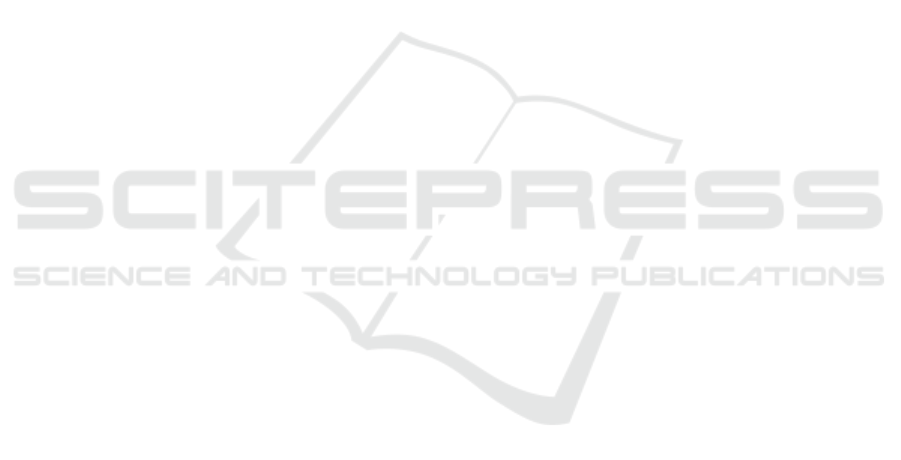
nance of possession attacks. In the match against Real
Madrid, the team performed defensive tactical actions
most of the time, indicating a struggle against a strong
opponent. In the match against Getafe, although of-
fensive tactical actions were predominant, the score
was 0-0. The estimation results predict that Barcelona
struggled to convert their attacks into goals. In fact,
Barcelona’s expected goals (xG) in this match were
significantly higher than Getafe’s, but they failed to
score. From these observations, we confirmed that
the estimation results align with the objective match
content. Furthermore, by representing match situa-
tions using the combination of tactical actions of the
two teams, we demonstrated the potential to predict
match situations.
6 CONCLUSION
In this study, we propose a new method for estimating
overlapped tactical actions from soccer match videos.
We enable the estimation of overlapped tactical ac-
tions considering exclusive relationships. We achieve
this by having the deep learning model learn all tacti-
cal actions simultaneously. We handcraft a large num-
ber of tactical actions based on simple definitions to
estimate tactical actions using deep learning. We esti-
mate tactical actions in deep learning by focusing on
the temporal changes of the positional relationships
among 22 players and between the ball and the play-
ers.
We validated the estimation of overlapped tactical
actions considering exclusive relationships by using
10 matches. We succeeded in expressing the tacti-
cal actions performed at a given time in terms of the
strength of several tactical actions. In the ablation
study, we confirmed the validity of the estimation re-
sults.
ACKNOWLEDGEMENTS
Part of this research is supported by JSPS KAKENHI
Grand Number 21H03476 and 21H05300.
REFERENCES
Barnerat, T., Crevoisier, J., Hoek, F., Redon, P., and
Ritschard, M. (2000). FIFA Coaching. Druckerei
Feldegg AG.
Bauer, P. and Anzer, G. (2021). Data-driven detection of
counterpressing in professional football: A supervised
machine learning task based on synchronized posi-
tional and event data with expert-based feature extrac-
tion. Data Mining and Knowledge Discovery, 35.
Bialkowski, A., Lucey, P., Carr, P., Yue, Y., Sridharan, S.,
and Matthews, I. (2014). Large-scale analysis of soc-
cer matches using spatiotemporal tracking data. In
2014 IEEE International Conference on Data Mining,
pages 725–730.
Fassmeyer, D., Anzer, G., Bauer, P., and Brefeld, U. (2021).
Toward automatically labeling situations in soccer.
Frontiers in Sports and Active Living, 3.
FIFA Training Centre (2022). EFI Explanation
Documents (PDF) — fifatrainingcentre.com.
https://www.fifatrainingcentre.com/en/fwc2022/
efi-metrics/efi-metrics-pdfs.php. [accessed on 13th
July 2024].
Forcher, L., Altmann, S., Forcher, L., Jekauc, D., and
Kempe, M. (2022). The use of player tracking data to
analyze defensive play in professional soccer - a scop-
ing review. International journal of Sports Science &
Coaching, 17:174795412210757.
Kamiya, K., Nakanishi, W., and Izumi, Y. (2017). Track-
ing data to extract changes in football game situation.
Proceedings of the Institute of Statistical Mathemat-
ics, 65.
Kobayashi, Y., Kawamura, H., and Suzuki, K. (2012).
Counter attack detection with machine learning from
log files of robocup simulation. In The 6th Interna-
tional Conference on Soft Computing and Intelligent
Systems, and The 13th International Symposium on
Advanced Intelligence Systems, pages 1821–1826.
Lucey, P., Bialkowski, A., Carr, P., Foote, E., and Matthews,
I. (2012). Characterizing multi-agent team behavior
from partial team tracings: Evidence from the english
premier league. In The AAAI Conference on Artificial
Intelligence, pages 1387–1393.
Lucey, P., Bialkowski, A., Monfort, M., Carr, P., and
Matthews, I. (2015). ”quality vs quantity”: Improved
shot prediction in soccer using strategic features from
spatiotemporal data. In The 8th Annual MIT Sloan
Sports Analytics Conference, pages 1–9.
Sigari, M.-H., Soltanian-Zadeh, H., Kiani, V., and Pour-
reza, A.-R. (2015). Counterattack detection in broad-
cast soccer videos using camera motion estimation. In
2015 The International Symposium on Artificial Intel-
ligence and Signal Processing (AISP), pages 101–106.
Suzuki, G., Takahashi, S., Ogawa, T., and Haseyama, M.
(2020). An estimation method of candidate region
for superimposing information based on gaze track-
ing data in soccer videos. In 2020 IEEE Interna-
tional Conference on Consumer Electronics - Taiwan
(ICCE-Taiwan), pages 1–2.
Wang, Q., Zhu, H., Hu, W., Shen, Z., and Yao, Y. (2015).
Discerning tactical patterns for professional soccer
teams: an enhanced topic model with applications. In
The 21th ACM SIGKDD International Conference on
Knowledge Discovery and Data Mining, pages 2197–
2206.
icSPORTS 2024 - 12th International Conference on Sport Sciences Research and Technology Support
264