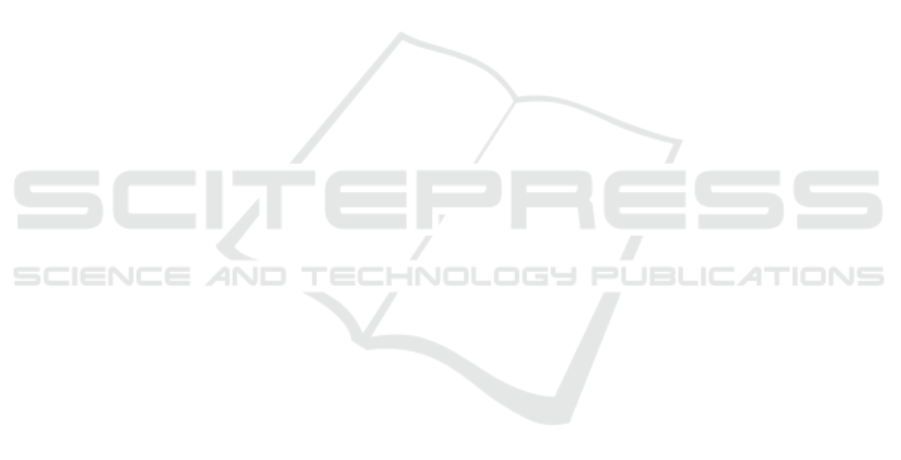
Crevelário de Melo R, Victoria Ribeiro AÂ, Luquine Jr
CD, et alEffectiveness and safety of yoga to treat
chronic and acute pain: a rapid review of systematic
reviews. British medical journal Open
2021;11:e048536. doi: 10.1136/bmjopen-2020-048536
Gothe, N. P., Khan, I., Hayes, J., Erlenbach, E., &
Damoiseaux, J. S. (2019). Yoga Effects on Brain
Health: A Systematic Review of the Current Literature.
Brain plasticity (Amsterdam, Netherlands), 5(1), 105–
122. https://doi.org/10.3233/BPL-190084
Büssing, A., Michalsen, A., Khalsa, S. B., Telles, S., &
Sherman, K. J. (2012). Effects of yoga on mental and
physical health: a short summary of reviews. Evidence-
based complementary and alternative medicine: eCAM,
2012, 165410. https://doi.org/10.1155/2012/165410
Sawyer, Amy & Martinez, Sarah & Warren, Gordon.
(2012). Impact of Yoga on Low Back Pain and
Function: A Systematic Review and Meta-Analysis.
Journal of Yoga and Physical Therapy. 2. 120.
10.4172/2157-7595.1000120.
Susilowati IH, Kurniawidjaja LM, Nugraha S, Nasri SM,
Pujiriani I, Hasiholan BP. The prevalence of bad posture
and musculoskeletal symptoms originating from the use
of gadgets as an impact of the work from home program
of the university community. Heliyon.
2022;8(10):e11059.
doi:10.1016/j.heliyon.2022.e11059
Salsali M, Sheikhhoseini R, Sayyadi P, Hides JA, Dadfar
M, Piri H. Association between physical activity and
body posture: a systematic review and meta-analysis.
BMC Public Health. 2023;23(1):1670. Published 2023
Aug 30. doi:10.1186/s12889-023-16617-4
Koren, Leon & Stipancic, Tomislav & Ricko, Andrija &
Orsag, Luka. (2022). Person Localization Model Based
on a Fusion of Acoustic and Visual Inputs. Electronics.
11. 440. 10.3390/electronics11030440.
Huang, Xiaoyang & Lin, Zhi & Huang, Shaohui & Wang,
Fu Lee & Chan, Moon-Tong & Wang, Liansheng.
(2022). Contrastive learning–guided multi-meta
attention network for breast ultrasound video diagnosis.
Frontiers in Oncology. 12. 10.3389/fonc.2022.952457.
Gabriele Guarnieri, Marco Fontani, Francesco Guzzi,
Sergio Carrato, Martino Jerian.Perspective registration
and multi-frame super-resolution of license plates in
surveillance videos. Forensic Science International:
Digital Investigation, Volume 36,2021,301087, ISSN
2666-2817. https://doi.org/10.1016/j.fsidi.2020.
301087.
