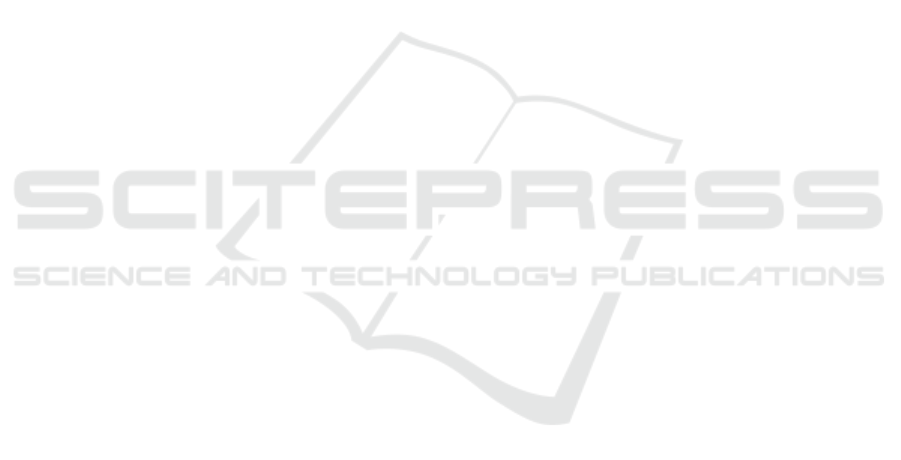
Hlomani, H. and Stacey, D. (2014). Approaches, methods,
metrics, measures, and subjectivity in ontology evalu-
ation: A survey. Semant. Web J., 1(5):1–11.
Johnson, A. E. W., Pollard, T. J., Shen, L., Lehman, L.-
w. H., Feng, M., Ghassemi, M., Moody, B., Szolovits,
P., Anthony Celi, L., and Mark, R. G. (2016). MIMIC-
III, a freely accessible critical care database. Sci.
Data, 3(1).
Ju, L., Yu, Z., Wang, L., Zhao, X., Wang, X., Bonnington,
P., and Ge, Z. (2024). Hierarchical Knowledge Guided
Learning for Real-World Retinal Disease Recogni-
tion. IEEE Trans. Med. Imaging, 43(1):335–350.
Karthik, S., Prabhu, A., Dokania, P. K., and Gandhi, V.
(2021). No Cost Likelihood Manipulation at Test
Time for Making Better Mistakes in Deep Networks.
arXiv:2104.00795 [cs].
Lourdusamy, R. and John, A. (2018). A review on metrics
for ontology evaluation. In Proc. ICISC, pages 1415–
1421.
Ma, F., Wang, Y., Xiao, H., Yuan, Y., Chitta, R., Zhou, J.,
and Gao, J. (2019). Incorporating medical code de-
scriptions for diagnosis prediction in healthcare. BMC
Med. Inf. Decis. Making, 19(6).
Ma, F., You, Q., Xiao, H., Chitta, R., Zhou, J., and Gao, J.
(2018). KAME: Knowledge-based Attention Model
for Diagnosis Prediction in Healthcare. In Proc.
CIKM, pages 743–752.
Marino, K., Salakhutdinov, R., and Gupta, A. (2017). The
More You Know: Using Knowledge Graphs for Image
Classification. arXiv:1612.04844 [cs].
Mc Gurk, S., Abela, C., and Debattista, J. (2017). Towards
ontology quality assessment. In Proc. LDQ2017,
pages 94–106.
McDaniel, M. and Storey, V. C. (2020). Evaluating Domain
Ontologies: Clarification, Classification, and Chal-
lenges. ACM Comput. Surv., 52(4).
Min, H., Mobahi, H., Irvin, K., Avramovic, S., and Woj-
tusiak, J. (2017). Predicting activities of daily living
for cancer patients using an ontology-guided machine
learning methodology. J. Biomed. Semant., 8(1).
