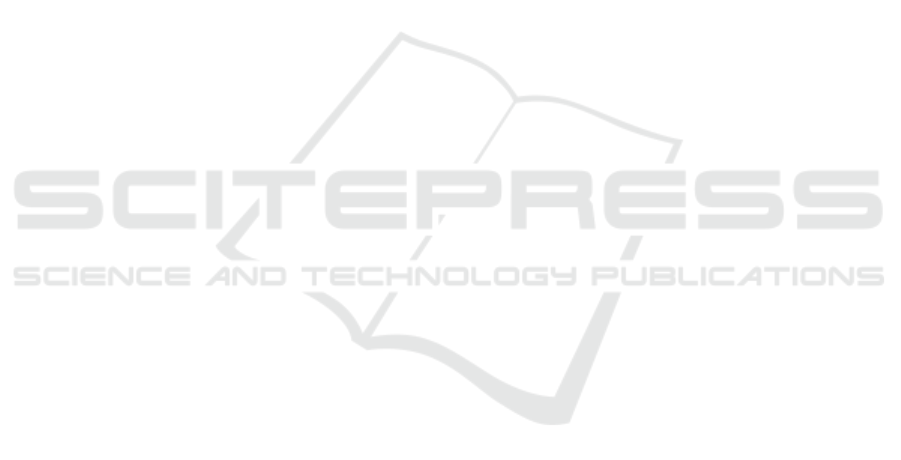
Technologies for Wearable Sleep Staging. Sensors,
21(5):1562.
In-Ho Choi and Yong-Guk Kim (2014). Head pose and gaze
direction tracking for detecting a drowsy driver. In
2014 International Conference on Big Data and Smart
Computing (BIGCOMP), pages 241–244. IEEE.
Kang, D.-H., Jeon, J.-K., and Lee, J.-H. (2015). Effects
of low-frequency electrical stimulation on cumulative
fatigue and muscle tone of the erector spinae. Journal
of Physical Therapy Science, 27(1):105–108.
Kaur, K. and Singh, P. (2023). Trends in speech emo-
tion recognition: a comprehensive survey. Multimedia
Tools and Applications.
Khushaba, R. N., Kodagoda, S., Lal, S., and Dissanayake,
G. (2011). Driver Drowsiness Classification Using
Fuzzy Wavelet-Packet-Based Feature-Extraction Al-
gorithm. IEEE Transactions on Biomedical Engineer-
ing, 58(1):121–131.
Kimble, M., Boxwala, M., Bean, W., Maletsky, K., Halper,
J., Spollen, K., and Fleming, K. (2014). The impact
of hypervigilance: Evidence for a forward feedback
loop. Journal of Anxiety Disorders, 28(2):241–245.
Kumar, J. S. and Bhuvaneswari, P. (2012). Analysis of
Electroencephalography (EEG) Signals and Its Cate-
gorization–A Study. Procedia Engineering, 38:2525–
2536.
Kunasegaran, K., Ismail, A. M. H., Ramasamy, S., Gnanou,
J. V., Caszo, B. A., and Chen, P. L. (2023). Un-
derstanding mental fatigue and its detection: a com-
parative analysis of assessments and tools. PeerJ,
11:e15744.
Lohani, M., Payne, B. R., and Strayer, D. L. (2019). A
Review of Psychophysiological Measures to Assess
Cognitive States in Real-World Driving. Frontiers in
Human Neuroscience, 13.
M. R. Rosekind, K. B. Gregory, E. L. Co, D. L. Miller and
Dinges, D. F. (2000). Crew Factors in Flight Opera-
tions XII: A Survey of Sleep Quantity and Quality in
On-Board Crew Rest Facilities.
Martins, A. P. G. (2016). A review of important cognitive
concepts in aviation. Aviation, 20(2):65–84.
May, J. F. and Baldwin, C. L. (2009). Driver fatigue:
The importance of identifying causal factors of fatigue
when considering detection and countermeasure tech-
nologies. Transportation Research Part F: Traffic Psy-
chology and Behaviour, 12(3):218–224.
