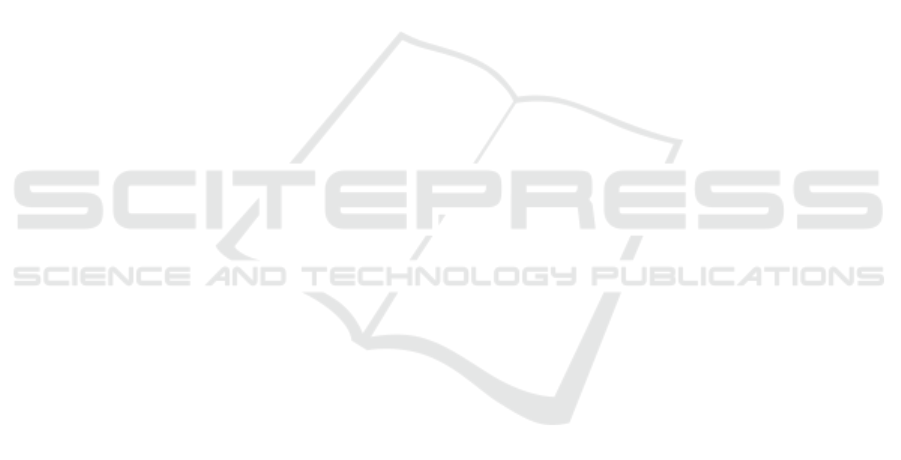
intents (Spatola and Chaminade, 2022). Regarding
the disturbance construct, the authors themselves
argue, that the scale is more ambiguous than the
others, which could result in larger variance in the
scenario at hand.
Limitations are given by the moderate sample
size and single interaction task and robot design.
More test persons and different interaction scenarios
may contribute to increase the effects and generalize
the findings.
6 CONCLUSIONS
In the presented human-robot cooperative pick-and-
place study, induction of cognitive load contributed
to a significant positive association of the
anthropomorphism dimensions sociability and
animacy with experienced mental demand and
cognitive load of the test persons. Variable strength
of anthropomorphism due to cognitive load variation
may influence cooperative task performance, which
is generally dependent on the level of perceived
human-likeness of a robot in manifold ways and thus
may impact human-robot interaction quality in a
variety of application scenarios.
Future work will target additional aspects, which
might result from different levels of
anthropomorphism due to cognitive load variation in
the context of safety and trust.
ACKNOWLEDGEMENTS
This paper is supported in part by DAAD.
REFERENCES
Mayer, M., Odenthal, B., Ewert, D., Kempf, T., Behnen, D.,
Bücher, C., Kuz, S., Hauck, E., Kausch, B., Schilberg,
D., Herfs, W., Schlick, C., Jeschke, S. and Brecher, C.
(2012). “Self-optimising assembly systems based on
cognitive technologies,” in Integrative Production
Technology for High-Wage Countries, C. Brecher, Ed.
Springer, pp. 877–990.
Brecher, C., Müller, S., Kuz, S. and Lohse, W. (2013).
“Towards anthropomorphic movements for industrial
robots, likeability, perceived intelli- gence, and
perceived safety of robots,” Proc. 4th Int. Conf. Digit.
Hum. Modeling and Applic in Health, Saf., Erg., and
Risk Man.
Kirsch, A., Kruse, T., Sisbot, A., Alami, R., Lawitzky, M.,
Brscic ́, D., Hirche, S., Basili, P. and Glasauer, S.
(2010). “Plan-based control of joint human-robot
activities,” KI, 24(3):223–231.
Faber, M., Bützler, J. and Schlick, C. (2015). “Human-robot
cooperation in future production systems: Analysis of
requirements for designing an ergonomic work
system,” Procedia Manufacturing, vol. 3, pp. 510– 517.
Shen, Y. and Reinhart, G. (2013). “Safe assembly motion -
a novel approach for applying human-robot co-
operation in hybrid assembly systems,” in Proc. IEEE
ICMA.
Bley, H., Reinhart, G., Seliger, G., Bernardi, M. and Korne,
T. (2004). “Appropriate human involvement in
assembly and disassembly,” CIRP Annals - Man. Tech.,
Elsevier,53:487–509,2004.
Petruck, H., Kuz, S., Mertens, A. and Schlick, C. (2016).
Increasing Safety in Human-Robot Collaboration by
Using Anthropomorphic Speed Profiles of Robot
Movements.
Spatola, N. and Chaminade, T. (2022). Cognitive load
increases anthropomorphism of humanoid robot. The
automatic path of anthropomorphism, International
Journal of Human-Computer Studies, Volume 167.
Mars, R. B., Neubert, F. X., Noonan, M. A. P., Sallet, J.,
Toni, I., Rushworth, M. F. S. (2012). On the
relationship between the “default mode network” and
the “social brain.” Front. Hum. Neurosci., 6, pp. 1-9.
Darlow, A. L., Sloman, S. A. (2010). Two systems of
reasoning: architecture and relation to emotion. Wiley
Interdisciplinary Reviews: Cognitive Science, 1,
pp. 382-392.
Evans, J. S. B. T., Stanovich, K. E. (2013). Dual-process
theories of higher cognition: advancing the debate.
Perspect. Psycholog. Sci., 8 (3), pp. 223-241.
Kilner, J., Paulignan, Y. and Blakemore, S. (2003). “An
interference effect of observed biological movement on
action,” Current Biology, vol. 13, no. 6, pp. 522–525.
Chaminade, T., Franklin, D., Oztop, E. and Cheng, G.
(2005). “Motor interference between humans and
humanoid robots: Effect of biological and artificial
motion,” Proc. IEEE ICDL.
Kühnlenz, K. and Kühnlenz, B. (2020). “Motor interference
of incongruent motions increases workload in close
HRI,” Advanced Robotics, vol. 34, no. 6, p. 400–406.
Kupferberg, A., Glasauer, S., Huber, M., Rickert, M.,
Knoll, A. and Brandt, T. (2011). “Biological movement
increases acceptance of humanoid robots as human
partners in motor interaction,” AI & Society, vol. 26, no.
4, pp. 339–345.
Spatola, N., Kühnlenz, B., Cheng, G. (2021). Perception
and evaluation in human–robot interaction: The
human–robot interaction evaluation scale (HRIES)—a
multicomponent approach of anthropomorphism.
International Journal of Social Robotics, 13(7), pp.
1517-1539.
Deck, C., Jahedi, S. and Sheremeta, R. (2021). On the
consistency of cognitive load, European Economic
Review, Volume 134.
ICINCO 2024 - 21st International Conference on Informatics in Control, Automation and Robotics
318