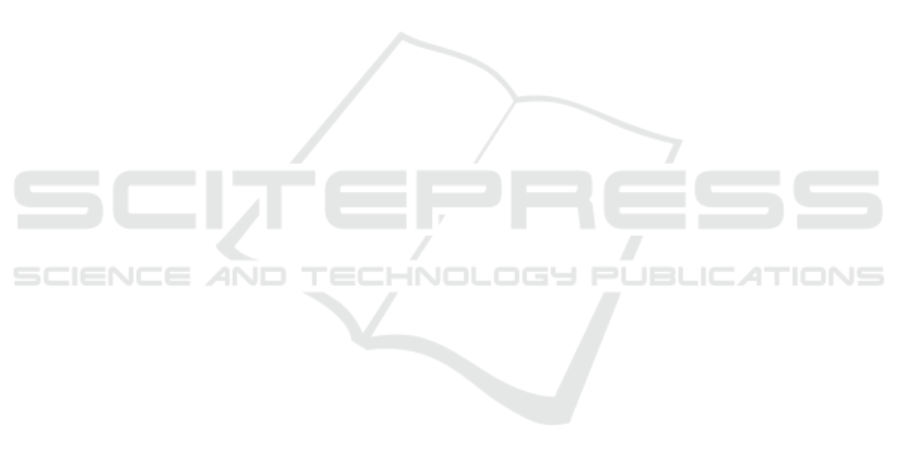
termined their weaknesses, and proposed essential im-
provements to the pipeline. Our improvements led to
an increase in performance in all three aspects of the
answer attribution process. We hope our study will
help future developments of this emerging NLP task.
REFERENCES
Afzal., A., Vladika., J., Braun., D., and Matthes., F. (2023).
Challenges in domain-specific abstractive summariza-
tion and how to overcome them. In Proceedings of
the 15th International Conference on Agents and Artifi-
cial Intelligence - Volume 3: ICAART, pages 682–689.
INSTICC, SciTePress.
Augenstein, I., Baldwin, T., Cha, M., Chakraborty, T.,
Ciampaglia, G. L., Corney, D., DiResta, R., Ferrara,
E., Hale, S., Halevy, A., Hovy, E., Ji, H., Menczer, F.,
Miguez, R., Nakov, P., Scheufele, D., Sharma, S., and
Zagni, G. (2023). Factuality challenges in the era of
large language models.
Bohnet, B., Tran, V. Q., Verga, P., Aharoni, R., Andor, D.,
Soares, L. B., Ciaramita, M., Eisenstein, J., Ganchev,
K., Herzig, J., Hui, K., Kwiatkowski, T., Ma, J., Ni, J.,
Saralegui, L. S., Schuster, T., Cohen, W. W., Collins,
M., Das, D., Metzler, D., Petrov, S., and Webster, K.
(2023). Attributed question answering: Evaluation and
modeling for attributed large language models.
Chen, S., Buthpitiya, S., Fabrikant, A., Roth, D., and Schus-
ter, T. (2023a). PropSegmEnt: A large-scale corpus for
proposition-level segmentation and entailment recogni-
tion. In Findings of the Association for Computational
Linguistics: ACL 2023.
Chen, S., Zhang, H., Chen, T., Zhou, B., Yu, W., Yu, D.,
Peng, B., Wang, H., Roth, D., and Yu, D. (2023b).
Sub-sentence encoder: Contrastive learning of propo-
sitional semantic representations. arXiv preprint
arXiv:2311.04335.
Chung, H. W., Hou, L., Longpre, S., Zoph, B., Tay, Y.,
Fedus, W., Li, Y., Wang, X., Dehghani, M., Brahma, S.,
Webson, A., Gu, S. S., Dai, Z., Suzgun, M., Chen, X.,
Chowdhery, A., Castro-Ros, A., Pellat, M., Robinson,
K., Valter, D., Narang, S., Mishra, G., Yu, A., Zhao, V.,
Huang, Y., Dai, A., Yu, H., Petrov, S., Chi, E. H., Dean,
J., Devlin, J., Roberts, A., Zhou, D., Le, Q. V., and
Wei, J. (2022). Scaling instruction-finetuned language
models.
Dhuliawala, S., Komeili, M., Xu, J., Raileanu, R., Li, X.,
Celikyilmaz, A., and Weston, J. (2023). Chain-of-
verification reduces hallucination in large language
models.
