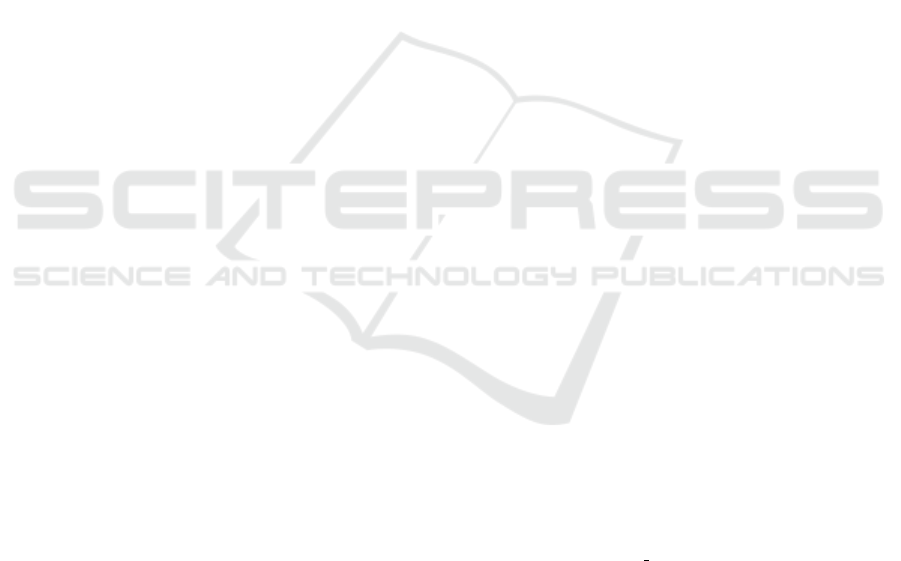
in this study. Fixing these parameters can enhance the
accuracy of the model. Third, in addition to the ongo-
ing development and refinement of a hand detection
model, consideration should be given to the integra-
tion of this model into virtual training, as previously
outlined in the main objective of this study. In other
words, the effectiveness of the model must be eval-
uated to determine whether the trainees are able to
adapt to it. One potential methodology for evaluat-
ing the efficacy of the aforementioned approach is to
devise a series of scenarios in which the trainee is re-
quired to position themselves and perform gestures in
a manner that allows for the assessment of their adap-
tation to the hand pose detection model.
8 CONCLUSIONS
A comparative analysis of two distinct methodologies
for hand pose estimation, one based on depth maps
and the other on stereo images, has yielded signifi-
cant insights into the relative strengths and limitations
of each approach. Although neither approach yielded
a near-optimal solution, both demonstrated effective-
ness in accurately capturing the spatial position of the
hand and constructing viable hand representations.
These results suggest the potential for substantial im-
provements in accuracy, robustness, and adaptability
through further refinement and optimisation of exist-
ing techniques. Consequently, continued research and
development in this area could lead to more advanced
solutions for applications such as virtual reality train-
ing and gesture-based control systems.
ACKNOWLEDGEMENTS
The authors thank Tecnologico de Monterrey for fi-
nancial support to produce this work.
REFERENCES
Alinezhad Noghre, G., Danesh Pazho, A., Katariya, V.,
and Tabkhi, H. (2023). Understanding the Challenges
and Opportunities of Pose-based Anomaly Detection.
In Proceedings of the 8th international Workshop on
Sensor-Based Activity Recognition and Artificial In-
telligence, pages 1–9, L
¨
ubeck Germany. ACM.
American Society for Surgery of the Hand (2024). Joints.
Publication Title: Body Anatomy: Upper Extremity
Joints | The Hand Society.
Buran Basha, M., Ravi Teja, S., Pavan Kumar, K., and
Anudeep, M. (2020). Hand poses detection using co-
volutional neural network. International Journal of
Scientific & Technology Research, 9(1):1887–1891.
Chatzis, T., Stergioulas, A., Konstantinidis, D., Dim-
itropoulos, K., and Daras, P. (2020). A Comprehen-
sive Study on Deep Learning-Based 3D Hand Pose
Estimation Methods. Applied Sciences, 10(19):6850.
Gomez-Donoso, F., Orts-Escolano, S., and Cazorla, M.
(2019). Large-scale multiview 3D hand pose dataset.
Image and Vision Computing, 81:25–33.
google (2024). GitHub - google/mediapipe: Cross-
platform, customizable ML solutions for live and
streaming media. Publication Title: GitHub.
Gu, F., Fan, J., Cai, C., Wang, Z., Liu, X., Yang, J., and Zhu,
Q. (2022). Automatic detection of abnormal hand ges-
tures in patients with radial, ulnar, or median nerve
injury using hand pose estimation. Frontiers in Neu-
rology, 13:1052505.
Guan, X., Shen, H., Nyatega, C. O., and Li, Q. (2023).
Repeated Cross-Scale Structure-Induced Feature Fu-
sion Network for 2D Hand Pose Estimation. Entropy,
25(5):724.
Haji Mohd, M. N., Mohd Asaari, M. S., Lay Ping, O., and
Rosdi, B. A. (2023). Vision-Based Hand Detection
and Tracking Using Fusion of Kernelized Correlation
Filter and Single-Shot Detection. Applied Sciences,
13(13):7433.
He, W., Xie, Z., Li, Y., Wang, X., and Cai, W. (2019).
Synthesizing Depth Hand Images with GANs and
Style Transfer for Hand Pose Estimation. Sensors,
19(13):2919.
Liu, S., Yuan, X., Feng, W., Ren, A., Hu, Z., Ming, Z., Za-
hid, A., Abbasi, Q., and Wang, S. (2023). A Novel
Heteromorphic Ensemble Algorithm for Hand Pose
Recognition. Symmetry, 15(3):769.
Malik, J., Elhayek, A., and Stricker, D. (2019). WHSP-Net:
A Weakly-Supervised Approach for 3D Hand Shape
and Pose Recovery from a Single Depth Image. Sen-
sors, 19(17):3784.
Sharma, S. and Huang, S. (2021). An end-to-end frame-
work for unconstrained monocular 3D hand pose esti-
mation. Pattern Recognition, 115:107892.
Wu, Y., Ma, S., Zhang, D., and Sun, J. (2020). 3D Capsule
Hand Pose Estimation Network Based on Structural
Relationship Information. Symmetry, 12(10):1636.
Zhang, J., Jiao, J., Chen, M., Qu, L., Xu, X., and Yang, Q.
(2016). 3D Hand Pose Tracking and Estimation Using
Stereo Matching.
eprint: 1610.07214.
Zhang, Q., Lin, Y., Lin, Y., and Rusinkiewicz, S. (2023).
Hand Pose Estimation with Mems-Ultrasonic Sensors.
In SIGGRAPH Asia 2023 Conference Papers, pages
1–11, Sydney NSW Australia. ACM.
A Comparative Analysis of Methods for Hand Pose Detection in 3D Environments
313