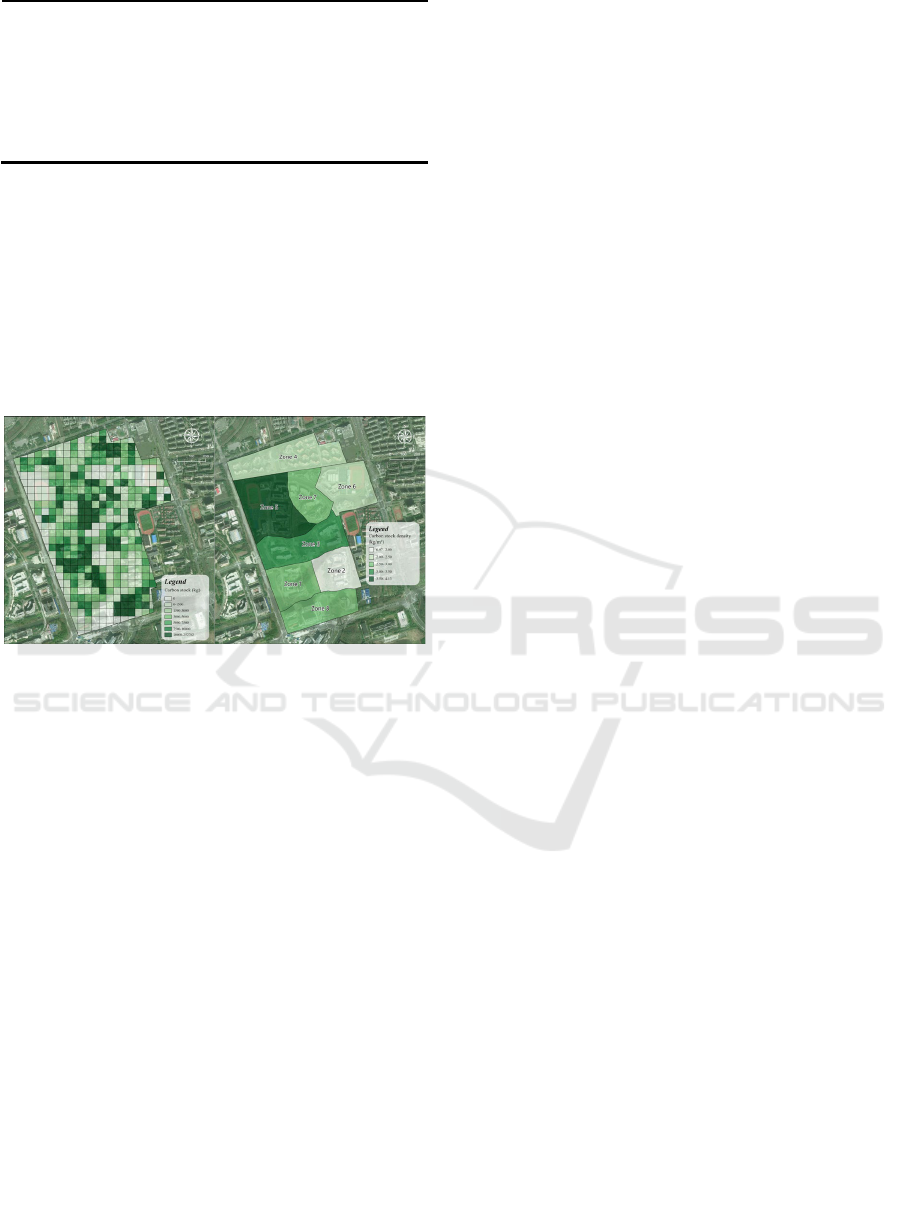
6 239094.774 239085.395 9.379
7 259546.127 259537.141 8.986
8 278720.130 278712.361 7.769
Total 2677442.666 2677353.950 88.719
To analyze the spatial distribution of carbon stock
within the campus, the study drew a carbon stock map
under the 50m×50m grid (Figure 8(a)) and the map of
carbon stock density in different zones (Figure 8(b)).
There are significant differences in the size and
density of carbon storage across various campus areas.
