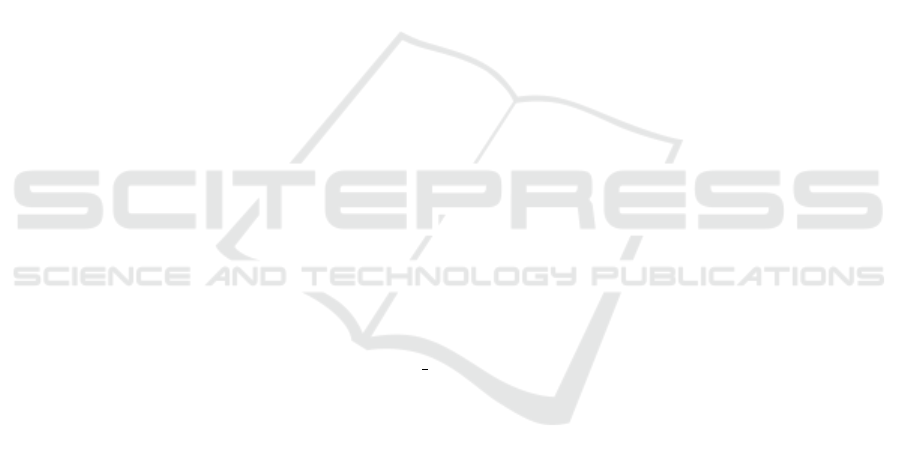
REFERENCES
Chen, J., Lin, H., Han, X., and Sun, L. (2024). Benchmark-
ing large language models in retrieval-augmented gen-
eration. In Proceedings of the AAAI Conference on Ar-
tificial Intelligence, volume 38, pages 17754–17762.
Fan, W., Ding, Y., Ning, L., Wang, S., Li, H., Yin, D.,
Chua, T.-S., and Li, Q. (2024). A survey on rag meet-
ing llms: Towards retrieval-augmented large language
models. In Proceedings of the 30th ACM SIGKDD
Conference on Knowledge Discovery and Data Min-
ing, pages 6491–6501.
Feng, Z., Feng, X., Zhao, D., Yang, M., and Qin, B.
(2024). Retrieval-generation synergy augmented large
language models. In ICASSP 2024-2024 IEEE Inter-
national Conference on Acoustics, Speech and Signal
Processing (ICASSP), pages 11661–11665. IEEE.
Gao, D., Wang, H., Li, Y., Sun, X., Qian, Y., Ding, B.,
and Zhou, J. (2023a). Text-to-sql empowered by large
language models: A benchmark evaluation. arXiv
preprint arXiv:2308.15363.
Gao, Y., Xiong, Y., Gao, X., Jia, K., Pan, J., Bi, Y., Dai, Y.,
Sun, J., and Wang, H. (2023b). Retrieval-augmented
generation for large language models: A survey. arXiv
preprint arXiv:2312.10997.
Giray, L. (2023). Prompt engineering with chatgpt: a guide
for academic writers. Annals of biomedical engineer-
ing, 51(12):2629–2633.
Jeong, C. (2023). A study on the implementation
of generative ai services using an enterprise data-
based llm application architecture. arXiv preprint
arXiv:2309.01105.
Jin, H., Huang, L., Cai, H., Yan, J., Li, B., and Chen, H.
(2024). From llms to llm-based agents for software
engineering: A survey of current, challenges and fu-
ture. arXiv preprint arXiv:2408.02479.
Langchain (2024). Langchain agents documenta-
tion. https://python.langchain.com/v0.1/docs/use
cases/sql/agents/. Accessed: 2024-09-06.
Lewis, P., Perez, E., Piktus, A., Petroni, F., Karpukhin,
V., Goyal, N., K
¨
uttler, H., Lewis, M., Yih, W.-t.,
Rockt
¨
aschel, T., et al. (2020). Retrieval-augmented
generation for knowledge-intensive nlp tasks. Ad-
vances in Neural Information Processing Systems,
33:9459–9474.