Douze, M., Guzhva, A., Deng, C., Johnson, J., Szilvasy, G.,
Mazar
´
e, P.-E., Lomeli, M., Hosseini, L., and J
´
egou, H.
(2024). The faiss library.
Gao, L., Dai, Z., Pasupat, P., Chen, A., Chaganty, A. T., Fan,
Y., Zhao, V., Lao, N., Lee, H., Juan, D.-C., and Guu,
K. (2023). RARR: Researching and revising what lan-
guage models say, using language models. In Rogers,
A., Boyd-Graber, J., and Okazaki, N., editors, Proceed-
ings of the 61st Annual Meeting of the Association for
Computational Linguistics (Volume 1: Long Papers),
pages 16477–16508, Toronto, Canada. Association for
Computational Linguistics.
Gao, T., Yao, X., and Chen, D. (2021). SimCSE: Simple con-
trastive learning of sentence embeddings. In Empirical
Methods in Natural Language Processing (EMNLP).
Guo, Z., Schlichtkrull, M., and Vlachos, A. (2022). A survey
on automated fact-checking. Transactions of the Asso-
ciation for Computational Linguistics, 10:178–206.
Ji, Z., Lee, N., Frieske, R., Yu, T., Su, D., Xu, Y., Ishii, E.,
Bang, Y. J., Madotto, A., and Fung, P. (2023). Survey
of hallucination in natural language generation. ACM
Computing Surveys, 55(12):1–38.
Kaddour, J., Harris, J., Mozes, M., Bradley, H., Raileanu, R.,
and McHardy, R. (2023). Challenges and applications
of large language models.
Kamalloo, E., Jafari, A., Zhang, X., Thakur, N., and Lin, J.
(2023). HAGRID: A human-llm collaborative dataset
for generative information-seeking with attribution.
arXiv:2307.16883.
Kasneci, E., Seßler, K., K
¨
uchemann, S., Bannert, M.,
Dementieva, D., Fischer, F., Gasser, U., Groh, G.,
G
¨
unnemann, S., H
¨
ullermeier, E., et al. (2023). Chatgpt
for good? on opportunities and challenges of large lan-
guage models for education. Learning and individual
differences, 103:102274.
Laurer, M., van Atteveldt, W., Casas, A., and Welbers, K.
(2024). Less annotating, more classifying: Addressing
the data scarcity issue of supervised machine learn-
ing with deep transfer learning and bert-nli. Political
Analysis, 32(1):84–100.
Lewis, M. (2023). Generative artificial intelligence and
copyright current issues. Morgan Lewis LawFlash.
Li, D., Sun, Z., Hu, X., Liu, Z., Chen, Z., Hu, B., Wu, A.,
and Zhang, M. (2023). A survey of large language
models attribution.
Li, X. and Li, J. (2023). Angle-optimized text embeddings.
Liu, N., Zhang, T., and Liang, P. (2023). Evaluating verifi-
ability in generative search engines. In Bouamor, H.,
Pino, J., and Bali, K., editors, Findings of the Associ-
ation for Computational Linguistics: EMNLP 2023,
pages 7001–7025, Singapore. Association for Compu-
tational Linguistics.
Liu, Y., Yao, Y., Ton, J.-F., Zhang, X., Guo, R., Cheng,
H., Klochkov, Y., Taufiq, M. F., and Li, H. (2024).
Trustworthy llms: a survey and guideline for evaluating
large language models’ alignment.
Malaviya, C., Lee, S., Chen, S., Sieber, E., Yatskar, M., and
Roth, D. (2024). ExpertQA: Expert-curated questions
and attributed answers. In 2024 Annual Conference
of the North American Chapter of the Association for
Computational Linguistics.
Min, S., Krishna, K., Lyu, X., Lewis, M., Yih, W.-t., Koh, P.,
Iyyer, M., Zettlemoyer, L., and Hajishirzi, H. (2023).
FActScore: Fine-grained atomic evaluation of factual
precision in long form text generation. In Bouamor,
H., Pino, J., and Bali, K., editors, Proceedings of the
2023 Conference on Empirical Methods in Natural
Language Processing, pages 12076–12100, Singapore.
Association for Computational Linguistics.
Enhancing Answer Attribution for Faithful Text Generation with Large Language Models
155