Generally, the carbon stock is smaller in areas with
dense buildings and open areas (e.g. playgrounds),
and the larger carbon stock is mainly in areas where
trees are concentrated (e.g. both sides of the main
road and the green spaces).
Figure 8: (a) Carbon stock map under 50m×50m
Grid, (b) Carbon stock density in different zones
Picture credit: Original
4 CONCLUSION
This study establishes a comprehensive and reliable
technical framework for the fine estimation of urban
vegetation carbon stock by utilizing multi-source
LiDAR data. This framework includes following
steps: This framework includes: LiDAR data
preprocessing, shrub extraction and volume
calculation, tree segmentation, and carbon stock
calculation. Specifically, after data preprocessing, the
study first uses a random forest model to extract
shrubs from the point cloud and employs a grid
method to calculate their volume. Subsequently, by
comparing four different tree segmentation
algorithms across six sample plots, the layer stacking
algorithm, which demonstrates superior accuracy and
stability in both coniferous and broad-leaved forests,
is selected for tree segmentation in the study region.
After obtaining the morphological parameters of
individual trees, the study builds biomass models
suitable for the vegetation in the study area using the
average biomass method, and calculates the final
carbon storage to be 2,677,442.666 kg.
The technical framework proposed in this study is
universally applicable to the accurate estimation of
urban vegetation carbon stock. Additionally, due to
the rich semantic information contained in LiDAR
data, the research data (such as the morphological
parameters of individual trees) can be widely applied
to other forestry applications. This provides technical
and data support for helping achieve dual carbon
goals and formulate related policies.
However, this study also has certain limitations.
The formulae used to calculate carbon stocks for
different species of trees may vary, and the study's
lack of species differentiation may have contributed
to some degree of error in the carbon stock conclusion.
Future research could consider incorporating street
view images or high-resolution remote sensing
images for tree species identification, thus building
biomass models for different species and further
refining the precision of carbon stock calculations.
REFERENCES
Ayrey, E., Fraver, S., Kershaw Jr, J. A., Kenefic, L. S.,
Hayes, D., Weiskittel, A. R., & Roth, B. E. (2017).
Layer stacking: A novel algorithm for individual forest
tree segmentation from LiDAR point clouds. Canadian
Journal of Remote Sensing, 43(1), 16-27.
2
Chen, Q. , Baldocchi, D. , Gong, P. , & Kelly, M. (2006).
Isolating individual trees in a savanna woodland using
small footprint lidar data. Photogrammetric
Engineering and Remote Sensing, 72(8), 923-932.
Creutzig, F., Agoston, P., Minx, J. C., Canadell, J. G.,
Andrew, R. M., Le Quéré, C., Peters, G. P., Sharifi, A.,
Yamagata, Y., & Dhakal, S. (2016). Urban
infrastructure choices structure climate solutions.
Nature Climate Change, 6(12), 1054-1056.
Fang, Y. H. (2013). Carbon sequestration and its
relationship with leaf functional traits: a study of 25
greening shrubs in Shanghai. Master’s thesis, East
China Normal University.
Kaartinen, H., Hyyppä, J., Yu, X. W., Vastaranta, M.,
Hyyppä, H., Kukko, A., Holopainen, M., Heipke, C.,
Hirschmugl, M., Morsdorf, F., Næsset, E., Pitkänen, J.,
Popescu, S., Solberg, S., Wolf, B. M., & Wu, J. C.
(2012). An International Comparison of Individual Tree
Detection and Extraction Using Airborne Laser
Scanning. Remote Sensing, 4(4), 950-974.
Khosravipour, A., Skidmore, A. K., Isenburg, M., Wang, T.,
& Hussin, Y. A. (2014). Generating pit-free canopy
height models from airborne lidar. Photogrammetric
Engineering and Remote Sensing, 80(9), 863-872.
1
Koch, B., Heyder, U., & Weinacker, H. (2006). Detection
of individual tree crowns in airborne lidar data.
Precise Estimation of Urban Vegetation Carbon Stock Using Multi-Source LiDAR: A Case Study of East China Normal University
207