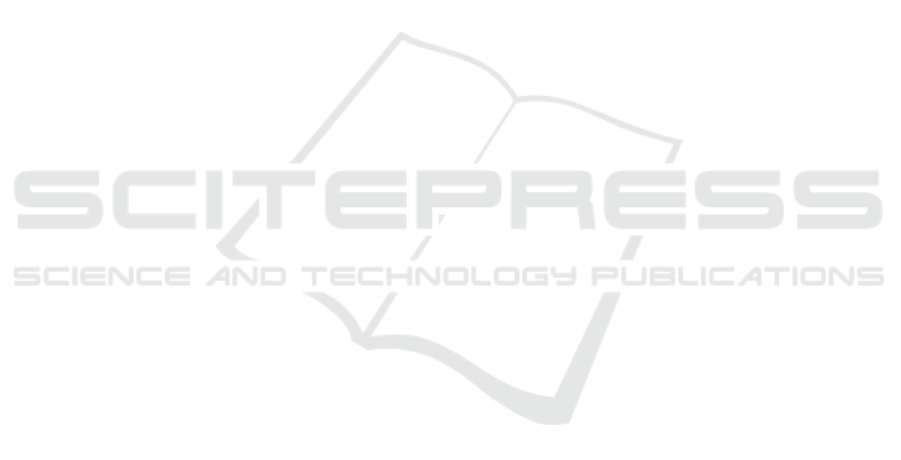
Berman, G. J., Choi, D. M., Bialek, W., and Shaevitz, J. W.
(2014). Mapping the stereotyped behaviour of freely
moving fruit flies. Journal of The Royal Society Inter-
face, 11(99):20140672.
Biderman, D., Whiteway, M. R., Hurwitz, C., Greenspan,
N., Lee, R. S., Vishnubhotla, A., Warren, R., Pedraja,
F., Noone, D., Schartner, M., et al. (2023). Light-
ning pose: improved animal pose estimation via semi-
supervised learning, bayesian ensembling, and cloud-
native open-source tools. BioRXiv.
Bohnslav, J. P., Wimalasena, N. K., Clausing, K. J., Dai,
Y. Y., Yarmolinsky, D. A., Cruz, T., Kashlan, A. D.,
Chiappe, M. E., Orefice, L. L., Woolf, C. J., et al.
(2021). Deepethogram, a machine learning pipeline
for supervised behavior classification from raw pixels.
Elife, 10:e63377.
Gharaee, Z., G
¨
ardenfors, P., and Johnsson, M. (2017). On-
line recognition of actions involving objects. Biologi-
cally inspired cognitive architectures, 22:10–19.
Gosztolai, A., G
¨
unel, S., Lobato-R
´
ıos, V., Pietro Abrate,
M., Morales, D., Rhodin, H., Fua, P., and Ramdya,
P. (2021). Liftpose3d, a deep learning-based ap-
proach for transforming two-dimensional to three-
dimensional poses in laboratory animals. Nature
methods, 18(8):975–981.
Graving, J. M., Chae, D., Naik, H., Li, L., Koger, B., Costel-
loe, B. R., and Couzin, I. D. (2019). Deepposekit, a
software toolkit for fast and robust animal pose esti-
mation using deep learning. Elife, 8:e47994.
G
¨
unel, S., Rhodin, H., Morales, D., Campagnolo, J.,
Ramdya, P., and Fua, P. (2019). Deepfly3d, a deep
learning-based approach for 3d limb and appendage
tracking in tethered, adult drosophila. Elife, 8:e48571.
Insafutdinov, E., Pishchulin, L., Andres, B., Andriluka, M.,
and Schiele, B. (2016). Deepercut: A deeper, stronger,
and faster multi-person pose estimation model. In
Computer Vision–ECCV 2016: 14th European Con-
ference, Amsterdam, The Netherlands, October 11-14,
2016, Proceedings, Part VI 14, pages 34–50. Springer.
Kabra, M., Robie, A. A., Rivera-Alba, M., Branson, S., and
Branson, K. (2013). Jaaba: interactive machine learn-
ing for automatic annotation of animal behavior. Na-
ture methods, 10(1):64–67.
