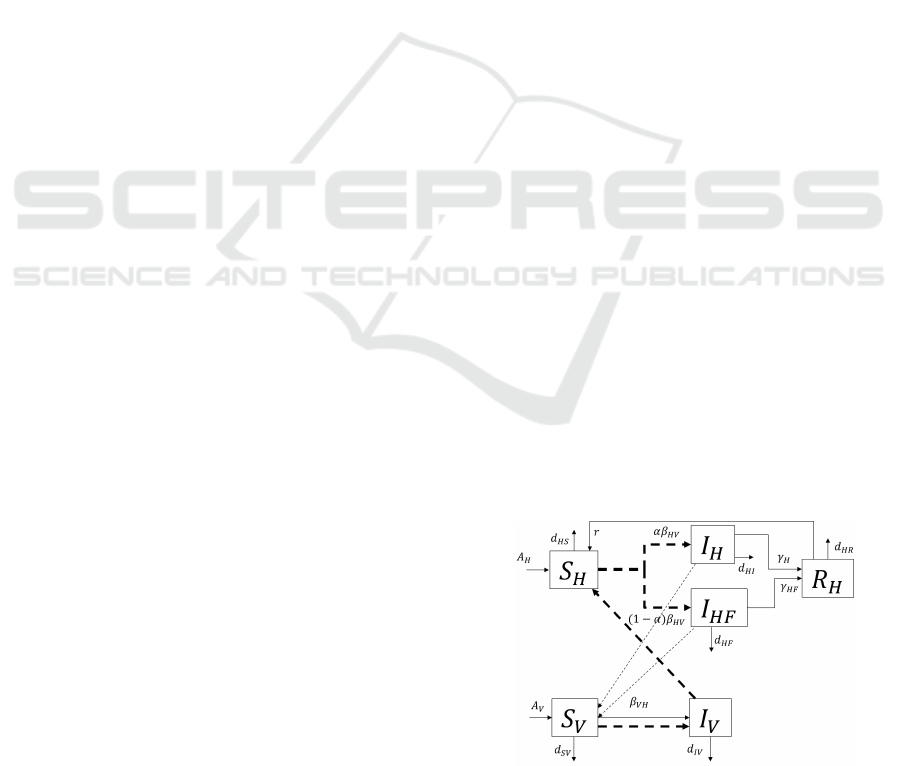
cessing egg-laying habitats.
Despite dengue diffusion depends on particular
environmental and climate conditions, climate change
and movement of population are making all the ar-
boviruses a global emergency, reaching regions not
involved up to few years ago.
Dengue has been studied in literature especially
since the last years of the 20
th
century, mainly using
the typical epidemic modelling considering the pecu-
liarity of the presence of a vector and a host to spread
the virus. An effective approach considers two in-
teracting models, one for human population and one
for the mosquitoes. For the former, a classical SEIR
model can be chosen, where S stands for the suscepti-
ble individuals, E for the exposed, that is for the sub-
jects infected but not infectious, I for the infected pa-
tients, R for the part of the population recovered or
dead. For the vector population a smaller one, for
example a SEI model is sufficient. This approach
is followed in (Yi et al., 2021), where the estimation
and the forecast of dengue outbreak dynamics are per-
formed by means of an ensemble Kalman filter. A
similar approach is implemented in (Schaum et al.,
2022), with reference to data from Mexico.
As said, a control of dengue spread could be im-
plemented by means of prevention, quarantining se-
vere patients, medical treatments and, in the last years
(in Europe only since 2022), also by vaccination. In
(Sow et al., 2024) it is shown that the most effec-
tive approach is a suitable integration of vaccination
and treatment. When contemporary different require-
ments are considered, especially in presence of re-
source limitation, optimal control, as confirmed by
previous results on different epidemics (Ayele et al.,
2021; Zhu et al., 2021; Di Giamberardino and Ia-
coviello, 2017), seems the framework for effective so-
lutions (Abidemi et al., 2024); the results put in evi-
dence the success of a coordinated application of all
the possible prevention actions.
A peculiarity of vector borne disease is the influ-
ence of climate factors on the vector presence and
diffusion. Favorable climate and environment con-
ditions, as well as prolonged weather anomalies, fa-
cilitate virus spread also in regions not used to such
epidemics, (Barcellos et al., 2024). This implies the
importance of suitably modelling the dynamics taking
account spatial and temporal diffusion of the virus;
in particular, the periodicity of dengue fever is re-
lated to local temperature, humidity and precipita-
tion characteristics, with an associated time-lag, (Xu
et al., 2024). An important contribution is given by
the study of data time series which, unfortunately, of-
ten are not available with lack in completeness and
consistency, (Arquam et al., 2020).
In this paper, it is proposed a model in which
the human population is described by a SIR model
where the set of infected patients is split into asymp-
tomatic patients, not aware of having contracted the
dengue virus and for which the infection does not pro-
duce consequences for their health, and the patients
who have severe dengue symptoms. The mosquitoes
population is described, in this preliminary study, by
means of a SI model, since the infection has no ef-
fects on the insect life. All the patients can infect an
uninfected mosquito; on the other hand, the infected
mosquito can spread the virus to susceptible humans.
Therefore, the complete model is represented by the
two populations with suitable interconnections.
The paper is organized as follows. The mathemat-
ical model is introduced and described in Section 2.
Section 3 is devoted to the model analysis, includ-
ing equilibria and stability, with particular emphasis
on the relationships with the epidemic spread charac-
teristics. Identification and validation procedures are
reported in Section 4, where some considerations on
the influence of dynamics characteristics, in term of
parameters values, to the qualitative behaviour of the
epidemics are reported. A concluding Section 5 ends
the paper.
2 THE MATHEMATICAL MODEL
The mathematical model adopted is based on the in-
teractions of two populations dynamics, the human
and the mosquitoes, with their natural growth and
with their mutual infectious interactions: a mosquito
biting an infected human becomes infectious, an in-
fected mosquito which bites a health human infects
the person in a light (that is without evident conse-
quences), or dangerous (with serious consequences,
even the death) way.
A block scheme is reported in Fig. 1 for a fast
visual representation.
Figure 1: Block diagram of the proposed model.
Modelling and Analysis of Spread Characteristics of Arbovirus Infections
601