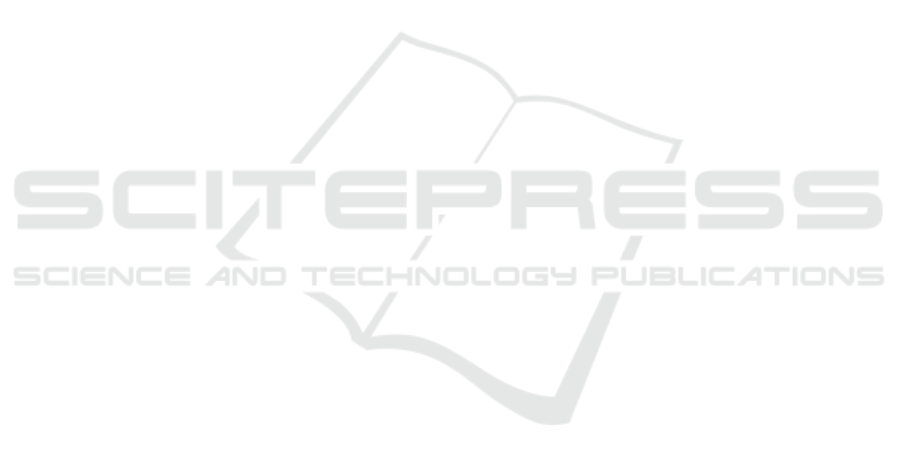
Baker, M. R. (2022). Ensemble learning with supervised
machine learning models to predict credit card fraud
transactions.
Bakhtiari, S., Nasiri, Z., and Vahidi, J. (2023). Credit card
fraud detection using ensemble data mining methods.
Multimedia Tools and Applications, 82(19):29057–
29075.
Carcillo, F., Dal Pozzolo, A., Le Borgne, Y.-A., Caelen, O.,
Mazzer, Y., and Bontempi, G. (2018). Scarff: a scal-
able framework for streaming credit card fraud detec-
tion with spark. Information fusion, 41:182–194.
Cheon, M.-j., Lee, D., Joo, H. S., and Lee, O. (2021). Deep
learning based hybrid approach of detecting fraudu-
lent transactions. Journal of Theoretical and Applied
Information Technology, 99(16):4044–4054.
de Zarz
`
a, I., de Curt
`
o, J., and Calafate, C. T. (2023). Op-
timizing neural networks for imbalanced data. Elec-
tronics, 12(12):2674.
El baida, M., Hosni, M., Boushaba, F., Chourak, M., et al.
(2024). A systematic literature review on classifica-
tion machine learning for urban flood hazard mapping.
Water Resources Management, pages 1–42.
Grossi, M., Ibrahim, N., Radescu, V., Loredo, R., Voigt,
K., and Altrock, C. V. O. N. (2022). Mixed quan-
tum – classical method for fraud detection with quan-
tum feature selection. IEEE Trans. Quantum Eng.,
3(October):1–12.
Hosni, M., Carrillo-de Gea, J. M., Idri, A., Fern
´
andez-
Alem
´
an, J. L., and Garc
´
ıa-Bern
´
a, J. A. (2019). Using
ensemble classification methods in lung cancer dis-
ease. In 2019 41st Annual International Conference
of the IEEE Engineering in Medicine and Biology So-
ciety (EMBC), pages 1367–1370. IEEE.
Hosni, M. and Idri, A. (2018). Software development effort
estimation using feature selection techniques. In New
trends in intelligent software methodologies, tools and
techniques, pages 439–452. IOS Press.
Kim, E. et al. (2019). Champion-challenger analysis for
credit card fraud detection: Hybrid ensemble and deep
learning. Expert Syst. Appl., 128:214–224.
Leevy, J. L., Johnson, J. M., Hancock, J., and Khoshgoftaar,
T. M. (2023). Threshold optimization and random un-
dersampling for imbalanced credit card data. J. Big
Data.
