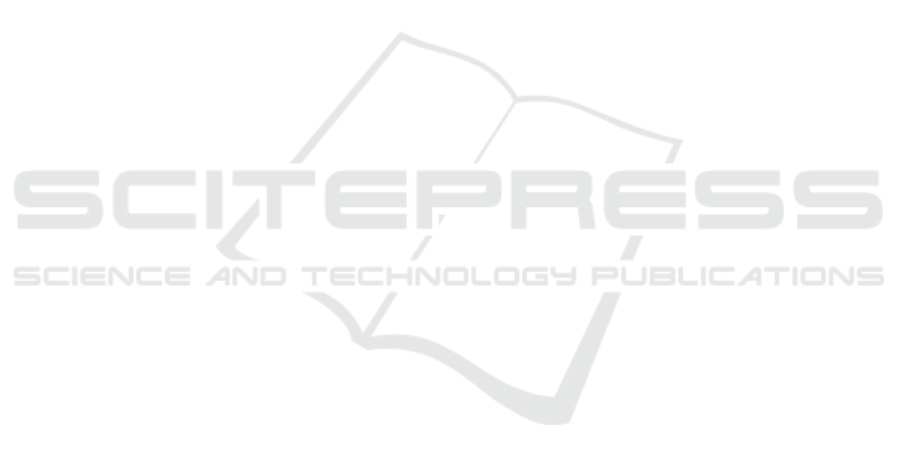
ing climate parameters alone will not help farmers and
feed the future generations.
7 CONCLUSION
In Senegal, agriculture dependent highly to soil types,
climate, soil salinity and water. This work provides a
set of results in order to know, how to optimize water
in different soil type and it proposes an agricultural
calendar for a good distribution of agriculture activi-
ties over time and find the relationship between crops.
Our results show the effectiveness of our solution to
avoid the dependences on raining season. This makes
possible to understand crops’ dependencies and an-
ticipate the agroecological phenomena, the planning
of production facilities and variations in agricultural
yields. In the future we aim to disseminate this tech-
nique in the other agroecological area of the Senegal.
Incorporate an analysis of the socio-economic impact
of our agricultural calendar on local communities by
selecting performances metrics and comparison with
traditional methods.
REFERENCES
ANSD (https://www.ansd.sn/regions, 2024). Agence
nationale de la statistique et de la demongra-
phie/accueil/regions.
Cheng, W., andXiaoting Wang, T. M., and Wang,
G. (2022). Anomaly detection for internet of
things time series data using generative adversar-
ial networks with attention mechanism in smart
agriculture. Frontiers in Plant Science, page
https://doi.org/10.3389/fpls.2022.890563.
Faye, P. F., Faye, J. A., and Senghor, M. (2024a). Decen-
tralized intelligence for smart agriculture. In Proceed-
ings of the 16th International Conference on Agents
and Artificial Intelligence (ICAART 2024), 1:240–
247, ISBN 978–989–758–680–4, ISSN 2184–433X.
DOI: 10.5220/0012342100003636.
Faye, P. F., Faye, J. A., and Senghor, M. (2024b). Machine
learning for a better agriculture calendar. In Proceed-
ings of the 19th International Conference on Software
Technologies(ICSOFT 2024), pages 307–314, ISBN
978–989–758–706–1, ISSN 2184–2833, pages 307–
314. DOI: 10.5220/0012691800003753.
Faye, P. F., Sall, M. M., Dembele, B., and Faye, J. A. A.
(2023). Plateforme num
´
erique de guides culturaux
dans un contexte de changement climatique et de
baisse des rendements agricoles. RAMReS Sciences
des Structures et de la Mati
´
ere, pages vol. 7, no. 1, pp.
85–115, ISSN : 2630–1180.
Faye, P. F., Senghor, M., and Ndione, G. A. (2022). Stable
trade coordinations in smart agriculture. International
Joint Conference on Web Intelligence and Intelligent
Agent Technology (WI-IAT), pages 215–222.
Feng, Q., Wang, S., Wang, H., Qin, Z., and Wang,
H. (2022). Circle fitting based image segmen-
tation and multi-scale block local binary pattern
based distinction of ring rot and anthracnose on
apple fruits. Frontiers in Plant Science, 13:doi:
10.3389/fpls.2022.884891.
Fu, L., Li, S., Rao, Y., Liang, J., Teng, J., and He, Q. (2022).
A novel heuristic target-dependent neural architecture
search method with small samples. Frontiers in Plant
Science, page 10.3389/fpls.2022.897883.
Maia, R. F., Lurbe, C. B., and Hornbuckle, J. (2022).
Machine learning approach to estimate soil matric
potential in the plant root zone based on remote
sensing data. Frontiers in Plant Science, page
https://doi.org/10.3389/fpls.2022.931491.
Onoda, T., Sakai, M., and Yamada, S. (2010). Careful seed-
ing based on independent component analysis for k-
means clustering. 2010 IEEE/WIC/ACM International
Conference on Web Intelligence and Intelligent Agent
Technology, 3:112–115.
Paswan, R. P. and Begum, S. A. (2013). Regression and
neural networks models for prediction of crop produc-
tion. International Journal of Scientific and Engineer-
ing Research, pages Volume 4, Issue 9,ISSN 2229–
5518.
Sellam, V. and Poovammal, E. (2010). Prediction of crop
yield using regression analysis. IEEE Trans. Knowl.
Data Eng., pages vol.23, no. 10, pp. 1498–1512.
Sun, M., Xu1, L., Luo, R., Lu, Y., and Jia, W. (2022).
Fast location and recognition of green apple based
on rgb-d image. Frontiers in Plant Science, 13:doi:
10.3389/fpls.2022.864458.
Umargono, E., Suseno, J. E., and K., V. G. S. (2020).
K-means clustering optimization using the elbow
method and early centroid determination based-on
mean and median. International Conferences on In-
formation System and Technology (CONRIST 2019,
DOI: 10.5220/0009908402340240:pages 234–240.
Uyeh, D. D., Iyiola, O., Mallipeddi, R., Asem-Hiablie,
S., Amaizu, M., Ha, Y., and Park, T. (2022). Grid
search for lowest root mean squared error in pre-
dicting optimal sensor location in protected culti-
vation systems. Frontiers in Plant Science, page
https://doi.org/10.3389/fpls.2022.920284.
Zhang, L., Lei, L., and Yan, D. (2010). Comparison of
two regression models for predicting crop yield. IEEE
International Symposium on Geoscience and Remote
Sensing (IGARSS).
Zingade, P. D., Buchade, O., Mehta, N., Ghodekar, S., and
Mehta, C. (2018). Machine learning-based crop pre-
diction system using multi-linear regression. Interna-
tional Journal of Emerging Technology and Computer
Science(IJETCS), pages Vol 3, Issue 2.
Water Optimization in Digital Farming
479