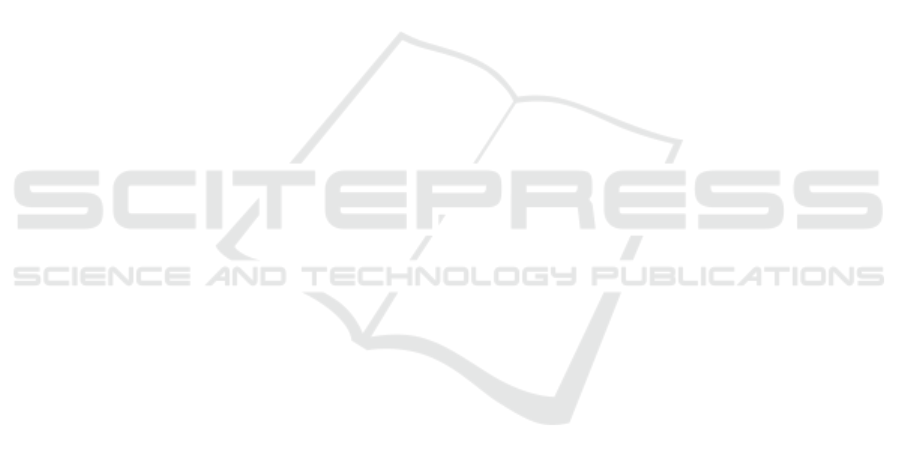
representations of knowledge maps of educational,
scientific and consulting activities, depending on the
stakeholder, the task itself, the selected level of
generalization for mapping
(institute/department/individual).
One of the key benefits of using this atlas is that
the maps from it help students, faculty and
administration see the big picture of the academic life.
By mapping out key concepts and their
interconnections, employees can gain a better
understanding of how different pieces of information
fit together, who are the experts and how they
contribute to the overall goals of the university unit in
teaching and research. This can help employees make
more informed decisions and work more effectively
towards shared objectives and smarter collaboration.
Systematic analysis of corporate, administrative
and scientific knowledge creates the potential to
significantly improve the quality of information
support, creating knowledge management systems for
more effective interaction between various groups of
organization employees and external users and
stakeholders.
Ultimately, using visual knowledge maps can lead
to smarter decision-making, more innovative
solutions, and a more efficient and effective
company. It is a step to visual organization (Bell,
Warren, & Schroeder, 2014).
ACKNOWLEDGEMENTS
Authors thank Dr. Dmitry Kudryavtsev, who was the
initiator of the project and started the research design,
and Miroslav Kubelsky for the digital support of
bibliometric maps. The work was carried out by
Gavrilova T.A., Alkanova O.N. as part of
METAKARTA PROJECT grant No. 23-21-00168,
https://rscf.ru/project/23-21-00168/.
REFERENCES
Aithal, A., Aithal, P. (2020). Development and Validation
of Survey Questionnaire & Experimental Data—A
Systematical Review-Based Statistical Approach.
International Journal of Management, Technology, and
Social Sciences (IJMTS), 5, 233-251.
Anthony, Jr, B. (2021). Information flow analysis of a
knowledge mapping-based system for university
alumni collaboration: a practical approach. Journal of
the Knowledge Economy, 12(2), 756-787.
Balaid, A., Abd Rozan, M.Z., Hikmi, S.N., and Memon, J.
(2016). Knowledge Maps: A Systematic Literature
Review and Directions for Future Research,
International Journal of Information Management, Vol
36, No. 3, 451–475.
Bell, E., Warren, S., and Schroeder, J. (2014). Introduction:
The visual organization. In The Routledge companion
to visual organization (pp. 1-16). Routledge.
Butt, S. A. (2021). et al. A knowledge map for ICT
integration in the silver economy. Procedia Computer
Science, (181), 93-701.
Faisal, H., Rahman, A., and Zaman, G. (2019). Knowledge
Mapping for Research Papers. International Journal of
Computer Science and Network Security, 19 (10), 158–
164.
Gardner, H. (2017). Smart Collaboration: How
Professionals and Their Firms Succeed by Breaking
Down Silos. Harvard Business Review Press.
Lengler, R., Eppler, M.J. (2007). A Periodic Table of
Visualization Methods. URL: https://www.visual-
literacy.org/periodic_table/periodic_table.html
Lengler, R., Eppler, M.J. (2007). Towards a periodic table
of visualization methods of management.
Liebowitz, J. (2005). Linking social network analysis with
the analytic hierarchy process for knowledge mapping
in organizations. J.of knowledge management,9,76–86.
Merriam-webster dictionary (https://www.merriam-
webster.com/dictionary/atlas#:)
Miller, F. The power of Diagrams.
https://www.francismiller.com/articles/
Moody, D. (2007). What makes a good diagram? improving
the cognitive effectiveness of diagrams in is
development. In Advances in Inform. Systems
Development: New Methods for the Networked
Society. Boston, MA: Springer US. 481-492.
Pereira, V., Bamel, U., Temouri, Y., Budhwar, P., Del
Giudice, M. (2023). Mapping the evolution, current
state of affairs and future research direction of
managing cross-border knowledge for innovation.
Intern. Business Review. – Vol. 32, No. 2, 101834.
Troise, C. (2022). Exploring knowledge visualization in the
digital age: an analysis of benefits and risks.
Management Decision, Vol. 60 No. 4, pp. 1116-1131.
https://doi.org/10.1108/MD-01-2021-0086
Zack, M., McKeen, J., and Singh, S. (2009). Knowledge
management and organizational performance: An
exploratory analysis. Journal of Knowledge
Management, 13(6), 392–409. https://doi.org/10.1108/
13673270910997088
KMIS 2024 - 16th International Conference on Knowledge Management and Information Systems
136