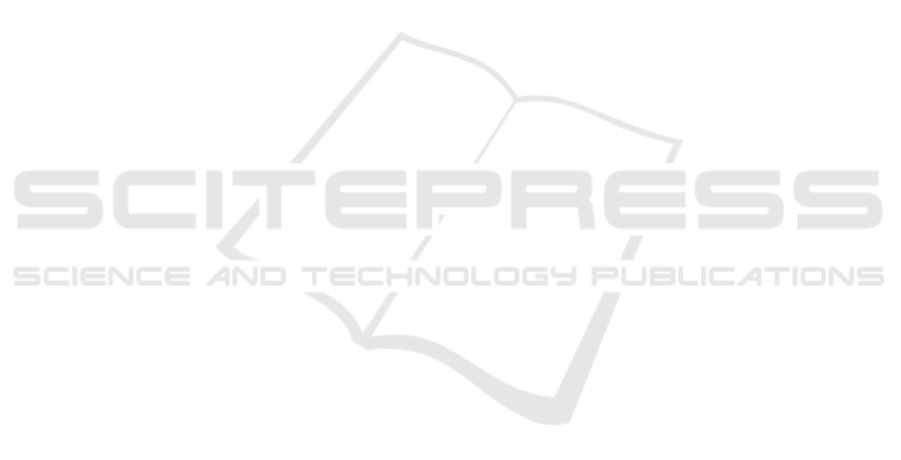
dynamic time decay. The J. of Supercomputing, 77(1),
244–262. https://doi.org/10.1007/s11227-020-03266-2
Chin, J. Y., Chen, Y., & Cong, G. (2022). The Datasets
Dilemma: How Much Do We Really Know About
Recommendation Datasets? Proceedings of the
Fifteenth ACM International Conference on Web
Search and Data Mining, 141–149.
https://doi.org/10.1145/3488560.3498519
Fareed, A., Hassan, S., Belhaouari, S. B., & Halim, Z.
(2023). A collaborative filtering recommendation
framework utilizing social networks. Machine Learning
with Applications, 14, 100495.
https://doi.org/10.1016/j.mlwa.2023.100495
Felfernig, A., Boratto, L., Stettinger, M., & Tkalčič, M.
(2018). Evaluating Group Recommender Systems. In
A. Felfernig, L. Boratto, M. Stettinger, & M. Tkalčič,
Group Recommender Systems (pp. 59–71). Springer.
https://doi.org/10.1007/978-3-319-75067-5_3
Fkih, F. (2022). Similarity measures for Collaborative
Filtering-based Recommender Systems: Review and
experimental comparison. Journal of King Saud
University – Comp. and Inf. Sciences, 34(9), 7645–
7669. https://doi.org/10.1016/j.jksuci.2021.09.014
Guo, G., Zhang, J., Thalmann, D., & Yorke-Smith, N.
(2014). ETAF: An extended trust antecedents
framework for trust prediction. 2014 IEEE/ACM
International Conference on Advances in Social
Networks Analysis and Mining, 540–547.
https://doi.org/10.1109/ASONAM.2014.6921639
Harper, F. M., & Konstan, J. A. (2016). The MovieLens
Datasets: History and Context. ACM Transactions on
Interactive Intelligent Systems, 5(4), 1–19.
https://doi.org/10.1145/2827872
Jain, G., Mahara, T., & Sharma, S. C. (2023). Performance
Evaluation of Time-based Recommendation System in
Collaborative Filtering Technique. Procedia Computer
Science, 218, 1834–1844. https://doi.org/10.1016/j.
procs.2023.01.161
Krichene, W., & Rendle, S. (2020). On Sampled Metrics
for Item Recommendation. Proceedings of the 26th
ACM SIGKDD International Conference on
Knowledge Discovery & Data Mining, 1748–1757.
https://doi.org/10.1145/3394486.3403226
Li, D., Jin, R., Gao, J., & Liu, Z. (2020). On Sampling Top-
K Recommendation Evaluation. Proceedings of the
26th ACM SIGKDD International Conference on
Knowledge Discovery & Data Mining, 2114–2124.
https://doi.org/10.1145/3394486.3403262
Margaris, D., Sgardelis, K., Spiliotopoulos, D., &
Vassilakis, C. (2024). Exploiting Rating Prediction
Certainty for Recommendation Formulation in
Collaborative Filtering. Big Data and Cognitive
Computing, 8(6), 53. https://doi.org/10.3390/
bdcc8060053
Margaris, D., Vassilakis, C., & Spiliotopoulos, D. (2020).
