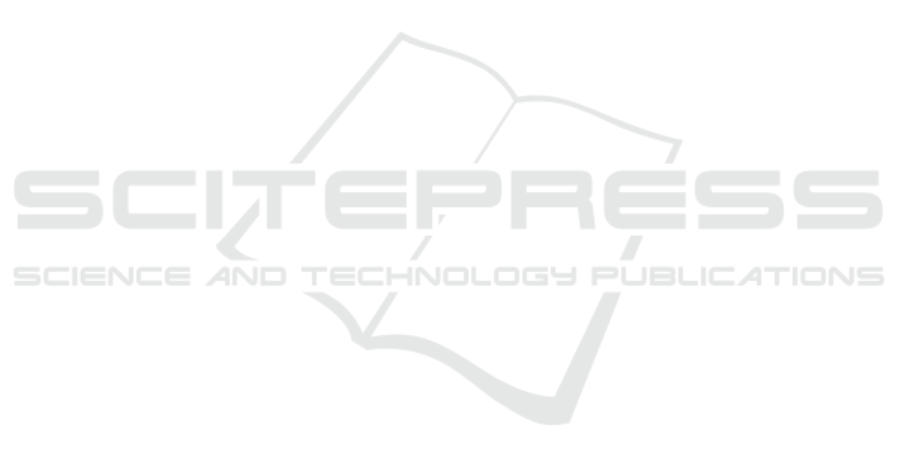
Lens 25m dataset. https://grouplens.org/datasets/
movielens/25m/.
Khan, Z. Y., Niu, Z., Sandiwarno, S., and Prince, R. (2021).
Deep learning techniques for rating prediction: a sur-
vey of the state-of-the-art. Artificial Intelligence Re-
view, 54(1):95–135.
Kuang, H., Xia, W., Ma, X., and Liu, X. (2021). Deep
matrix factorization for cross-domain recommenda-
tion. In 2021 IEEE 5th Advanced Information Tech-
nology, Electronic and Automation Control Confer-
ence (IAEAC), volume 5, pages 2171–2175. IEEE.
Liang, G., Sun, C., Zhou, J., Luo, F., Wen, J., and Li,
X. (2022). A general matrix factorization framework
for recommender systems in multi-access edge com-
puting network. Mobile Networks and Applications,
pages 1–13.
Mobius, A. (2020). Book recommendation dataset.
Mu, R. (2018). A survey of recommender systems based on
deep learning. Ieee Access, 6:69009–69022.
Navarin, N., Van Tran, D., and Sperduti, A. (2020). Learn-
ing kernel-based embeddings in graph neural net-
works. In ECAI 2020, pages 1387–1394. IOS Press.
Neophytou, N., Mitra, B., and Stinson, C. (2022). Re-
visiting popularity and demographic biases in recom-
mender evaluation and effectiveness. In European
Conference on Information Retrieval, pages 641–654.
Springer.
Purificato, E., Boratto, L., and De Luca, E. W. (2022). Do
graph neural networks build fair user models? assess-
ing disparate impact and mistreatment in behavioural
user profiling. In Proceedings of the 31st ACM In-
ternational Conference on Information & Knowledge
Management, pages 4399–4403.
Rahman, T., Surma, B., Backes, M., and Zhang, Y. (2019).
Fairwalk: Towards fair graph embedding.
Rahmattalabi, A., Jabbari, S., Lakkaraju, H., Vayanos, P.,
Izenberg, M., Brown, R., Rice, E., and Tambe, M.
(2021). Fair influence maximization: A welfare opti-
mization approach. In Proceedings of the AAAI Con-
ference on Artificial Intelligence, volume 35, pages
11630–11638.
Sang, L., Xu, M., Qian, S., and Wu, X. (2021). Knowl-
edge graph enhanced neural collaborative filtering
with residual recurrent network. Neurocomputing,
454:417–429.
Sha, X., Sun, Z., and Zhang, J. (2021). Disentangling
multi-facet social relations for recommendation. IEEE
Transactions on Computational Social Systems.
Spinelli, I., Scardapane, S., Hussain, A., and Uncini, A.
(2021). Fairdrop: Biased edge dropout for enhanc-
ing fairness in graph representation learning. IEEE
Transactions on Artificial Intelligence, 3(3):344–354.
Steck, H., Baltrunas, L., Elahi, E., Liang, D., Raimond,
Y., and Basilico, J. (2021). Deep learning for recom-
mender systems: A netflix case study. AI Magazine,
42(3):7–18.
Sun, W., Chang, K., Zhang, L., and Meng, K. (2021). In-
gcf: An improved recommendation algorithm based
on ngcf. In International Conference on Algorithms
and Architectures for Parallel Processing, pages 116–
129. Springer.
Tang, H., Zhao, G., Wu, Y., and Qian, X. (2021).
Multisample-based contrastive loss for top-k recom-
mendation. IEEE Transactions on Multimedia.
Wang, S., Hu, L., Wang, Y., He, X., Sheng, Q. Z., Orgun,
M. A., Cao, L., Ricci, F., and Yu, P. S. (2021).
Graph learning based recommender systems: A re-
view. arXiv preprint arXiv:2105.06339.
Wang, Y., Ma, W., Zhang*, M., Liu, Y., and Ma, S. (2022).
A survey on the fairness of recommender systems.
ACM Journal of the ACM (JACM).
Wang, Y., Ma, W., Zhang, M., Liu, Y., and Ma, S. (2023). A
survey on the fairness of recommender systems. ACM
Transactions on Information Systems, 41(3):1–43.
Wu, L., Chen, L., Shao, P., Hong, R., Wang, X., and Wang,
M. (2021). Learning fair representations for recom-
mendation: A graph-based perspective. In Proceed-
ings of the Web Conference 2021, pages 2198–2208.
Wu, S., Sun, F., Zhang, W., Xie, X., and Cui, B. (2020).
Graph neural networks in recommender systems: a
survey. ACM Computing Surveys (CSUR).
Xue, H.-J., Dai, X., Zhang, J., Huang, S., and Chen, J.
(2017). Deep matrix factorization models for recom-
mender systems. In IJCAI, volume 17, pages 3203–
3209. Melbourne, Australia.
Yang, C. (2022). Supervised contrastive learning for rec-
ommendation. arXiv preprint arXiv:2201.03144.
Yi, B., Shen, X., Liu, H., Zhang, Z., Zhang, W., Liu, S., and
Xiong, N. (2019). Deep matrix factorization with im-
plicit feedback embedding for recommendation sys-
tem. IEEE Transactions on Industrial Informatics,
15(8):4591–4601.
Zhang, Q., Wipf, D., Gan, Q., and Song, L. (2021). A bi-
ased graph neural network sampler with near-optimal
regret. Advances in Neural Information Processing
Systems, 34:8833–8844.
Zhang, Z., Liu, Y., Xu, G., and Luo, G. (2016). Recommen-
dation using dmf-based fine tuning method. Journal
of Intelligent Information Systems, 47(2):233–246.
Zheng, Y. and Wang, D. X. (2022). A survey of rec-
ommender systems with multi-objective optimization.
Neurocomputing, 474:141–153.
Zhou, J., Cui, G., Hu, S., Zhang, Z., Yang, C., Liu, Z.,
Wang, L., Li, C., and Sun, M. (2020). Graph neu-
ral networks: A review of methods and applications.
AI Open, 1:57–81.
WEBIST 2024 - 20th International Conference on Web Information Systems and Technologies
440