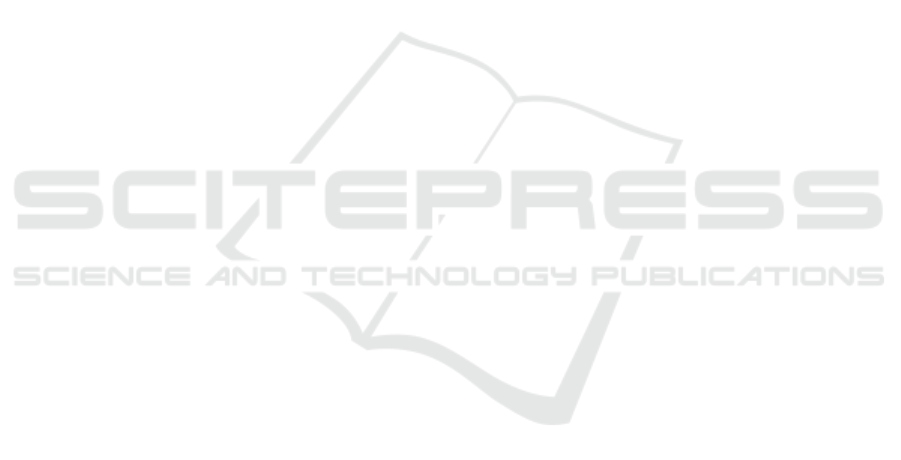
rithms in a System Supporting Money Laundering De-
tection. Information Sciences, 295:18–32.
Grover, A. and Leskovec, J. (2016). node2vec: Scalable
Feature Learning for Networks. In Proceedings of
the 22nd ACM SIGKDD International Conference on
Knowledge Discovery and Data Mining, pages 855–
864.
Guo, C., Zhang, S., Zhang, P., Alkubati, M., and Song, J.
(2023). Lb-glat: Long-term bi-graph layer attention
convolutional network for anti-money laundering in
transactional blockchain. Mathematics.
Husain, O. (2024). 13 biggest aml fines ($500 million plus).
Accessed: 2024-09-05.
Jayasree, V. and Balan, R. S. (2017). Money Launder-
ing Regulatory Risk Evaluation Using Bitmap Index-
Based Decision Tree. Journal of the Association of
Arab Universities for Basic and Applied Sciences,
23:96–102.
Jensen, R. I. T., Ferwerda, J., Jørgensen, K. S., Jensen,
E. R., Borg, M., Krogh, M. P., Jensen, J. B., and Iosi-
fidis, A. (2023). A Synthetic Data Set to Benchmark
Anti-Money Laundering Methods. Scientific Data,
10(1):661. Publisher: Nature Publishing Group UK
London.
Jullum, M., Løland, A., Huseby, R. B.,
˚
Anonsen, G., and
Lorentzen, J. (2020). Detecting Money Launder-
ing Transactions with Machine Learning. Journal of
Money Laundering Control, 23(1):173–186.
Jurgovsky, J., Granitzer, M., Ziegler, K., Calabretto, S.,
Portier, P.-E., He-Guelton, L., and Caelen, O. (2018).
Sequence classification for credit-card fraud detec-
tion. Expert Systems with Applications, 100:234–245.
Ketenci, U. G., Kurt, T.,
¨
Onal, S., Erbil, C., Akt
¨
urko
ˇ
glu,
S., and
˙
Ilhan, H. S¸. (2021). A Time-Frequency Based
Suspicious Activity Detection for Anti-Money Laun-
dering. IEEE Access, 9:59957–59967.
Keyan, L. and Tingting, Y. (2011). An Improved Support-
Vector Network Model for Anti-Money Laundering.
In 2011 Fifth International Conference on Manage-
ment of e-Commerce and e-Government, pages 193–
196. IEEE.
Kipf, T. N. and Welling, M. (2016). Semi-Supervised Clas-
sification with Graph Convolutional Networks. arXiv
preprint arXiv:1609.02907.
Kumar, A., Das, S., and Tyagi, V. (2020). Anti Money
Laundering Detection Using Na
¨
ıve Bayes Classifier.
