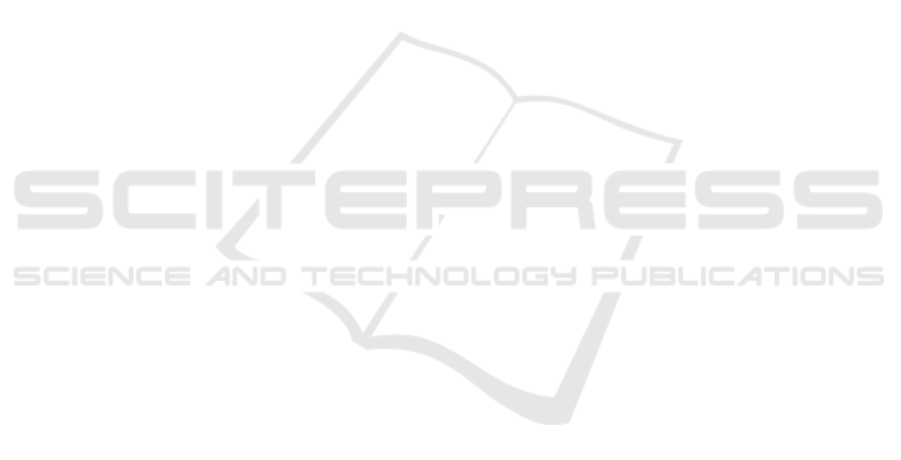
Bouckaert, R. R., Frank, E., Hall, M., Kirkby, R., Reute-
mann, P., Seewald, A., and Scuse, D. (2016). Weka
manual for version 3-9-1. University of Waikato:
Hamilton, New Zealand, pages 1–341.
Brandt, H. (2001). Correlation analysis of fitness land-
scapes.
Breiman, L. and Cutler, R. (2001). Random forests machine
learning [j]. journal of clinical microbiology, 2:199–
228.
Cervantes, J., Garcia-Lamont, F., Rodr
´
ıguez-Mazahua, L.,
and Lopez, A. (2020). A comprehensive survey on
support vector machine classification: Applications,
challenges and trends. Neurocomputing, 408:189–
215.
Cortes, C. (1995). Support-vector networks. Machine
Learning.
Daglayan, H. and Karakaya, M. (2016). The impact of
crossover and mutation operators on a ga solution for
the capacitated vehicle routing problem. Universal
Journal of Engineering Science, 4(3):39–44.
Goldberg, D. (1989). Genetic algorithms in search,
optimization and machine learning. reading, ma:
Addison-wesley professional.
Hassan, A. and Pillay, N. (2022). Automated design of
hybrid metaheuristics: A fitness landscape analysis.
In 2022 IEEE Congress on Evolutionary Computation
(CEC), pages 1–8. IEEE.
Huang, J., Wen, J., Chen, L., and Liu, H.-L. (2023). Trans-
fer learning based evolutionary algorithm framework
for multi-objective optimization problems. Applied
Intelligence, pages 1–20.
Jiang, M., Huang, Z., Qiu, L., Huang, W., and Yen, G. G.
(2017). Transfer learning-based dynamic multiobjec-
tive optimization algorithms. IEEE Transactions on
Evolutionary Computation, 22(4):501–514.
Jiang, M., Wang, Z., Hong, H., and Yen, G. G. (2020a).
Knee point-based imbalanced transfer learning for dy-
namic multiobjective optimization. IEEE Transac-
tions on Evolutionary Computation, 25(1):117–129.
Jiang, M., Wang, Z., Qiu, L., Guo, S., Gao, X., and Tan,
K. C. (2020b). A fast dynamic evolutionary multiob-
jective algorithm via manifold transfer learning. IEEE
Transactions on Cybernetics, 51(7):3417–3428.
Liu, Z. and Wang, H. (2021). Improved population pre-
diction strategy for dynamic multi-objective optimiza-
tion algorithms using transfer learning. In 2021 IEEE
Congress on Evolutionary Computation (CEC), pages
103–110. IEEE.
