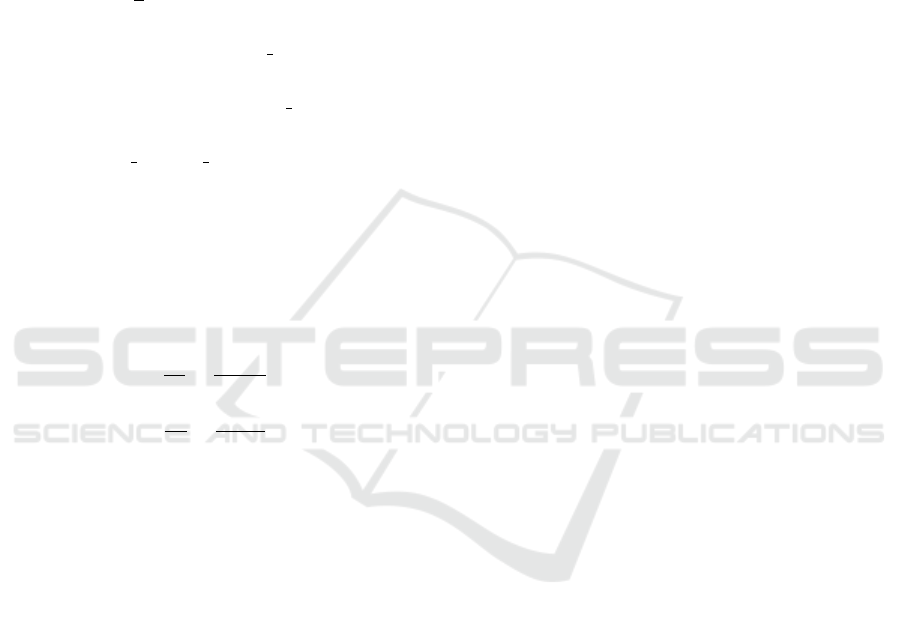
K(p
)
= 1/2pp2. The potential function is our ob-
jective function and it is through the gradient of the
potential that our state converges to the typical set.
The potential energy is calculated as the squared error
V (q) = 1/2(DGF)2 where D is the real forward im-
age, G is the propagator of the problem as described
above, and F is the current state of the HMC chain. At
each iteration, a new Hamiltonian resulting from new
kinetic and potential energies is calculated. The re-
sulting Hamiltonian evolves in time with the leapfrog
integration method.
For 0 ≤ n <
T
E
do
A ← A − E δV (q
n+
1
2
)δq
n
q
n+1
← q
n
+ E p
n+
1
2
A
n+
1
2
← A
n+
1
2
− 2δq(a
n
+ q)
End For.
Where T is the total time evolved and E is the
step size. This simple method allows us to evolve the
Hamiltonian in time following a path that minimizes
its action, as described by Hamilton equations. Note
that if K and V are scleronomic, Hamilton equations
reduce to
dq
dt
=
δK(p)
δp
(2)
d p
dt
=
δV (q)
δq
(3)
Which bears similarity to the leapfrog algorithm.
The forward image used for the HMC method was
of size 64 × 64 and was generated using the forward
model described above for 1, 3 and 50 sources. The
simplified single-source scenario was used to build
and tune hyperparameters for the HMC model. Hy-
perparameters in HMC govern the evolution of the
state and its convergence to the typical set. These
parameters are typically referred to as Temperature
and step size, where the former governs the amount
of chaos or variation introduced to the system at each
iteration, and the latter is the step size used in the
leapfrog integrator to calculate the variation of the
Hamiltonian at each integration step. One of the diffi-
culties of the HMC method is to use optimal parame-
ters for convergence, which depend on the typical set
and the nature of the problem. In addition to the ones
described above, it is also useful to find a good seed to
allow for faster convergence. To find the best values
for these parameters (T, step size, seed) an exhaustive
search over common parameter ranges was conducted
in the iris-cluster (Varrette et al., 2014). After find-
ing the best set of parameters, we run the HMC for
10,000 iterations for 1, 3 and 50 initial sources. To
compare the final states to the initial simulation we
perform a structural similarity test on the final states
smoothed with a Gaussian filter. For completeness,
we also compare the initial simulation and the final
HMC state with a full waveform inversion model ob-
tained using back projection.
2.3 Neural Networks approach
As we see the in GPR data (see Fig. 2 & 3) every pixel
intensity defines the reflectivity and shows the object
property. It means we have to predict the exact values
of these pixels to find the objects. Traditionally U-net
is used for segmentation and assigns class labels to
each pixel. But in our case, it is more of a regression
problem to predict the reflectivity values of pixels.
• Neural Networks Architecture: The network ar-
chitecture, inspired by U-net as illustrated in Fig.
1, consists of two main pathways: PATH A has
elements that compress, control or confine: re-
gressive, directive and enclosed (left side of the
figure), PATH B has elements that open up, en-
courage, and set free: progressive, persuasive and
most encompassing (right side of the figure). The
compressive path is implemented with the general
architecture of a convolutional network. It suc-
cessively performs two convolutions of 3x3 with
the same padding and uses the ReLU activation
function; and then a max pooling of 2x2 with
a stride of 2 for down sampling. Thus, in the
down-sampling processes, the number of chan-
nels is doubled while the size of the feature maps
is reduced. Steps of the expansive path are up-
sampling the feature map, then followed by a con-
volution operation with a 2 x 2 filter, which is also
known as up-convolution that reduces the num-
ber of channels in the feature map by two at that
step. Which is then concatenated to a feature map
extracted from the compressive path at the same
level but with precise cropping. Two 3 x 3 con-
volutions and ReLU are next to each other, one
convolution is followed by ReLU. The cropping
is needed because the convolution operation crops
out a portion of the border in each instance of the
feature map. The network ends with a 1x1 convo-
lution layer at the last stage that transforms each
64-element vector into the output. Blue rectan-
gles denote the multi-channel feature maps. Next
to each rectangular figure, there is a number that
represents the number of channels; the x-y dimen-
sions are also provided in the lower left corner.
White rectangles are the duplicative feature maps
as indicated below. Some of the operations are
IC3Com 2024 - International Conference on Cognitive & Cloud Computing
156