Nemcova, A., Svozilova, V., Bucsuhazy, K., Smisek, R.,
Mezl, M., Hesko, B., Belak, M., Bilik, M., Maxera,
P., Seitl, M., Dominik, T., Semela, M., Sucha, M.,
and Kolar, R. (2021). Multimodal Features for De-
tection of Driver Stress and Fatigue: Review. IEEE
Transactions on Intelligent Transportation Systems,
22(6):3214–3233.
Ngxande, M., Tapamo, J.-R., and Burke, M. (2017). Driver
drowsiness detection using behavioral measures and
machine learning techniques: A review of state-of-
art techniques. In 2017 Pattern Recognition Associ-
ation of South Africa and Robotics and Mechatronics
(PRASA-RobMech), pages 156–161. IEEE.
Ohrui, N., Kanazawa, F., Takeuchi, Y., Otsuka, Y., Tarui,
H., and Miyamoto, Y. (2008). Urinary Catecholamine
Responses in F-15 Pilots: Evaluation of the Stress In-
duced by Long-Distance Flights. Military Medicine,
173(6):594–598.
Rafid, A.-U.-I., Raha Niloy, A., Chowdhury, A. I., and
Sharmin, N. (2020). A Brief Review on Different
Driver’s Drowsiness Detection Techniques. Interna-
tional Journal of Image, Graphics and Signal Process-
ing, 12(3):41–50.
Sahayadhas, A., Sundaraj, K., Murugappan, M., and Pala-
niappan, R. (2015). Physiological signal based detec-
tion of driver hypovigilance using higher order spec-
tra. Expert Systems with Applications, 42(22):8669–
8677.
Shaw, D. M. and Harrell, J. W. (2023). Integrating phys-
iological monitoring systems in military aviation : a
brief narrative review of its importance , opportunities
, and risks ABSTRACT. Ergonomics, 0(0):1–13.
Shetty, J., Lawson, C. P., and Shahneh, A. Z. (2015). Sim-
ulation for temperature control of a military aircraft
cockpit to avoid pilot’s thermal stress. CEAS Aero-
nautical Journal, 6(2):319–333.
Smallwood, J. and Schooler, J. W. (2015). The Science of
Mind Wandering: Empirically Navigating the Stream
of Consciousness. Annual Review of Psychology,
66(Volume 66, 2015):487–518.
Stancin, I., Cifrek, M., and Jovic, A. (2021). A Review of
EEG Signal Features and Their Application in Driver
Drowsiness Detection Systems. Sensors, 21(11):3786.
Sullivan-Kwantes, W., Cramer, M., Bouak, F., and Good-
man, L. (2021). Environmental Stress in Military Set-
tings. In Handbook of Military Sciences, pages 1–27.
Springer International Publishing, Cham.
Trujillo, A. (1998). Pilot mental workload with predictive
system status information. In Proceedings Fourth An-
nual Symposium on Human Interaction with Complex
Systems, pages 73–80.
Velasco, J. M., Botella-Serrano, M., S
´
anchez-S
´
anchez, A.,
Aramendi, A., Mart
´
ınez, R., Maqueda, E., Garnica,
O., Contador, S., Lanchares, J., and Hidalgo, J. I.
(2022). Evaluating the Influence of Mood and Stress
on Glycemic Variability in People with T1DM Using
Glucose Monitoring Sensors and Pools. Diabetology,
3(2):268–275.
Zawilinski, L. (2020). Hypervigilance, pages 2101–2103.
Springer International Publishing, Cham.
Zhang, T., Yang, J., Liang, N., Pitts, B. J., Prakah-Asante,
K., Curry, R., Duerstock, B., Wachs, J. P., and Yu,
D. (2020). Physiological Measurements of Situation
Awareness: A Systematic Review. Human Factors,
65(5):737–758.
Zou, H., Zhou, H., Yan, R., Yao, Z., and Lu, Q. (2022).
Chronotype, circadian rhythm, and psychiatric dis-
orders: Recent evidence and potential mechanisms.
Frontiers in Neuroscience, 16.
Comprehensive Study on Fighter Pilot Attention and Vigilance Monitoring
125