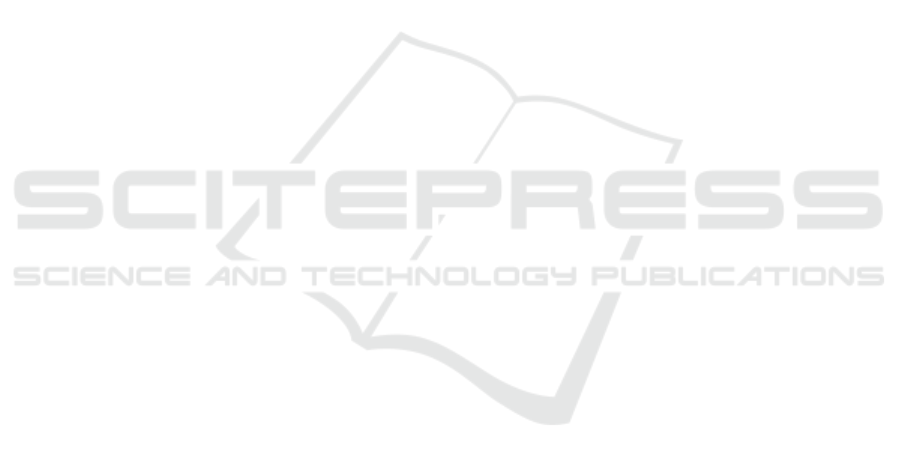
randomly chosen and the number of variables with
weights greater than 0 and percentage of selected vari-
ables among the 784 variables for each value of C is
given in Table 4.
The reconstruction results using these hyperpa-
rameter values for some images in the test dataset are
shown in Figure 4 and it can be noticed that U-FS-
ELM has reduced the number of features while keep-
ing useful information. It should be noted that this
approach is different from reduction methods which
determine a representation of the data in a subspace
while here a selection of important variables is done.
5 CONCLUSIONS
In this paper, an approach is proposed to deal with un-
supervised feature selection problems exploiting non-
linear relationships between variables. It consists of
assigning to each feature i a weight α
i
∈[0, 1] updated
during the reconstruction of the input variables and of
determining hyperparameters λ and C which are re-
spectively parameters for stability and sparsity. By
tuning these hyperparameters according to MSE, the
weights α
i
associated to the features make it possi-
ble to determine important features while minimizing
the reconstruction error. Many experiments have been
done on two synthetic data, three structured continu-
ous real-world data, and one image data and the re-
sults have been compared with other methods. They
show the effectiveness of the proposed approach.
REFERENCES
Cai, D., Zhang, C., and He, X. (2010). Unsupervised fea-
ture selection for multi-cluster data. In Proceedings
of the 16th ACM SIGKDD international conference
on Knowledge discovery and data mining, pages 333–
342.
Challita, N., Khalil, M., and Beauseroy, P. (2016). New
feature selection method based on neural network and
machine learning.
Deng, L. (2012). The mnist database of handwritten digit
images for machine learning research. IEEE Signal
Processing Magazine, 29(6):141–142.
Ding, C., Zhou, D., He, X., and Zha, H. (2006). R 1-
pca: Rotational invariant l 1-norm principal compo-
nent analysis for robust subspace factorization. In
ICML 2006 - Proceedings of the 23rd International
Conference on Machine Learning.
Han, K., Wang, Y., Zhang, C., Li, C., and Xu, C. (2018).
Autoencoder inspired unsupervised feature selection.
In 2018 IEEE international conference on acoustics,
speech and signal processing (ICASSP), pages 2941–
2945. IEEE.
He, X., Cai, D., and Niyogi, P. (2005). Laplacian score for
feature selection.
Hornik, K. (1991). Approximation capabilities of mul-
tilayer feedforward networks. Neural networks,
4(2):251–257.
Hotelling, H. (1933). Analysis of a complex of statistical
variables into principal components. Journal of edu-
cational psychology, 24(6):417.
Kanout
´
e, M., Grall-Ma
¨
es, E., and Beauseroy, P. (2023).
Neural network-based approach for supervised non-
linear feature selection. In Proceedings of the 15th In-
ternational Joint Conference on Computational Intel-
ligence - Volume 1: NCTA, pages 431–439. INSTICC,
SciTePress.
Mirzaei, A., Pourahmadi, V., Soltani, M., and Sheikhzadeh,
H. (2020). Deep feature selection using a teacher-
student network. Neurocomputing, 383:396–408.
Noble, B. and Daniel, J. W. (1997). Applied linear algebra.
2nd ed.
Paquette, S., Gordon, C. C., and Bradtmiller, B. (2009). An-
thropometric survey (ansur) ii pilot study: Methods
and summary statistics.
Redmond, M. (2009). Communities and Crime.
UCI Machine Learning Repository. DOI:
https://doi.org/10.24432/C53W3X.
Schmidt, W., Kraaijveld, M., and Duin, R. (1992). Feed-
forward neural networks with random weights. In
Proceedings., 11th IAPR International Conference on
Pattern Recognition. Vol.II. Conference B: Pattern
Recognition Methodology and Systems, pages 1–4.
Solorio-Fern
´
andez, S., Carrasco-Ochoa, J. A., and
Mart
´
ınez-Trinidad, J. F. (2020). A review of unsuper-
vised feature selection methods. Artificial Intelligence
Review, 53(2):907–948.
Tenenbaum, J. B., Silva, V. d., and Langford, J. C. (2000).
A global geometric framework for nonlinear dimen-
sionality reduction. science, 290(5500):2319–2323.
Van der Maaten, L. and Hinton, G. (2008). Visualizing data
using t-sne. Journal of machine learning research,
9(11).
Wang, S., Tang, J., and Liu, H. (2015). Embedded unsuper-
vised feature selection. In Proceedings of the AAAI
conference on artificial intelligence, volume 29.
Zhu, P., Zuo, W., Zhang, L., Hu, Q., and Shiu, S. C. (2015).
Unsupervised feature selection by regularized self-
representation. Pattern Recognition, 48(2):438–446.
Zwitter, M. and Soklic, M. (1988). Breast Can-
cer. UCI Machine Learning Repository. DOI:
https://doi.org/10.24432/C51P4M.
NCTA 2024 - 16th International Conference on Neural Computation Theory and Applications
628