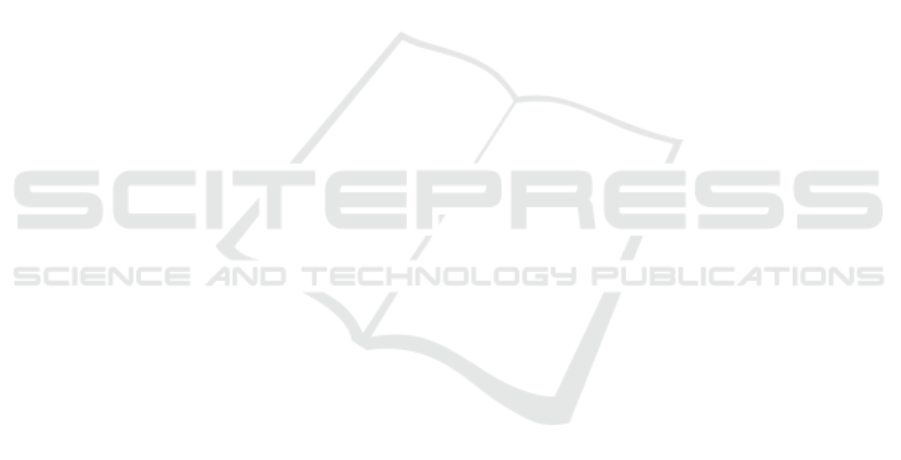
vre, E. (2008a). Fast unfolding of communities in
large networks. Journal of Statistical Mechanics:
Theory and Experiment, 2008(10):P10008.
Blondel, V. D., Guillaume, J.-L., Lambiotte, R., and
Lefebvre, E. (2008b). Louvain algorithm. Jour-
nal of Statistical Mechanics: Theory and Experiment,
2008(10):P10008.
Cai, B., Wang, Y., Zeng, L., Hu, Y., and Li, H. (2020).
Edge classification based on convolutional neural net-
works for community detection in complex network.
Physica A: statistical mechanics and its applications,
556:124826.
Cavallari, S., Cambria, E., Cai, H., Chang, K. C.-C., and
Zheng, V. W. (2019). Embedding both finite and infi-
nite communities on graphs [application notes]. IEEE
computational intelligence magazine, 14(3):39–50.
Cavallari, S., Zheng, V. W., Cai, H., Chang, K. C.-C., and
Cambria, E. (2017). Learning community embed-
ding with community detection and node embedding
on graphs. In Proceedings of the 2017 ACM on Con-
ference on Information and Knowledge Management,
pages 377–386.
Dalleau, K., Couceiro, M., and Smail-Tabbone, M. (2020a).
Computing vertex-vertex dissimilarities using random
trees: Application to clustering in graphs. In Ad-
vances in Intelligent Data Analysis XVIII, pages 132–
144, Berlin, Heidelberg. Springer-Verlag.
Dalleau, K., Couceiro, M., and Smail-Tabbone, M. (2020b).
Computing vertex-vertex dissimilarities using random
trees: Application to clustering in graphs. In Advances
in Intelligent Data Analysis XVIII, pages 132–144.
Girvan, M. and Newman, M. E. (2002). Community struc-
ture in social and biological networks. Proceedings of
the national academy of sciences, 99(12):7821–7826.
Gong, M., Liu, J., Ma, L., Cai, Q., and Jiao, L. (2014).
Novel heuristic density-based method for community
detection in networks. Physica A: Statistical Mechan-
ics and its Applications, 403:71–84.
Grover, A. and Leskovec, J. (2016). node2vec: Scal-
able feature learning for networks. In Proceedings
of the 22nd ACM SIGKDD international conference
on Knowledge discovery and data mining, pages 855–
864.
Guo, W.-F. and Zhang, S.-W. (2016). A general method of
community detection by identifying community cen-
ters with affinity propagation. Physica A: Statistical
Mechanics and its Applications, 447:508–519.
