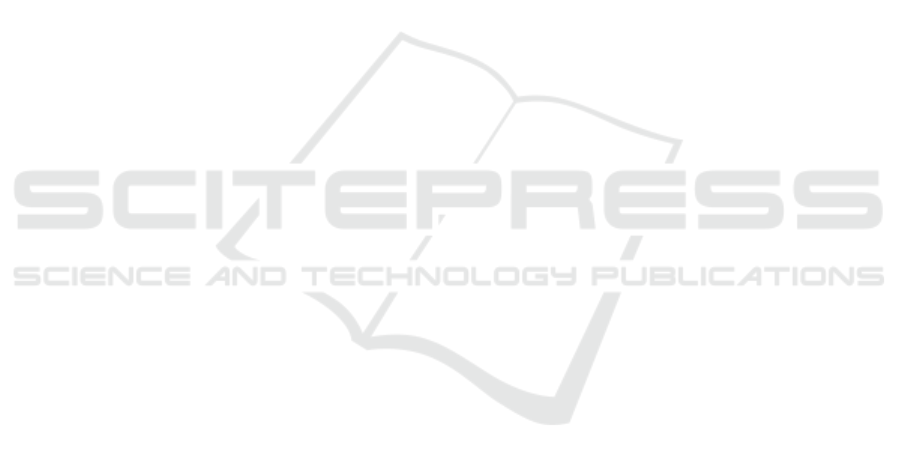
method was tested on two virtual datasets, includ-
ing scenarios with occlusions, and a real-world in-
door dataset, showing promising results even under
challenging conditions such as occlusions and noise.
The proposed framework achieved, expectedly, a bet-
ter accuracy in the occluded virtual dataset than on the
real-world indoor dataset, due to the noisy nature of
the measurements (that is not replicated in the virtual
datasets). Still, these results demonstrate the poten-
tial of the approach for future applications in indus-
trial environments, where it can significantly enhance
efficiency and safety. Future work will include the ac-
quisition of a new dataset in an industrial setting, with
further validation of the method proposed.
ACKNOWLEDGEMENTS
This work has been supported by the Por-
tuguese Foundation for Science and Technology
(FCT) through grant UIDB/00048/2020 (DOI
10.54499/UIDB/00048/2020) and by Agenda
“GreenAuto: Green innovation for the Automotive
Industry”, with reference PRR-C644867037-
00000013.
REFERENCES
Cao, Z., Sheikh, Y., and Banerjee, N. K. (2016). Real-time
scalable 6DOF pose estimation for textureless objects.
In 2016 IEEE International Conference on Robotics
and Automation (ICRA).
Chen, X. and Guhl, J. (2018). Industrial Robot Control with
Object Recognition based on Deep Learning. Proce-
dia CIRP, 76:149–154.
Drost, B., Ulrich, M., Navab, N., and Ilic, S. (2010). Model
globally, match locally: Efficient and robust 3D object
recognition. In 2010 IEEE Computer Society Confer-
ence on Computer Vision and Pattern Recognition.
Dwyer, B., Nelson, J., Hansen, T., et al. (2024). Roboflow
(version 1.0) [software]. https://roboflow.com.
Fragapane, G., De Koster, R., Sgarbossa, F., and Strandha-
gen, J. O. (2021). Planning and control of autonomous
mobile robots for intralogistics: Literature review and
research agenda. European Journal of Operational
Research, 294(2):405–426.
Gorschl
¨
uter, F., Rojtberg, P., and P
¨
ollabauer, T. (2022). A
Survey of 6D Object Detection Based on 3D Mod-
els for Industrial Applications. Journal of Imaging,
8(3):53.
He, K., Zhang, X., Ren, S., and Sun, J. (2016). Deep Resid-
ual Learning for Image Recognition. In 2016 IEEE
Conference on Computer Vision and Pattern Recogni-
tion (CVPR), Las Vegas, NV, USA. IEEE.
Hinterstoisser, S., Lepetit, V., Ilic, S., Holzer, S., Bradski,
G., Konolige, K., and Navab, N. (2013). Model Based
Training, Detection and Pose Estimation of Texture-
Less 3D Objects in Heavily Cluttered Scenes. In 11th
Asian Conference on Computer Vision. Springer.
Hinterstoisser, S., Lepetit, V., Ilic, S., Holzer, S., Kono-
lige, K., Bradski, G., and Navab, N. (2012). Techni-
cal Demonstration on Model Based Training, Detec-
tion and Pose Estimation of Texture-Less 3D Objects
in Heavily Cluttered Scenes. In Computer Vision –
ECCV 2012. Workshops and Demonstrations, volume
7585. Springer Berlin Heidelberg, Berlin, Heidelberg.
Hinterstoisser, S., Lepetit, V., Rajkumar, N., and Konolige,
K. (2016). Going Further with Point Pair Features.
volume 9907, pages 834–848. arXiv:1711.04061 [cs].
Jocher, G., Chaurasia, A., and Qiu, J. (2023). Ultralytics
yolov8. https://github.com/ultralytics/ultralytics. Ac-
cessed: 2024-06-5.
Knitt, M., Schyga, J., Adamanov, A., Hinckeldeyn, J., and
Kreutzfeldt, J. (2022). PalLoc6D-Estimating the Pose
of a Euro Pallet with an RGB Camera based on Syn-
thetic Training Data. https://doi.org/10.15480/336.
4470.
Li, Y., Wang, G., Ji, X., Xiang, Y., and Fox, D. (2020).
DeepIM: Deep Iterative Matching for 6D Pose Esti-
mation. International Journal of Computer Vision,
128(3):657–678. arXiv:1804.00175 [cs].
Li, Z., Wang, G., and Ji, X. (2019). CDPN: Coordinates-
Based Disentangled Pose Network for Real-Time
RGB-Based 6-DoF Object Pose Estimation. In 2019
IEEE/CVF International Conference on Computer Vi-
sion (ICCV).
Luong, M.-T., Pham, H., and Manning, C. D. (2015). Ef-
fective approaches to attention-based neural machine
translation. arXiv preprint arXiv:1508.04025.
Peng, S., Zhou, X., Liu, Y., Lin, H., Huang, Q., and Bao, H.
(2022). PVNet: Pixel-Wise Voting Network for 6DoF
Object Pose Estimation. IEEE Transactions on Pat-
tern Analysis and Machine Intelligence, 44(6):3212–
3223.
Srinivas, A., Lin, T., Parmar, N., Shlens, J., Abbeel, P., and
Vaswani, A. (2021). Bottleneck transformers for vi-
sual recognition. CoRR, abs/2101.11605.
Wang, C., Xu, D., Zhu, Y., Mart
´
ın-Mart
´
ın, R., Lu, C.,
Fei-Fei, L., and Savarese, S. (2019). DenseFusion:
6D Object Pose Estimation by Iterative Dense Fusion.
arXiv:1901.04780 [cs].
Wang, C.-Y., Liao, H.-Y. M., Wu, Y.-H., Chen, P.-Y., Hsieh,
J.-W., and Yeh, I.-H. (2020). CSPNet: A new back-
bone that can enhance learning capability of cnn. In
Proceedings of the IEEE/CVF conference on com-
puter vision and pattern recognition workshops, pages
390–391.
Zhao, H., Shi, J., Qi, X., Wang, X., and Jia, J. (2017). Pyra-
mid Scene Parsing Network. arXiv:1612.01105 [cs].
ICINCO 2024 - 21st International Conference on Informatics in Control, Automation and Robotics
352