Min, H. and Wojtusiak, J. (2012). Clinical data analysis
using ontology-guided rule learning. In Proc. MIXHS,
pages 17–22.
Mitchell, T. M. (1997). Machine learning. McGraw-Hill
New York.
Nickel, M. and Kiela, D. (2017). Poincar
´
e Embeddings
for Learning Hierarchical Representations. In Proc.
NIPS.
Northcutt, C., Jiang, L., and Chuang, I. (2021). Confident
learning: Estimating uncertainty in dataset labels. J.
Artif. Intell. Res., 70:1373–1411.
Ohta, M., Kozaki, K., and Mizoguchi, R. (2011). A Quality
Assurance Framework for Ontology Construction and
Refinement. In Proc. AWIC, pages 207–216.
Pittet, P. and Barth
´
el
´
emy, J. (2015). Exploiting Users’ Feed-
backs - Towards a Task-based Evaluation of Applica-
tion Ontologies Throughout Their Lifecycle:. In Proc.
IC3K, pages 263–268.
Porzel, R. and Malaka, R. (2004). A task-based approach
for ontology evaluation. In Proc. ECAI Workshop On-
tol. Learn. Popul.
Rezende, P. M., Xavier, J. S., Ascher, D. B., Fernandes,
G. R., and Pires, D. E. V. (2022). Evaluating hierarchi-
cal machine learning approaches to classify biological
databases. Briefings Bioinf., 23(4).
Rigoni, D., Elliott, D., and Frank, S. (2023). Cleaner Cat-
egories Improve Object Detection and Visual-Textual
Grounding. In Proc. SCIA, pages 412–442.
Silla, C. N. and Freitas, A. A. (2011). A survey of hierarchi-
cal classification across different application domains.
Data Min. Knowl. Discovery, 22(1-2):31–72.
Vendrov, I., Kiros, R., Fidler, S., and Urtasun, R.
(2016). Order-Embeddings of Images and Language.
arXiv:1511.06361 [cs].
von Rueden, L., Mayer, S., Beckh, K., Georgiev, B., Gies-
selbach, S., Heese, R., Kirsch, B., Pfrommer, J., Pick,
A., Ramamurthy, R., Walczak, M., Garcke, J., Bauck-
hage, C., and Schuecker, J. (2023). Informed Machine
Learning – A Taxonomy and Survey of Integrating
Prior Knowledge into Learning Systems. IEEE Trans.
Knowl. Data Eng., 35(1):614–633.
Wang, H., Wang, H., and Xu, K. (2018). Categorizing con-
cepts with basic level for vision-to-language. In Proc.
CVPR, pages 4962–4970.
Willard, J., Jia, X., Xu, S., Steinbach, M., and Kumar, V.
(2023). Integrating Scientific Knowledge with Ma-
chine Learning for Engineering and Environmental
Systems. ACM Comput. Surv., 55(4).
Wilson, R. S. I., Goonetillake, J. S., Indika, W. A., and
Ginige, A. (2022). A conceptual model for ontology
quality assessment. Semant. Web, 14(6):1051–1097.
Yin, C., Zhao, R., Qian, B., Lv, X., and Zhang, P.
(2019). Domain Knowledge Guided Deep Learn-
ing with Electronic Health Records. In Proc. ICDM,
pages 738–747.
Yu, T. (2007). Incorporating Prior Domain Knowledge into
Inductive Machine Learning: its Implementation in
Contemporary Capital Markets. PhD thesis, Univer-
sity of Technology Sydney, Australia.
Zeng, C., Zhou, W., Li, T., Shwartz, L., and Grabarnik,
G. Y. (2017). Knowledge Guided Hierarchical Multi-
Label Classification Over Ticket Data. IEEE Trans.
Netw. Serv. Manage., 14(2):246–260.
KEOD 2024 - 16th International Conference on Knowledge Engineering and Ontology Development
226