In 2020 IEEE International Conference on Comput-
ing, Power and Communication Technologies (GU-
CON), pages 568–572. IEEE.
Kumar, A., Ghosh, S., and Verma, J. (2022). Guided Self-
Training Based Semi-Supervised Learning for Fraud
Detection. In Proceedings of the Third ACM Interna-
tional Conference on AI in Finance, pages 148–155,
New York, NY, USA. ACM.
Kute, D. V., Pradhan, B., Shukla, N., and Alamri, A.
(2021). Deep Learning and Explainable Artifi-
cial Intelligence Techniques Applied for Detecting
Money Laundering–A Critical Review. IEEE Access,
9:82300–82317.
Labib, N. M., Rizka, M. A., and Shokry, A. E. M. (2020).
Survey of machine learning approaches of anti-money
laundering techniques to counter terrorism finance. In
Ghalwash, A. Z., El Khameesy, N., Magdi, D. A., and
Joshi, A., editors, Internet of Things—Applications
and Future, pages 73–87, Singapore. Springer Singa-
pore.
Liu, X. and Zhang, P. (2010). A Scan Statistics Based
Suspicious Transactions Detection Model for Anti-
Money Laundering (AML) in Financial Institutions.
In 2010 International Conference on Multimedia
Communications, pages 210–213. IEEE.
Lopez-Rojas, E., Elmir, A., and Axelsson, S. (2016).
PaySim: A Financial Mobile Money Simulator for
Fraud Detection. In 28th European Modeling and
Simulation Symposium, EMSS, Larnaca, pages 249–
255. Dime University of Genoa.
Lopez-Rojas, E. A. and Axelsson, S. (2012). Multi-Agent
Based Simulation (MABS) of Financial Transactions
for Anti-Money Laundering (AML). In Nordic Con-
ference on Secure IT Systems. Blekinge Institute of
Technology.
Mani, I. and Zhang, I. (2003). knn approach to unbalanced
data distributions: A case study involving information
extraction. In Proceedings of the ICML 2003 Work-
shop on Learning from Imbalanced Data Sets, pages
1–7.
Marasi, S. and Ferretti, S. (2024). Anti-money laundering
in cryptocurrencies through graph neural networks: A
comparative study. Proceedings Article.
Mikolov, T., Sutskever, I., Chen, K., Corrado, G. S., and
Dean, J. (2013). Distributed Representations of Words
and Phrases and Their Compositionality. Advances in
Neural Information Processing Systems, 26.
Ning, P., Wang, P., and Zhang, Z. (2024). An anti-
money laundering method based on spatio-temporal
graph convolutional networks. Advances in Transdis-
ciplinary Engineering.
Oztas, B., Cetinkaya, D., Adedoyin, F., Budka, M., Do-
gan, H., and Aksu, G. (2023). Enhancing Anti-Money
Laundering: Development of a Synthetic Transaction
Monitoring Dataset. In 2023 IEEE International Con-
ference on e-Business Engineering (ICEBE), pages
47–54. IEEE.
Palamuttam, R. and Chen, W. (2017). Evaluating Net-
work Embeddings: Node2Vec vs Spectral Clustering
vs GCN.
Pambudi, B. N., Hidayah, I., and Fauziati, S. (2019). Im-
proving Money Laundering Detection Using Opti-
mized Support Vector Machine. In 2019 International
Seminar on Research of Information Technology and
Intelligent Systems (ISRITI), pages 273–278. IEEE.
Pandey, A., Bhatraju, A., Markam, S., and Bhatt, D. (2022).
Adversarial Fraud Generation for Improved Detec-
tion. In Proceedings of the Third ACM International
Conference on AI in Finance, pages 123–129, New
York, NY, USA. ACM.
Paula, E. L., Ladeira, M., Carvalho, R. N., and Marza-
gao, T. (2016). Deep Learning Anomaly Detection as
Support Fraud Investigation in Brazilian Exports and
NCTA 2024 - 16th International Conference on Neural Computation Theory and Applications
506