Li, C., Ding, N., Zhai, Y., and Dong, H. (2021a). Compara-
tive study on credit card fraud detection based on dif-
ferent support vector machines. Intelligent Data Anal-
ysis, 25(1):105–119.
Li, Z., Huang, M., Liu, G., and Jiang, C. (2021b). A hybrid
method with dynamic weighted entropy for handling
the problem of class imbalance with overlap in credit
card fraud detection. Expert Syst. Appl., 175(Febru-
ary):114750.
Marco, G. et al. (2022). The role of diversity and ensem-
ble learning in credit card fraud detection. Adv. Data
Anal. Classif.
Mienye, I. D., Sun, Y., and Member, S. (2023). A deep
learning ensemble with data resampling for credit card
fraud detection. IEEE Access, 11(February):30628–
30638.
Petersen, K., Feldt, R., Mujtaba, S., and Mattsson, M.
(2008). Systematic mapping studies in software
engineering. In 12Th International Conference on
Evaluation and Assessment in Software Engineering,
page 10.
Pozzolo, A. D., Boracchi, G., Caelen, O., and Alippi, C.
(2018). Credit card fraud detection: A realistic model-
ing and a novel learning strategy. IEEE Trans. Neural
Networks Learn. Syst., 29(8):3784–3797.
Rakhshaninejad, M., Fathian, M., Amiri, B., and Yazdan-
jue, N. (2022). An ensemble-based credit card fraud
detection algorithm using an efficient voting strategy.
The Computer Journal, 65(8):1998–2015.
Randhawa, K., Loo, C. H. U. K., and Member, S. (2018).
Credit card fraud detection using adaboost and major-
ity voting. IEEE Access, 6:14277–14284.
Report, N. (October 2023). The world’s top card issuers and
merchant acquirers.
Sadgali, I., Sael, N., and Benabbou, F. (2021). Hu-
man behavior scoring in credit card fraud detection.
IAES International Journal of Artificial Intelligence,
10(3):698.
Salekshahrezaee, Z., Leevy, J. L., and Khoshgoftaar, T. M.
(2023). The effect of feature extraction and data sam-
pling on credit card fraud detection. J. Big Data.
Sharma, P., Banerjee, S., Tiwari, D., and Patni, J. C.
(2021). Machine learning model for credit card fraud
detection- a comparative analysis. The International
Arab Journal of Information Technology, 18(6):789–
796.
Taha, A. A. and Malebary, S. J. (2020). An intelligent ap-
proach to credit card fraud detection using an opti-
mized light gradient boosting machine. IEEE access,
8:25579–25587.
Tayebi, M. and El, S. (2022). Performance analysis
of metaheuristics based hyperparameters optimization
for fraud transactions detection. Evol. Intell., page
0123456789.
Trisanto, D. (2021). Modified focal loss in imbalanced xg-
boost for credit card fraud detection. Int. J. Ind. Eng.
Syst., 14(4):350–358.
Wu, Y., Xu, Y., and Li, J. (2019). Feature construction for
fraudulent credit card cash-out detection. Decis. Sup-
port Syst., page 113155.
Yara, A., Albatul, A., and A, R. M. (2020). A financial
fraud detection model based on lstm deep learning
technique. J. Appl. Secur. Res., 0(0):1–19.
Zheng, L., Liu, G., Yan, C., Jiang, C., Zhou, M., and Li,
M. (2020). Improved tradaboost and its application
to transaction fraud detection. IEEE Transactions on
Computational Social Systems, 7(5):1304–1316.
Zhu, H., Liu, G., Zhou, M., Xie, Y., and Abusorrah, A.
(2020). Optimizing weighted extreme learning ma-
chines for imbalanced classification and application to
credit card fraud detection. Neurocomputing, 407:50–
62.
Reviewing Machine Learning Techniques in Credit Card Fraud Detection
187