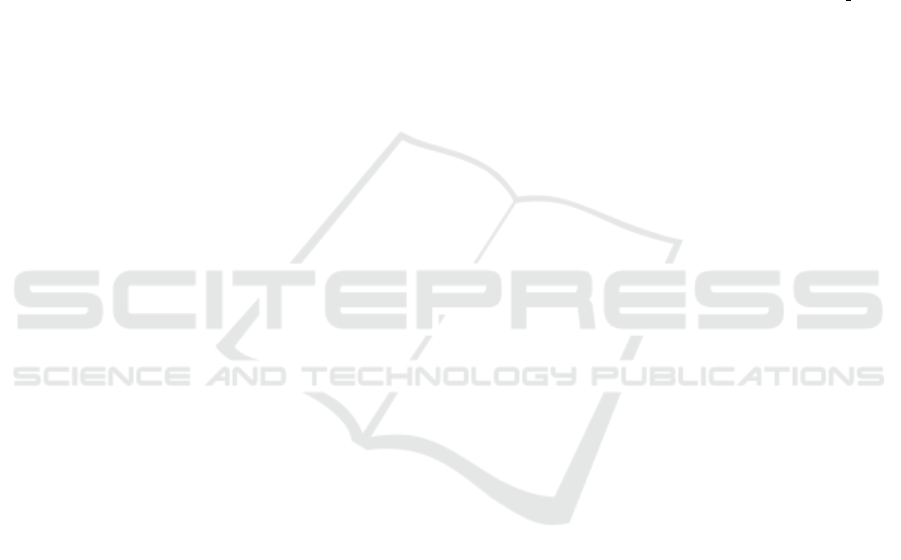
Ferragina, P. and Gulli, A. (2005). A personalized search
engine based on web-snippet hierarchical clustering.
In Special interest tracks and posters of the 14th inter-
national conference on World Wide Web, WWW ’05,
pages 801–810, New York, NY, USA. Association for
Computing Machinery.
Fishburn, P. C. (1991). Nontransitive Preferences in De-
cision Theory. Journal of Risk and Uncertainty,
4(2):113–134. Publisher: Springer.
Gauch, S., Chaffee, J., and Pretschner, A. (2003). Ontology-
based personalized search and browsing. Web Intelli.
and Agent Sys., 1(3-4):219–234.
Gendin, S. (1996). Why Preference is Not Transitive. The
Philosophical Quarterly (1950-), 46(185):482–488.
Publisher: [Oxford University Press, University of St.
Andrews, Scots Philosophical Association].
Hambarde, K. A. and Proenc¸a, H. (2023). Information Re-
trieval: Recent Advances and Beyond. IEEE Access,
11:76581–76604. Conference Name: IEEE Access.
Heinrich, B. and Klier, M. (2011). Assessing data cur-
rency—a probabilistic approach. Journal of Informa-
tion Science, 37(1):86–100. Publisher: Sage Publica-
tions Sage UK: London, England.
Jaenich, T., McDonald, G., and Ounis, I. (2024). Query Ex-
posure Prediction for Groups of Documents in Rank-
ings. In Goharian, N., Tonellotto, N., He, Y., Lipani,
A., McDonald, G., Macdonald, C., and Ounis, I., ed-
itors, Advances in Information Retrieval, pages 143–
158, Cham. Springer Nature Switzerland.
Jayarathna, S., Patra, A., and Shipman, F. (2013). Min-
ing user interest from search tasks and annotations.
In Proceedings of the 22nd ACM international con-
ference on Information & Knowledge Management,
CIKM ’13, pages 1849–1852, New York, NY, USA.
Association for Computing Machinery.
Kelly, D., Dollu, V. D., and Fu, X. (2005). The loqua-
cious user: a document-independent source of terms
for query expansion. In Proceedings of the 28th
annual international ACM SIGIR conference on Re-
search and development in information retrieval, SI-
GIR ’05, pages 457–464, New York, NY, USA. Asso-
ciation for Computing Machinery.
Khokhlov, I. and Reznik, L. (2020). What is the value of
data value in practical security applications. In 2020
IEEE Systems Security Symposium (SSS), pages 1–8.