He, D., You, X., Feng, Z., Jin, D., Yang, X., and Zhang,
W. (2018). A network-specific markov random field
approach to community detection. In Proceedings of
the AAAI Conference on Artificial Intelligence, vol-
ume 32.
Jin, D., Liu, Z., Li, W., He, D., and Zhang, W. (2019a).
Graph convolutional networks meet markov random
fields: Semi-supervised community detection in at-
tribute networks. In Proceedings of the AAAI confer-
ence on artificial intelligence, volume 33, pages 152–
159.
Jin, D., You, X., Li, W., He, D., Cui, P., Fogelman-
Souli
´
e, F., and Chakraborty, T. (2019b). Incorpo-
rating network embedding into markov random field
for better community detection. In Proceedings of
the AAAI Conference on Artificial Intelligence, vol-
ume 33, pages 160–167.
Jin, D., Zhang, B., Song, Y., He, D., Feng, Z., Chen, S., Li,
W., and Musial, K. (2020). Modmrf: A modularity-
based markov random field method for community de-
tection. Neurocomputing, 405:218–228.
Karatas¸, A. and S¸ahin, S. (2018). Application areas of com-
munity detection: A review. In 2018 International
congress on big data, deep learning and fighting cy-
ber terrorism (IBIGDELFT), pages 65–70. IEEE.
Karrer, B. and Newman, M. E. (2011). Stochastic block-
models and community structure in networks. Physi-
cal review E, 83(1):016107.
Kirkpatrick, S., Gelatt Jr, C. D., and Vecchi, M. P.
(1983). Optimization by simulated annealing. science,
220(4598):671–680.
Leskovec, J., Kleinberg, J., and Faloutsos, C. (2007).
Graph evolution: Densification and shrinking diame-
ters. ACM transactions on Knowledge Discovery from
Data (TKDD), 1(1):2–es.
Lusseau, D., Schneider, K., Boisseau, O. J., Haase, P.,
Slooten, E., and Dawson, S. M. (2003). The bot-
tlenose dolphin community of doubtful sound features
a large proportion of long-lasting associations. Behav-
ioral Ecology and Sociobiology, 54(4):396–405.
Mikolov, T., Chen, K., Corrado, G., and Dean, J. (2013).
Efficient estimation of word representations in vector
space. arXiv preprint arXiv:1301.3781.
Paim, E. C., Bazzan, A. L. C., and Chira, C. (2020). De-
tecting communities in networks: a decentralized ap-
proach based on multiagent reinforcement learning. In
2020 IEEE Symposium Series on Computational Intel-
ligence (SSCI), pages 2225–2232.
Perozzi, B., Al-Rfou, R., and Skiena, S. (2014). Deepwalk:
Online learning of social representations. In Proceed-
ings of the 20th ACM SIGKDD international confer-
ence on Knowledge discovery and data mining, pages
701–710.
Rosvall, M. and Bergstrom, C. T. (2012). Infomap algo-
rithm. Physical Review E, 86(1):016108.
Rozemberczki, B., Davies, R., Sarkar, R., and Sutton, C.
(2019). Gemsec: Graph embedding with self clus-
tering. In Proceedings of the 2019 IEEE/ACM inter-
national conference on advances in social networks
analysis and mining, pages 65–72.
Shi, X., Lu, H., He, Y., and He, S. (2015). Community de-
tection in social network with pairwisely constrained
symmetric non-negative matrix factorization. In Pro-
ceedings of the 2015 IEEE/ACM International Con-
ference on Advances in Social Networks Analysis and
Mining 2015, pages 541–546.
Sui, S.-K., Li, J.-P., Zhang, J.-G., and Sui, S.-J. (2016).
The community detection based on SVM algorithm.
In 2016 13th International Computer Conference on
Wavelet Active Media Technology and Information
Processing (ICCWAMTIP).
Taheri, S. and Bouyer, A. (2020a). Community detection in
SPACED: A Novel Deep Learning Method for Community Detection in Social Networks
151