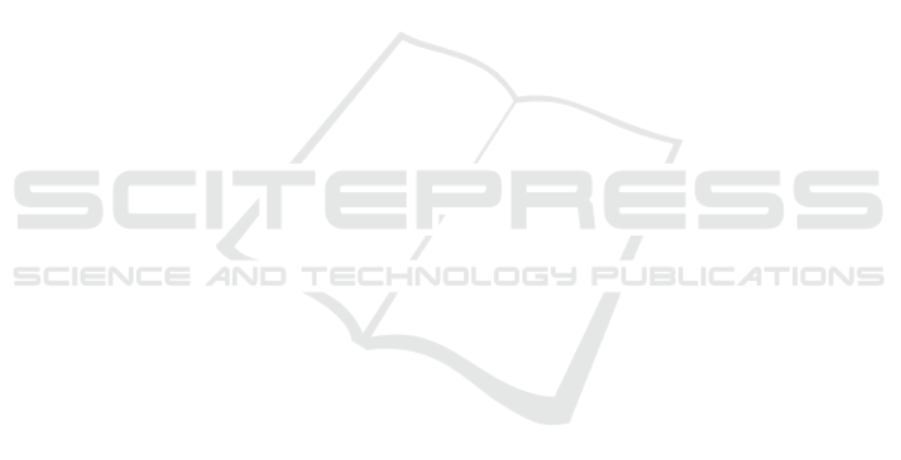
ChatGPT: ChatGPT’s ontology requires more
manual intervention. While it can generate a basic
structure, the lack of logical constraints and reason-
ing capabilities means that significant human effort is
required to refine and complete the ontology.
Expert: The expert ontology requires the most
human effort, as it is manually created by a domain
expert. This results in high accuracy and complete-
ness but is time-consuming and requires specialized
knowledge.
In this evaluation, the term ”expert” refers specif-
ically to an expert in Semantic Web technologies and
ontology engineering, rather than a domain expert in
the field of fitness or physical training. This distinc-
tion is important when considering the complexity
and richness of the expert-generated ontology. It is
also critical to note that the techniques applied in this
evaluation are not limited to fitness but are broadly
applicable to any domain requiring ontology-driven
knowledge representation and reasoning.
5 DISCUSSION
The evaluation of the proposed approach to ontol-
ogy generation reveals several strengths, particularly
in terms of completeness, consistency, and correct-
ness, achieved with minimal human intervention. By
automating the extraction of structured knowledge
from unstructured text, our system significantly re-
duces the manual effort typically required in ontol-
ogy creation. While the expert-generated ontology
demonstrates superior richness and advanced reason-
ing capabilities, it requires extensive human effort and
domain expertise. This labor-intensive process is of-
ten not feasible for dynamic applications, where rapid
ontology updates are necessary. In contrast, our ap-
proach effectively balances automation and accuracy,
allowing domain specialists who may lack deep ex-
pertise in Semantic Web technologies to contribute to
ontology development. The comparison with Chat-
GPT underscores the added value of our system. Al-
though ChatGPT can identify key concepts, it lacks
the intricate reasoning mechanisms and formal logical
structures required for a fully coherent and logically
sound ontology. The necessity for significant manual
refinement with ChatGPT limits its usability in com-
plex domains. Our system introduces domain-specific
logical constraints and consistency checks, which en-
hance the quality of the generated ontologies and re-
duce reliance on manual post-processing.
However, these observations are only an initial as-
sessment of the results of our approach. As the evalu-
ation is based on a single text source and not various
corpora, it would be necessary to broaden and deepen
the evaluation of our approach on several text sources
representing various domains in order to further val-
idate and refine its performance. Therefore, our fu-
ture work will aim to improve the experimental results
by including quantitative measures, such as precision,
recall and F1 scores, as well as qualitative analyses
to support our conclusions. This dual approach will
provide a better understanding of the performance of
our system compared with existing methods, includ-
ing other ontology generation systems. In addition,
these initial results have enabled us to identify ar-
eas for improvement in future research, such as as-
sertion enrichment, SWRL rule creation, and the sys-
tem’s ability to handle complex, multi-layered con-
straints. With these advances, we aim to increase the
practical applicability and efficiency of our approach,
ultimately contributing to the broader landscape of
knowledge management and representation.
REFERENCES
Al-Aswadi, F. N., Chan, H. Y., and Gan, K. H. (2020).
Automatic ontology construction from text: a review
from shallow to deep learning trend. Artificial Intelli-
gence Review, 53(6):3901–3928.
Antoniou, G. and Harmelen, F. v. (2009). Web ontology
language: Owl. Handbook on ontologies, pages 91–
110.
Asim, M. N., Wasim, M., Khan, M. U. G., Mahmood,
W., and Abbasi, H. M. (2018). A survey of ontol-
ogy learning techniques and applications. Database,
2018:bay101.
Baktash, J. A. and Dawodi, M. (2023). Gpt-4: A review on
advancements and opportunities in natural language
processing. arXiv preprint arXiv:2305.03195.
Brown, T., Mann, B., Ryder, N., Subbiah, M., Kaplan, J. D.,
Dhariwal, P., Neelakantan, A., Shyam, P., Sastry, G.,
Askell, A., et al. (2020). Language models are few-
shot learners. Advances in neural information pro-
cessing systems, 33:1877–1901.
Chen, S., Zhang, Y., and Yang, Q. (2024). Multi-task
learning in natural language processing: An overview.
ACM Computing Surveys, 56(12):1–32.
Cimiano, P. and V
¨
olker, J. (2005). Text2onto: A framework
for ontology learning and data-driven change discov-
ery. In International conference on application of nat-
ural language to information systems, pages 227–238.
Springer.
Etzioni, O., Banko, M., Soderland, S., and Weld, D. S.
(2011). Open information extraction: The second gen-
eration. In Proceedings of the Twenty-Second Inter-
national Joint Conference on Artificial Intelligence,
pages 3–10.
Fensel, D. and Fensel, D. (2001). Ontologies. Springer.
WEBIST 2024 - 20th International Conference on Web Information Systems and Technologies
464