Lu, G., Li, J., and Yao, X. (2011). Fitness-probability cloud
and a measure of problem hardness for evolutionary
algorithms. In Evolutionary Computation in Com-
binatorial Optimization: 11th European Conference,
EvoCOP 2011, Torino, Italy, April 27-29, 2011. Pro-
ceedings 11, pages 108–117. Springer.
Luke, S. (1998). ECJ evolutionary com-
putation library. Available for free at
http://cs.gmu.edu/∼eclab/projects/ecj/.
Lysgaard, J. (1997). Clarke & wright’s savings algorithm.
Department of Management Science and Logistics,
The Aarhus School of Business, 44.
Merkuryeva, G. and Bolshakovs, V. (2011). Benchmark fit-
ness landscape analysis. International Journal of Sim-
ulation Systems, Science and Technology, 12(2):38–
45.
Nyathi, T. and Pillay, N. (2021). On the transfer learn-
ing of genetic programming classification algorithms.
In Aranha, C., Mart
´
ın-Vide, C., and Vega-Rodr
´
ıguez,
M. A., editors, Theory and Practice of Natural Com-
puting, pages 47–58, Cham. Springer International
Publishing.
Russell, J. and Pillay, N. (2023). A selection hyper-heuristic
for transfer learning in genetic programming. In Pro-
ceedings of the Companion Conference on Genetic
and Evolutionary Computation, GECCO ’23 Com-
panion, pages 631–634, New York, NY, USA. Asso-
ciation for Computing Machinery.
Scheepers, D. and Pillay, N. (2021). A study of transfer
learning in a generation constructive hyper-heuristic
for one dimensional bin packing. In 2021 IEEE Sym-
posium Series on Computational Intelligence (SSCI),
pages 1–7.
Schonlau, M. and Zou, R. Y. (2020). The random forest
algorithm for statistical learning. The Stata Journal,
20(1):3–29.
Sen, P. C., Hajra, M., and Ghosh, M. (2020). Super-
vised classification algorithms in machine learning:
A survey and review. In Emerging Technology in
Modelling and Graphics: Proceedings of IEM Graph
2018, pages 99–111. Springer.
Sinaga, K. P. and Yang, M.-S. (2020). Unsupervised k-
means clustering algorithm. IEEE access, 8:80716–
80727.
Stadler, P. F. (2002). Fitness landscapes. In Biologi-
cal evolution and statistical physics, pages 183–204.
Springer.
Sun, Y., Ding, S., Zhang, Z., and Jia, W. (2021). An im-
proved grid search algorithm to optimize svr for pre-
diction. Soft Computing, 25:5633–5644.
Vanneschi, L., Pirola, Y., and Collard, P. (2006). A quanti-
tative study of neutrality in gp boolean landscapes. In
Proceedings of the 8th annual conference on Genetic
and evolutionary computation, pages 895–902.
Wright, S. et al. (1932). The roles of mutation, inbreeding,
crossbreeding, and selection in evolution.
Yadav, S. L. and Sohal, A. (2017). Comparative study
of different selection techniques in genetic algorithm.
International Journal of Engineering, Science and
Mathematics, 6(3):174–180.
Zhang, T.-T., Hao, G.-S., Lim, M.-H., Gu, F., and Wang, X.
(2023). A deep hybrid transfer learning-based evolu-
tionary algorithm and its application in the optimiza-
tion of high-order problems. Soft Computing, pages
1–12.
Zhuang, F., Qi, Z., Duan, K., Xi, D., Zhu, Y., Zhu, H.,
Xiong, H., and He, Q. (2020). A comprehensive sur-
vey on transfer learning. Proceedings of the IEEE,
109(1):43–76.
Zou, F., Chen, D., Liu, H., Cao, S., Ji, X., and Zhang, Y.
(2022). A survey of fitness landscape analysis for op-
timization. Neurocomputing, 503:129–139.
ECTA 2024 - 16th International Conference on Evolutionary Computation Theory and Applications
374