Mousa Al-Akhras, Zainab Darwish, Samer Atawneh,
Mohamed Habib. Improving Association Rules
Accuracy in Noisy Domains Using Instance Reduction
Techniques, Computers, Materials and Continua.
Volume 72, Issue 2, 2022, Pages 3719-3749, ISSN
1546-2218.
https://doi.org/10.32604/cmc.2022.025196.
Siqi Ye, Shao-Ping Lu, Adrian Munteanu. Color correction
for large-baseline multiview video. Signal Processing:
Image Communication. Volume 53, 2017. Pages 40-50,
ISSN 0923-5965. https://doi.org/10.1016/j.image.
2017.01.004.
Anima Pramanik, Sobhan Sarkar, Sankar K. Pal. Video
surveillance-based fall detection system using object-
level feature thresholding and Z−numbers, Knowledge-
Based Systems Volume 280, 2023, 110992, ISSN 0950-
7051. https://doi.org/10.1016/j.knosys.2023.110992.
Shreya Talati, Darshan Vekaria, Aparna Kumari, Sudeep
Tanwar. An AI-driven object segmentation and speed
control scheme for autonomous moving platforms,
Computer Networks Volume 186, 2021, 107783, ISSN
1389-1286. https://doi.org/10.1016/j.comnet.2020.
107783.
Calvin Perumalla, LaDonna Kearse, Michael Peven,
Shlomi Laufer, Cassidi Goll, Brett Wise, Su Yang, Carla
Pugh, AI-Based Video Segmentation: Procedural Steps
or Basic Maneuvers? Journal of Surgical Research,
Volume 283, 2023, Pages 500-506, ISSN 0022-4804.
https://doi.org/10.1016/j.jss.2022.10.069."
Nidhi Tewathia, Anant Kamath, P. Vigneswara Ilavarasan,
Social inequalities, fundamental inequities, and
recurring of the digital divide: Insights from India,
Technology in Society, Volume 61, 2020, 101251, ISSN
0160-791X. https://doi.org/10.1016/j.techsoc.2020.
101251.
Rahmillah FI, Tariq A, King M, Oviedo-Trespalacios O.
Evaluating the Effectiveness of Apps Designed to
Reduce Mobile Phone Use and Prevent Maladaptive
Mobile Phone Use: Multimethod Study. J Med Internet
Res. 2023;25:e42541. Published 2023 Aug 29.
doi:10.2196/42541
Qingyue Tan, Gerui Lv, Xing Fang, Jiaxing Zhang, Zejun
Yang, Yuan Jiang, and Qinghua Wu. 2024. Accurate
Bandwidth Prediction for Real-Time Media Streaming
with Offline Reinforcement Learning. In Proceedings
of the 15th ACM Multimedia Systems Conference
(MMSys '24). Association for Computing Machinery,
New York, NY, USA, 381–387.
https://doi.org/10.1145/3625468. 3652183
W. Zhang et al., "A Streaming Cloud Platform for Real-
Time Video Processing on Embedded Devices," in
IEEE Transactions on Cloud Computing, vol. 9, no. 3,
pp. 868-880, 1 July-Sept. 2021, doi:
10.1109/TCC.2019.2894621.
Isabelle Kniestedt, Iulia Lefter, Stephan Lukosch, Frances
M. Brazier. Re-framing engagement for applied games:
A conceptual framework, Entertainment Computing,
Volume 41, 2022, 100475, ISSN 1875-9521.
https://doi.org/10.1016/j.entcom.2021.100475."
Kai-Yu Wang, Abdul R. Ashraf, Narongsak Tek
Thongpapanl, Oanh Nguyen. Influence of social
augmented reality app usage on customer relationships
and continuance intention: The role of shared social
experience, Journal of Business Research. Volume 166,
2023, 114092, ISSN 0148-2963,
https://doi.org/10.1016/j.jbusres.2023.114092"
Clare Sullivan. EU GDPR or APEC CBPR? A comparative
analysis of the approach of the EU and APEC to cross
border data transfers and protection of personal data in
the IoT era, Computer Law & Security Review, Volume
35, Issue 4, 2019, Pages 380-397, ISSN 0267-3649.
https://doi.org/10.1016/j.clsr.2019.05.004.
Leveraging AI to Mitigate Risks in Yoga Practice: A Real-Time Posture Correction Application
249