Karashchuk, P., Rupp, K. L., Dickinson, E. S., Walling-
Bell, S., Sanders, E., Azim, E., Brunton, B. W., and
Tuthill, J. C. (2021). Anipose: A toolkit for robust
markerless 3d pose estimation. Cell reports, 36(13).
Lauer, J., Zhou, M., Ye, S., Menegas, W., Schneider, S.,
Nath, T., Rahman, M. M., Di Santo, V., Soberanes, D.,
Feng, G., et al. (2022). Multi-animal pose estimation,
identification and tracking with deeplabcut. Nature
Methods, 19(4):496–504.
Li, C. and Lee, G. H. (2023). Scarcenet: Animal pose esti-
mation with scarce annotations. In Proceedings of the
IEEE/CVF Conference on Computer Vision and Pat-
tern Recognition, pages 17174–17183.
Mathis, A., Mamidanna, P., Cury, K. M., Abe, T., Murthy,
V. N., Mathis, M. W., and Bethge, M. (2018).
Deeplabcut: markerless pose estimation of user-
defined body parts with deep learning. Nature neu-
roscience, 21(9):1281–1289.
Nilsson, S. R., Goodwin, N. L., Choong, J. J., Hwang, S.,
Wright, H. R., Norville, Z. C., Tong, X., Lin, D., Bent-
zley, B. S., Eshel, N., et al. (2020). Simple behavioral
analysis (simba)–an open source toolkit for computer
classification of complex social behaviors in experi-
mental animals. BioRxiv, pages 2020–04.
Pereira, T. D., Aldarondo, D. E., Willmore, L., Kislin,
M., Wang, S. S.-H., Murthy, M., and Shaevitz, J. W.
(2019). Fast animal pose estimation using deep neural
networks. Nature methods, 16(1):117–125.
Pereira, T. D., Tabris, N., Matsliah, A., Turner, D. M., Li, J.,
Ravindranath, S., Papadoyannis, E. S., Normand, E.,
Deutsch, D. S., Wang, Z. Y., et al. (2022). Sleap: A
deep learning system for multi-animal pose tracking.
Nature methods, 19(4):486–495.
Pfister, T., Simonyan, K., Charles, J., and Zisserman, A.
(2015). Deep convolutional neural networks for effi-
cient pose estimation in gesture videos. In Computer
Vision–ACCV 2014: 12th Asian Conference on Com-
puter Vision, Singapore, Singapore, November 1-5,
2014, Revised Selected Papers, Part I 12, pages 538–
552. Springer.
Pishchulin, L., Insafutdinov, E., Tang, S., Andres, B., An-
driluka, M., Gehler, P. V., and Schiele, B. (2016).
Deepcut: Joint subset partition and labeling for multi
person pose estimation. In Proceedings of the IEEE
conference on computer vision and pattern recogni-
tion, pages 4929–4937.
Segalin, C., Williams, J., Karigo, T., Hui, M., Zelikowsky,
M., Sun, J. J., Perona, P., Anderson, D. J., and
Kennedy, A. (2021). The mouse action recognition
system (mars) software pipeline for automated analy-
sis of social behaviors in mice. Elife, 10:e63720.
Tompson, J., Goroshin, R., Jain, A., LeCun, Y., and Bregler,
C. (2015). Efficient object localization using convo-
lutional networks. In Proceedings of the IEEE con-
ference on computer vision and pattern recognition,
pages 648–656.
Tompson, J. J., Jain, A., LeCun, Y., and Bregler, C. (2014).
Joint training of a convolutional network and a graph-
ical model for human pose estimation. Advances in
neural information processing systems, 27.
Toshev, A. and Szegedy, C. (2014). Deeppose: Human pose
estimation via deep neural networks. In Proceedings
of the IEEE conference on computer vision and pat-
tern recognition, pages 1653–1660.
Wiltschko, A. B., Johnson, M. J., Iurilli, G., Peterson,
R. E., Katon, J. M., Pashkovski, S. L., Abraira, V. E.,
Adams, R. P., and Datta, S. R. (2015). Mapping
sub-second structure in mouse behavior. Neuron,
88(6):1121–1135.
NCTA 2024 - 16th International Conference on Neural Computation Theory and Applications
620