Li, H., Su, Y., Cai, D., Wang, Y., and Liu, L. (2022). A
survey on retrieval-augmented text generation. arXiv
preprint arXiv:2202.01110.
Liu, A., Hu, X., Wen, L., and Yu, P. S. (2023). A com-
prehensive evaluation of chatgpt’s zero-shot text-to-
sql capability. arXiv preprint arXiv:2303.13547.
Mialon, G., Dess
`
ı, R., Lomeli, M., Nalmpantis, C., Pa-
sunuru, R., Raileanu, R., Rozi
`
ere, B., Schick, T.,
Dwivedi-Yu, J., Celikyilmaz, A., et al. (2023). Aug-
mented language models: a survey. arXiv preprint
arXiv:2302.07842.
OpenAI (2023a). Chatgpt fine-tune descrip-
tion. https://help.openai.com/en/articles/
6783457-what-is-chatgpt. Accessed: 2024-03-
01.
OpenAI (2023b). Chatgpt prompt engineer-
ing. https://platform.openai.com/docs/guides/
prompt-engineering. Accessed: 2024-04-01.
Pinheiro, J., Victorio, W., Nascimento, E., Seabra, A.,
Izquierdo, Y., Garcıa, G., Coelho, G., Lemos, M.,
Leme, L. A. P. P., Furtado, A., et al. (2023). On the
construction of database interfaces based on large lan-
guage models. In Proceedings of the 19th Interna-
tional Conference on Web Information Systems and
Technologies - Volume 1: WEBIST, pages 373–380.
INSTICC, SciTePress.
Saeed, M., De Cao, N., and Papotti, P. (2023). Query-
ing large language models with sql. arXiv preprint
arXiv:2304.00472.
Seabra, A., Nepomuceno, J., Lago, L., Ruberg, N., and
Lifschitz, S. (2024). Contrato360: uma aplicac¸
˜
ao de
perguntas e respostas usando modelos de linguagem,
documentos e bancos de dados. In Anais do XXXIX
Simp
´
osio Brasileiro de Bancos de Dados.
Singh, A., Ehtesham, A., Kumar, S., and Khoei, T. T.
(2024). Enhancing ai systems with agentic workflows
patterns in large language model. In 2024 IEEE World
AI IoT Congress (AIIoT), pages 527–532. IEEE.
Vaswani, A., Shazeer, N., Parmar, N., Uszkoreit, J., Jones,
L., Gomez, A. N., Kaiser, Ł., and Polosukhin, I.
(2017). Attention is all you need. Advances in neural
information processing systems, 30.
Wang, M., Wang, M., Xu, X., Yang, L., Cai, D., and Yin,
M. (2023). Unleashing chatgpt’s power: A case study
on optimizing information retrieval in flipped class-
rooms via prompt engineering. IEEE Transactions on
Learning Technologies.
Wang, Z., Wang, Z., Le, L., Zheng, H. S., Mishra, S., Perot,
V., Zhang, Y., Mattapalli, A., Taly, A., Shang, J., et al.
(2024). Speculative rag: Enhancing retrieval aug-
mented generation through drafting. arXiv preprint
arXiv:2407.08223.
White, J., Fu, Q., Hays, S., Sandborn, M., Olea, C., Gilbert,
H., Elnashar, A., Spencer-Smith, J., and Schmidt,
D. C. (2023). A prompt pattern catalog to enhance
prompt engineering with chatgpt. arXiv preprint
arXiv:2302.11382.
Yao, S., Zhao, J., Yu, D., Du, N., Shafran, I., Narasimhan,
K., and Cao, Y. (2023). React: Synergizing rea-
soning and actin in langage models. arXiv preprint
arXiv:2210.03629v3.
KDIR 2024 - 16th International Conference on Knowledge Discovery and Information Retrieval
178