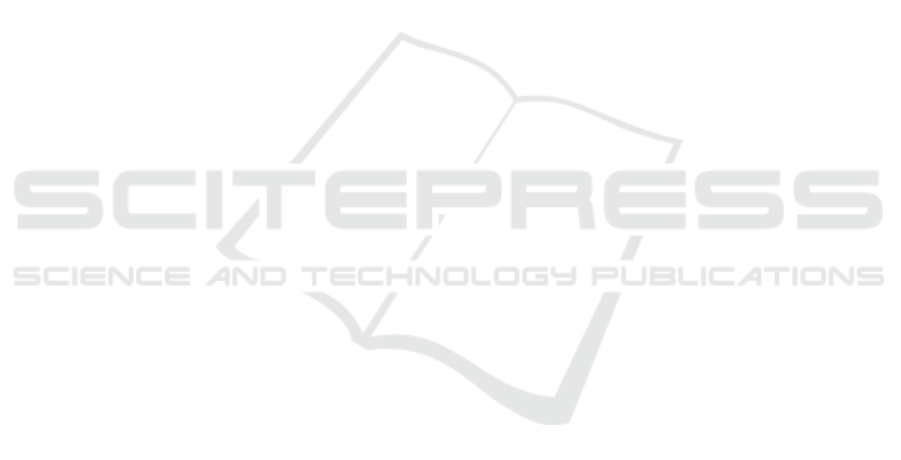
Joublin, F., Ceravola, A., Smirnov, P., Ocker, F.,
Deigmoeller, J., Belardinelli, A., Wang, C., Hasler, S.,
Tanneberg, D., and Gienger, M. (2023). Copal: Cor-
rective planning of robot actions with large language
models.
Ling, W., Yogatama, D., Dyer, C., and Blunsom, P. (2017).
Program induction by rationale generation: Learning
to solve and explain algebraic word problems. In Pro-
ceedings of the 55th Annual Meeting of the Associa-
tion for Computational Linguistics (Volume 1: Long
Papers), pages 158–167, Vancouver, Canada. Associ-
ation for Computational Linguistics.
Moens, M. and Steedman, M. (1988). Temporal ontology
and temporal reference. In International Conference
on Computational Logic.
OpenAI (2023). Gpt-4 technical report.
Parmar, M., Patel, N., Varshney, N., Nakamura, M., Luo,
M., Mashetty, S., Mitra, A., and Baral, C. (2024).
Logicbench: Towards systematic evaluation of logical
reasoning ability of large language models.
Patel, A., Bhattamishra, S., and Goyal, N. (2021). Are NLP
models really able to solve simple math word prob-
lems? In Proceedings of the 2021 Conference of the
North American Chapter of the Association for Com-
putational Linguistics: Human Language Technolo-
gies, pages 2080–2094, Online. Association for Com-
putational Linguistics.
Pustejovsky, J. (2005). Time and the semantic Web. In 12th
International Symposium on Temporal Representation
and Reasoning (TIME’05), pages 5–8. ISSN: 2332-
6468.
Pustejovsky, J., Lee, K., Bunt, H., and Romary, L. (2010).
ISO-TimeML: An international standard for seman-
tic annotation. In Calzolari, N., Choukri, K., Mae-
gaard, B., Mariani, J., Odijk, J., Piperidis, S., Ros-
ner, M., and Tapias, D., editors, Proceedings of the
Seventh International Conference on Language Re-
sources and Evaluation (LREC’10), Valletta, Malta.
European Language Resources Association (ELRA).
Saparov, A. and He, H. (2023). Language models are
greedy reasoners: A systematic formal analysis of
chain-of-thought.
Srivastava, A. and et al. (2023). Beyond the imitation game:
Quantifying and extrapolating the capabilities of lan-
guage models. Transactions on Machine Learning Re-
search.
Str
¨
otgen, J. (2015). Domain-sensitive temporal tagging for
event-centric information retrieval.
Suzgun, M., Scales, N., Sch
¨
arli, N., Gehrmann, S., Tay, Y.,
Chung, H. W., Chowdhery, A., Le, Q. V., Chi, E. H.,
Zhou, D., and Wei, J. (2022). Challenging big-bench
tasks and whether chain-of-thought can solve them.
Team, G., Mesnard, T., Hardin, C., Dadashi, R., Bhupati-
raju, S., Pathak, S., Sifre, L., Rivi
`
ere, M., Kale, M. S.,
Love, J., Tafti, P., Hussenot, L., Sessa, P. G., Chowd-
hery, A., Roberts, A., Barua, A., Botev, A., Castro-
Ros, A., Slone, A., H
´
eliou, A., Tacchetti, A., Bu-
lanova, A., Paterson, A., Tsai, B., Shahriari, B., Lan,
C. L., Choquette-Choo, C. A., Crepy, C., Cer, D., Ip-
polito, D., Reid, D., Buchatskaya, E., Ni, E., Noland,
E., Yan, G., Tucker, G., Muraru, G.-C., Rozhdestven-
skiy, G., Michalewski, H., Tenney, I., Grishchenko, I.,
Austin, J., Keeling, J., Labanowski, J., Lespiau, J.-B.,
Stanway, J., Brennan, J., Chen, J., Ferret, J., Chiu, J.,
Mao-Jones, J., Lee, K., Yu, K., Millican, K., Sjoesund,
L. L., Lee, L., Dixon, L., Reid, M., Mikuła, M., Wirth,
M., Sharman, M., Chinaev, N., Thain, N., Bachem,
O., Chang, O., Wahltinez, O., Bailey, P., Michel, P.,
Yotov, P., Chaabouni, R., Comanescu, R., Jana, R.,
Anil, R., McIlroy, R., Liu, R., Mullins, R., Smith,
S. L., Borgeaud, S., Girgin, S., Douglas, S., Pandya,
S., Shakeri, S., De, S., Klimenko, T., Hennigan, T.,
Feinberg, V., Stokowiec, W., hui Chen, Y., Ahmed, Z.,
Gong, Z., Warkentin, T., Peran, L., Giang, M., Fara-
bet, C., Vinyals, O., Dean, J., Kavukcuoglu, K., Hass-
abis, D., Ghahramani, Z., Eck, D., Barral, J., Pereira,
F., Collins, E., Joulin, A., Fiedel, N., Senter, E., An-
dreev, A., and Kenealy, K. (2024). Gemma: Open
models based on gemini research and technology.
Valmeekam, K., Marquez, M., Olmo, A., Sreedharan, S.,
and Kambhampati, S. (2023). Planbench: An exten-
sible benchmark for evaluating large language models
on planning and reasoning about change.
van Lambalgen, M. and Hamm, F. (2006). The proper
treatment of events. Bulletin of Symbolic Logic,
12(1):139–141.
Vendler, Z. (1957). Verbs and times. The Philosophical
Review, 66(2):143–160.
Wei, J., Tay, Y., Bommasani, R., Raffel, C., Zoph, B.,
Borgeaud, S., Yogatama, D., Bosma, M., Zhou, D.,
Metzler, D., Chi, E. H., Hashimoto, T., Vinyals, O.,
Liang, P., Dean, J., and Fedus, W. (2022). Emergent
abilities of large language models.
Wei, J., Wang, X., Schuurmans, D., Bosma, M., Ichter, B.,
Xia, F., Chi, E., Le, Q., and Zhou, D. (2023). Chain-
of-thought prompting elicits reasoning in large lan-
guage models.
Xiong, S., Payani, A., Kompella, R., and Fekri, F. (2024).
Large language models can learn temporal reasoning.
KEOD 2024 - 16th International Conference on Knowledge Engineering and Ontology Development
82