What makes a review a reliable rating in recommender
systems? Inf. Processing & Management, 57(6),
102304. https://doi.org/10.1016/j.ipm.2020.102304
Margaris, D., Vassilakis, C., & Spiliotopoulos, D. (2022).
On Producing Accurate Rating Predictions in Sparse
Collaborative Filtering Datasets. Information, 13(6),
302. https://doi.org/10.3390/info13060302
Nguyen, L. V., Vo, Q.-T., & Nguyen, T.-H. (2023).
Adaptive KNN-Based Extended Collaborative Filtering
Recommendation Services. Big Data and Cognitive
Computing, 7(2), 106. https://doi.org/10.3390/
bdcc7020106
Ni, J., Li, J., & McAuley, J. (2019). Justifying
Recommendations using Distantly-Labeled Reviews
and Fine-Grained Aspects. Proceedings of the 2019
Conference on Empirical Methods in Natural Language
Processing and the 9th International Joint Conference
on Natural Language Processing (EMNLP-IJCNLP),
188–197. https://doi.org/10.18653/v1/D19-1018
Sen, R., Goswami, S., & Chakraborty, B. (2019). Jeffries-
Matusita distance as a tool for feature selection. 2019
International Conference on Data Science and
Engineering (ICDSE), 15–20. https://doi.org/10.1109/
ICDSE47409.2019.8971800
Singh, P. K., Sinha, M., Das, S., & Choudhury, P. (2020).
Enhancing recommendation accuracy of item-based
collaborative filtering using Bhattacharyya coefficient
and most similar item. Applied Intel., 50(12), 4708–
4731. https://doi.org/10.1007/s10489-020-01775-4
Spiliotopoulos, D., Margaris, D., & Vassilakis, C. (2022).
On Exploiting Rating Prediction Accuracy Features in
Dense Collaborative Filtering Datasets. Information,
13(9), 428. https://doi.org/10.3390/info13090428
Trattner, C., Said, A., Boratto, L., & Felfernig, A. (2024).
Evaluating Group Recommender Systems. In A.
Felfernig, L. Boratto, M. Stettinger, & M. Tkalčič
(Eds.), Group Recommender Systems (pp. 63–75).
Springer Nature Switzerland. https://doi.org/10.1007/
978-3-031-44943-7_3
Vuong Nguyen, L., Nguyen, T., Jung, J. J., & Camacho, D.
(2021). Extending collaborative filtering
recommendation using word embedding: A hybrid
approach. Concurrency and Computation: Practice and
Experience, 35(16), e6232. https://doi.org/10.1002/
cpe.6232
Wang, D., Yih, Y., & Ventresca, M. (2020). Improving
neighbor-based collaborative filtering by using a hybrid
similarity measurement. Exp. Syst. with Appl., 160,
113651. https://doi.org/10.1016/j.eswa.2020.113651
Wang, R., Wu, Z., Lou, J., & Jiang, Y. (2022). Attention-
based dynamic user modeling and Deep Collaborative
filtering recommendation. Expert Systems with
Applications, 188, 116036. https://doi.org/10.1016/j.
eswa.2021.116036
Wang, Z. (2023). Intelligent recommendation model of
tourist places based on collaborative filtering and user
preferences. Applied Artificial Intel., 37(1), 2203574.
https://doi.org/10.1080/08839514.2023.2203574
Zhang, L., Li, Z., & Sun, X. (2021). Iterative rating
prediction for neighborhood-based collaborative
filtering. Applied Intelligence, 51(10), 6810–6822.
https://doi.org/10.1007/s10489-021-02237-1
Improving Recommendation Quality in Collaborative Filtering by Including Prediction Confidence Factors
379