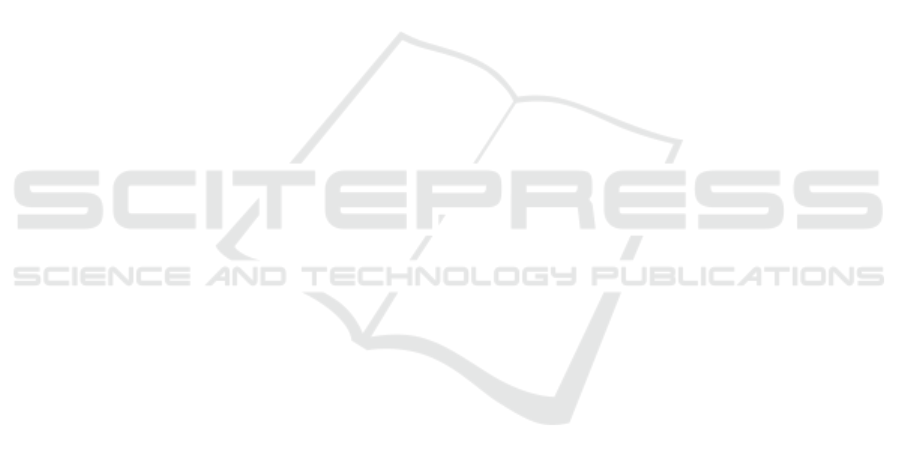
to the CIL model. Nevertheless, the co-existence
based loss in Equation 4 only showed improvement
compared to the basic classification model but failed
to outperform the CIL regression model. MTL ap-
proaches such as MoEs (Jacobs et al., 1991), soft
parameter sharing and sluice networks (Ruder et al.,
2019) showed improvement to the CIL model espe-
cially in new town, but failed to outperform our CIC
model while stitch network (Misra et al., 2016) failed
to learn the driving task, sine wave encoding (Eraqi
et al., 2017) also showed slight improvement com-
pared to the CIL model.
5 CONCLUSION
In this work, we propose two contributions to the end-
to-end steering problem tackled by the conditional
imitation learning (CIL) model, the CIL model suf-
fered from lack of generalization and poor perfor-
mance when tested in unseen environment, the first
contribution of this work is conditional imitation co-
learning (CIC), the introduced approach proposes a
modified network architecture that allows the special-
ist branches in the CIL model to co-learn to overcome
the generalization issue and increase the model’s ro-
bustness in unseen environment, the other contribu-
tion is posing the steering regression problem as clas-
sification by using a combination of CCE and MSE
losses. The CIC model showed a significant improve-
ment to performance in unseen environment by 62%
while posing regression as classification showed only
improvement by 21%.
REFERENCES
Bekey, G. A. (2005). Autonomous robots: from biological
inspiration to implementation and control. The MIT
Press.
Bengio, S., Dean, J., Erhan, D., Ie, E., Le, Q., Rabinovich,
A., Shlens, J., and Singer, Y. (2013). Using web co-
occurrence statistics for improving image categoriza-
tion. ArXiv preprint, arXiv:1312.5697.
Bewley, A., Rigley, J., Liu, Y., Hawke, J., Shen, R., Lam,
V.-D., and Kendall, A. (2019). Learning to drive from
simulation without real world labels. In 2019 In-
ternational Conference on Robotics and Automation
(ICRA), pages 4817–4823. IEEE.
Bojarski, M., Testa, D. D., Dworakowski, D., Firner,
B., Flepp, B., Goyal, P., Jackel, L. D., Monfort,
M., Muller, U., Zhang, J., et al. (2016). End to
end learning for self-driving cars. arXiv preprint,
arXiv:1604.07316.
Codevilla, F., Miiller, M., Lopez, A., Koltun, V., and Doso-
vitskiy, A. (2018). End-to-end driving via conditional
imitation learning. In Proceedings of the 2018 IEEE
International Conference on Robotics and Automation
(ICRA), pages 1–9.
Cui, J., Qiu, H., Chen, D., Stone, P., and Zhu, Y. (2022).
Coopernaut: End-to-end driving with cooperative per-
ception for networked vehicles. In Proceedings of the
IEEE/CVF Conference on Computer Vision and Pat-
tern Recognition (CVPR), pages 17252–17262.
Dammen, J. (2019). End-to-end deep learning for au-
tonomous driving. Master’s thesis, Norwegian Uni-
versity of Science and Technology.
Dauphin, Y. N., Fan, A., Auli, M., and Grangier, D.
(2017). Language modeling with gated convolutional
networks. In Proceedings of the 34th International
Conference on Machine Learning (ICML), pages 933–
941. PMLR.
Dosovitskiy, A., Ros, G., Codevilla, F., L
´
opez, A., and
Koltun, V. (2017). Carla: An open urban driving sim-
ulator. In Proceedings of the Conference on Robot
Learning (CoRL).
Eraqi, H., Moustafa, M., and Honer, J. (2017). End-
to-end deep learning for steering autonomous ve-
hicles considering temporal dependencies. CoRR,
abs/1710.03804.
Eraqi, H. M., Moustafa, M. N., and Honer, J. (2022). Dy-
namic conditional imitation learning for autonomous
driving. IEEE Transactions on Intelligent Transporta-
tion Systems.
Hawke, J., Shen, R., Gurau, C., Sharma, S., Reda, D.,
Nikolov, N., Mazur, P., Micklethwaite, S., Grif-
fiths, N., Shah, A., et al. (2019). Urban driving
with conditional imitation learning. arXiv preprint,
arXiv:1912.00177.
Huber, P. J. (1992). Robust estimation of a location param-
eter. In Breakthroughs in statistics, pages 492–518.
Springer, New York.
Jacobs, R. A., Jordan, M. I., Nowlan, S. J., and Hinton, G. E.
(1991). Adaptive mixtures of local experts. Neural
Computation, 3(1):79–87.
Kingma, D. and Ba, J. (2014). Adam: A method
for stochastic optimization. arXiv preprint,
arXiv:1412.6980.
Kourbane, I. and Genc, Y. (2021). A hybrid classification-
regression approach for 3d hand pose estimation us-
ing graph convolutional networks. arXiv preprint,
arXiv:2105.10902.
Liang, X., Wang, T., Yang, L., and Xing, E. (2018).
Cirl: Controllable imitative reinforcement learning for
vision-based self-driving. In Proceedings of the Euro-
pean Conference on Computer Vision (ECCV), pages
584–599.
Misra, I., Shrivastava, A., Gupta, A., and Hebert, M. (2016).
Cross-stitch networks for multi-task learning. In Pro-
ceedings of the IEEE Conference on Computer Vision
and Pattern Recognition (CVPR), pages 3994–4003.
Moustafa, M. N. (2005). System and method for pose-angle
estimation.
Muller, M., Dosovitskiy, A., Ghanem, B., and Koltun, V.
(2018). Driving policy transfer via modularity and ab-
End-to-End Steering for Autonomous Vehicles via Conditional Imitation Co-Learning
635