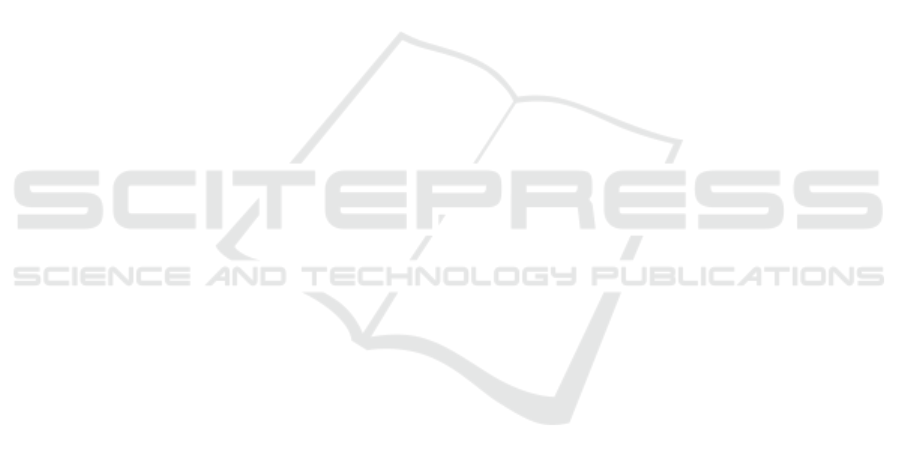
on developing critical thinking and problem-solving
skills yielded a 25% increase in these areas. The
adaptive feedback mechanisms and real-time task
adjustments fostered deeper cognitive engagement,
much like similar systems explored by (Awais et al.,
2019).By emphasizing the learning process,
IntelliFrame ensures that students reflect on their
approaches, explore alternatives, and refine their
work iteratively. Student engagement was another
area of significant improvement, with a 35% increase
compared to traditional methods. The adaptive
learning pathways and personalized feedback helped
sustain motivation, similar to findings by (Hadyaoui
& Cheniti-Belcadhi, 2023). IntelliFrame's real-time
support kept students engaged throughout the course,
preventing disengagement that often occurs with
static assessments.
The broader implications of IntelliFrame suggest
a shift toward more personalized, process-oriented
assessments in education. As highlighted by (Xu,
2024), AI's role in tailoring assessments to individual
needs can close learning gaps and promote more
inclusive practices. The system's continuous feedback
model offers educators real-time insights into student
progress. However, challenges remain. The
complexity of developing domain-specific ontologies
limits scalability. Additionally, concerns about over-
reliance on AI and data privacy, raised by (Smolansky
et al., 2023), must be addressed to ensure ethical use
of AI in education. Future work should focus on
refining IntelliFrame's scalability and exploring its
application across other disciplines, as well as
enhancing personalization algorithms and exploring
long-term impacts on student success.
REFERENCES
Awais, M., Habiba, U., Khalid, H., Shoaib, M., & Arshad,
S. (2019). An Adaptive Feedback System to Improve
Student Performance Based on Collaborative Behavior.
IEEE Access, PP, 1.
https://doi.org/10.1109/ACCESS.2019.2931565
Contrino, M. F., Reyes-Millán, M., Vázquez-Villegas, P.,
& Membrillo-Hernández, J. (2024). Using an adaptive
learning tool to improve student performance and
satisfaction in online and face-to-face education for a
more personalized approach. Smart Learning
Environments, 11(1), 6. https://doi.org/10.1186/
s40561-024-00292-y
Feuerriegel, S., Hartmann, J., Janiesch, C., & Zschech, P.
(2024). Generative AI. Business and Information
Systems Engineering, 66(1), 111–126.
https://doi.org/10.1007/s12599-023-00834-7
Hadyaoui, A., & Cheniti-belcadhi, L. (2022). Towards an
Adaptive Intelligent Assessment Framework for
Collaborative Learning. 1(Csedu), 601–608.
https://doi.org/10.5220/0011124400003182
Hadyaoui, A., & Cheniti-Belcadhi, L. (2022). Towards an
Ontology-based Recommender System for Assessment
in a Collaborative Elearning Environment. Webist,
294–301. https://doi.org/10.5220/0011543500003318
Hadyaoui, A., & Cheniti-Belcadhi, L. (2023). An Ontology-
Based Collaborative Assessment Analytics Framework
to Predict Groups’ Disengagement BT - Intelligent
Decision Technologies (I. Czarnowski, R. J. Howlett, &
L. C. Jain, Eds.; pp. 74–84). Springer Nature Singapore.
Long, H., Kerr, B., Emler, T., & Birdnow, M. (2022). A
Critical Review of Assessments of Creativity in
Education. Review of Research in Education, 46, 288–
323. https://doi.org/10.3102/0091732X221084326
Matthews, K., Janicki, T., He, L., & Patterson, L. (2012).
Implementation of an Automated Grading System with
an Adaptive Learning Component to Affect Student
Feedback and Response Time.
Menucha Birenbaum, & Filip Dochy. (1996). Alternatives
in Assessment of Achievements, Learning Processes
and Prior Knowledge. In Alternatives in Assessment of
Achievements, Learning Processes and Prior
Knowledge. Springer Netherlands.
https://doi.org/10.1007/978-94-011-0657-3
Mizumoto, A., & Eguchi, M. (2023). Exploring the
potential of using an AI language model for automated
essay scoring. Research Methods in Applied
Linguistics, 2(2), 100050. https://doi.org/https://doi.org
/10.1016/j.rmal.2023.100050
Nguyen, T., Tran, H., & Nguyen, M. (2023). Empowering
Education: Exploring the Potential of Artificial
Intelligence; Chapter 9 -Artificial Intelligence (AI) in
Teaching and Learning: A Comprehensive Review.
Paiva, J., Leal, J., & Figueira, Á. (2022). Automated
Assessment in Computer Science Education: A State-
of-the-Art Review. ACM Transactions on Computing
Education, 22. https://doi.org/10.1145/3513140
Pellegrino, J., Chudowsky, N., & Glaser, R. (2001).
Knowing What Students Know: The Science and Design
of Educational Assessment.
Smolansky, A., Cram, A., Raduescu, C., Zeivots, S., Huber,
E., & Kizilcec, R. F. (2023). Educator and Student
Perspectives on the Impact of Generative AI on
Assessments in Higher Education. Proceedings of the
Tenth ACM Conference on Learning @ Scale, 378–
382. https://doi.org/10.1145/3573051.3596191
Vanlehn, K., Banerjee, C., Milner, F., & Wetzel, J. (2020).
Teaching algebraic model construction: A tutoring
system, lessons learned and an evaluation 1
Introduction. 1–22.
Xu, Z. (2024). AI in education: Enhancing learning
experiences and student outcomes. Applied and
Computational Engineering, 51, 104–111.
https://doi.org/10.54254/2755-2721/51/20241187
WEBIST 2024 - 20th International Conference on Web Information Systems and Technologies
448