IEEE.
Kraft, R., Maghoul, F., and Chang, C. C. (2005). Y!Q:
contextual search at the point of inspiration. In Pro-
ceedings of the 14th ACM international conference on
Information and knowledge management, CIKM ’05,
pages 816–823, New York, NY, USA. Association for
Computing Machinery.
Kunze, S. R. and Auer, S. (2013). Dataset Retrieval. In 2013
IEEE Seventh International Conference on Semantic
Computing, pages 1–8.
Laney, D. B. (2017). Infonomics: how to monetize, manage,
and measure information as an asset for competitive
advantage. Routledge.
Lavrenko, V. and Croft, W. B. (2001). Relevance based
language models. In Proceedings of the 24th annual
international ACM SIGIR conference on Research
and development in information retrieval, SIGIR ’01,
pages 120–127, New York, NY, USA. Association for
Computing Machinery.
Liu, F., Yu, C., and Meng, W. (2002). Personalized web
search by mapping user queries to categories. In Pro-
ceedings of the eleventh international conference on
Information and knowledge management, CIKM ’02,
pages 558–565, New York, NY, USA. Association for
Computing Machinery.
Liu, H. and Hoeber, O. (2011). A Luhn-Inspired Vector Re-
weighting Approach for Improving Personalized Web
Search. pages 301–305. IEEE Computer Society.
Liu, J., Liu, C., and Belkin, N. J. (2020). Personal-
ization in text information retrieval: A survey.
Journal of the Association for Information Sci-
ence and Technology, 71(3):349–369. eprint:
https://onlinelibrary.wiley.com/doi/pdf/10.1002/asi.
24234.
Liu, T.-Y. (2009). Learning to Rank for Information Re-
trieval. Found. Trends Inf. Retr., 3(3):225–331.
Lv, Y., Sun, L., Zhang, J., Nie, J.-Y., Chen, W., and Zhang,
W. (2006). An iterative implicit feedback approach
to personalized search. In Proceedings of the 21st In-
ternational Conference on Computational Linguistics
and the 44th annual meeting of the Association for
Computational Linguistics, ACL-44, pages 585–592,
USA. Association for Computational Linguistics.
Ma, X. and Zhang, X. (2019). MDV: A Multi-Factors Data
Valuation Method. In 2019 5th International Con-
ference on Big Data Computing and Communications
(BIGCOM), pages 48–53.
Maron, M. E. and Kuhns, J. L. (1960). On Relevance, Prob-
abilistic Indexing and Information Retrieval. J. ACM,
7(3):216–244.
Miutra, B. and Craswell, N. (2018). An Introduction to
Neural Information Retrieval. Found. Trends Inf. Retr.,
13(1):1–126.
Odu, G. O. (2019). Weighting methods for multi-criteria de-
cision making technique. Journal of Applied Sciences
and Environmental Management, 23(8):1449–1457.
Pitkow, J., Sch
¨
utze, H., Cass, T., Cooley, R., Turnbull, D.,
Edmonds, A., Adar, E., and Breuel, T. (2002). Per-
sonalized search: A contextual computing approach
may prove a breakthrough in personalized search effi-
ciency. Commun. ACM, 45(9):50–55.
Pretschner, A. and Gauch, S. (1999). Ontology based per-
sonalized search. In Proceedings 11th International
Conference on Tools with Artificial Intelligence, pages
391–398. ISSN: 1082-3409.
Qiu, S., Zhang, D., and Du, X. (2017). An Evaluation
Method of Data Valuation Based on Analytic Hi-
erarchy Process. In 2017 14th International Sym-
posium on Pervasive Systems, Algorithms and Net-
works & 2017 11th International Conference on Fron-
tier of Computer Science and Technology & 2017
Third International Symposium of Creative Comput-
ing (ISPAN-FCST-ISCC), pages 524–528. ISSN:
2375-527X.
Roberts, S. W. (1959). Control Chart Tests Based on Ge-
ometric Moving Averages. Technometrics, 1(3):239–
250.
Personalization of Dataset Retrieval Results Using a Data Valuation Method
133