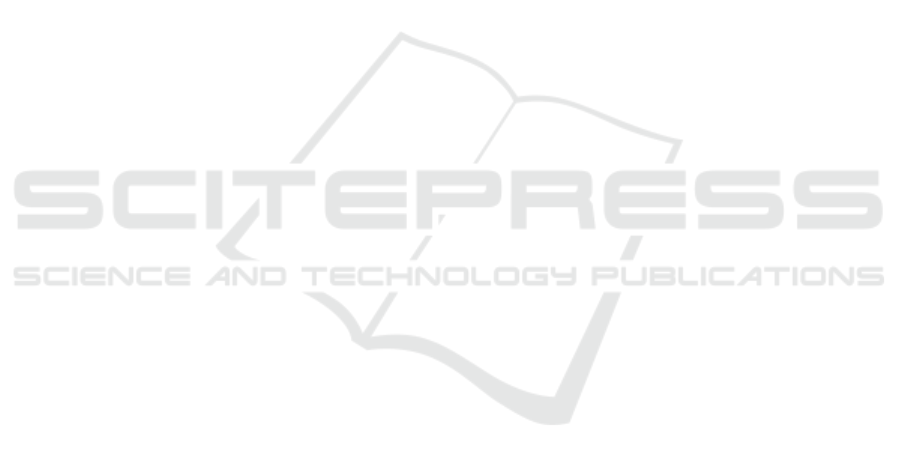
suggesting that a player’s overall performance is posi-
tively influenced by the team’s victory and significant
playing time, showcasing their consistent contribution
to the team’s success.
These trends are generally expected. When we
look at plus-minus, we focus on players who fall
into the fourth category for each win-share category.
These players are average or above average and have
significantly contributed to their team’s success dur-
ing the season. They can be described as ”role play-
ers” or good players but are not considered ”all-star”
caliber players. These players either support high-
level talent in a roster by assisting them when they
are on the court together or by holding down the team
when their all-star players are not on the court.
When analyzing their decision trees, the number
of minutes played in the previous game becomes an
important feature in the player’s plus-minus. If the
team lost and the player’s previous game minutes
were over 25, the player’s current game performance
had a plus-minus in the first category, which is ex-
tremely low. However, if their minutes were less than
25, their current game performance had a plus-minus
in the second category, which is still low but not as
bad. The difference in plus-minus is small; however,
we can see that the excessive use of role players in
the previous game not only negatively affects their
overall performance in the current game but may also
cause their team’s loss. This suggests that role players
should be used cautiously if their previous game was
strenuous.
In the early part of the season, players in groups
1 and 2 are most affected by the gap between games,
while the impact on the rest of the players is mini-
mal. However, as we move into the later part of the
season, players in groups 1 and 2 still experience an
impact on their +/-. This impact is reduced compared
to the early part of the season. Once again, players
in the middle of the pack do not experience a major
impact, while top players see a significant impact on
their performance due to the gaps between games.
The results are shown in Figure, 5, it is worth not-
ing that the accuracy of these decision trees ranges
from 0.3 to 0.5, representing extremely low accuracy.
This could be attributed to the extensive use of equal-
frequency binning. Increasing the number of bins
from 6 might lead to more accurate results.
As the season begins, players in the lower skill
level groups, specifically bins 1 and 2, are most af-
fected by the extended breaks between games. This
can have a significant impact on their performance
and readiness. However, as the season advances, the
influence on players in these skill-level groups grad-
ually decreases. Meanwhile, players in the interme-
diate skill level range continue to encounter mini-
mal impact from the gaps between games, while the
top-tier players are notably affected by the extended
breaks, potentially impacting their momentum and
form.
Upon analyzing the impact of the timing of the
season and the duration between games on player per-
formance, the findings are as follows: Figure 6. In the
early stages of the season, underperforming players
are notably more affected by longer breaks between
games. As the season progresses, top-performing
players are increasingly impacted by the duration of
breaks between games, while mid-range players tend
to maintain consistent performance regardless of the
gap. From a strategic perspective, top players should
prioritize rest at the season’s commencement and re-
duce rest as the season advances. Moreover, due to
their versatility, role players can be utilized more fre-
quently. Lastly, players ranked at the bottom in terms
of performance would benefit from consistent game
time, particularly at the season’s onset.
5.3 Question 3: Player’s Performance
After analyzing the k-means clustering plot, we dis-
covered some fascinating results. Previous studies,
such as the one by Berri et al. (Berri et al., 2011),
have indicated that height plays a significant role in
the selection of amateur players in drafts. However,
our plot unveiled an intriguing pattern. The players
were effectively categorized into three clusters based
on height, with distinct groups for shorter, medium-
height, and taller players.
The analysis of the players’ heights in relation to
their performance yielded intriguing findings. Upon
closer examination, it was noted that the shortest play-
ers tended to exhibit lower offensive win-shares, sug-
gesting a diminished level of performance. How-
ever, this trend was not confined to shorter players,
as taller players also displayed a similar pattern. Inter-
estingly, a performance peak was identified within the
medium-height range, followed by a decline among
the taller players. These observations point to the pos-
sibility that optimal performance may not necessarily
correlate with extreme heights, but rather lie some-
where within the middle range of heights.
Upon analyzing the height-rebounds graph, a clear
pattern emerges indicating that players with higher
overall performance also excel in rebounds, in line
with expectations. Notably, players in the average
height category (H2) exhibit the most impressive re-
bound performance within each group. Consistently,
a trend is evident wherein shorter players tend to un-
derperform compared to their average or tall counter-
icSPORTS 2024 - 12th International Conference on Sport Sciences Research and Technology Support
90