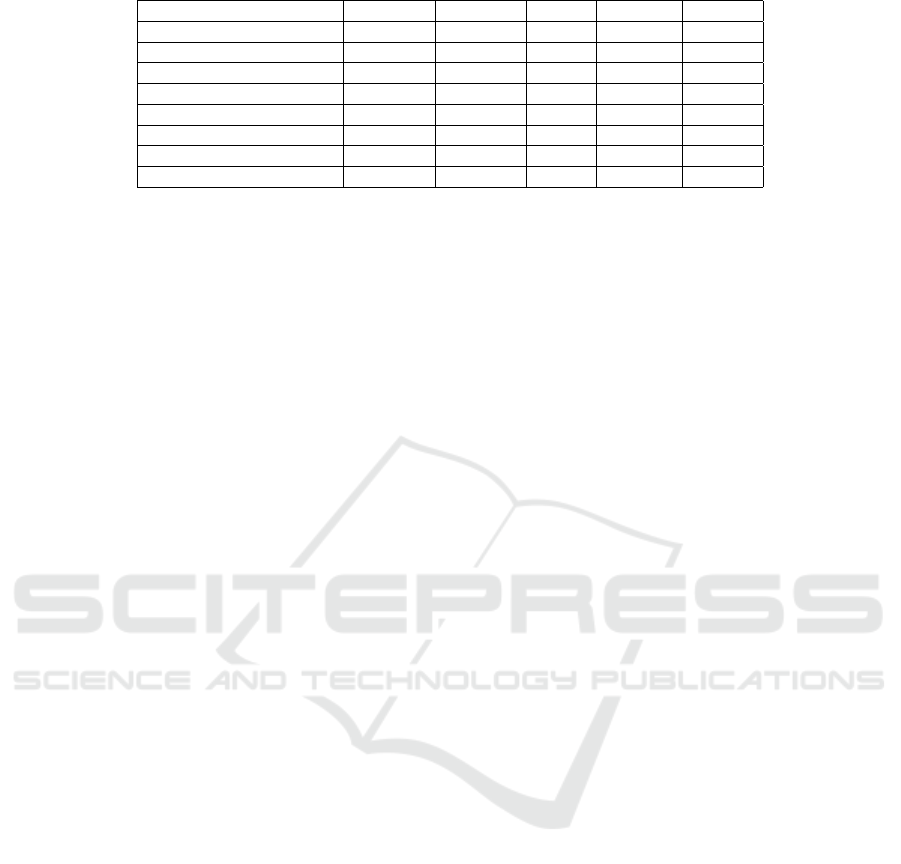
Table 1: Prediction results and comparative analysis of models
Name of the Model Accuracy Precision Recall F1-score Support
Logistic Regression 97.24% 0.98 0.99 0.99 391
Decision Tree 99.00% 1 0.99 0.99 391
Random Forest Classifier 99.50% 0.99 1 1 391
KNN 97.49% 0.99 0.98 0.99 391
SVC 97.99% 0.98 1 0.99 391
Adaboost Classifier 100.00% 1 1 1 391
LSTM 97.99% 0.98 1 0.99 391
XGB Classifier 100.00% 1 1 1 391
ity in Indian rivers by means of machine learning,
specifically aimed at promoting sustainable resource
management and the equivalent water parameters that
are needed to predict water quality. The application
of sophisticated ML models such as Logistic Regres-
sion, Decision Tree, Random forest classifier, KNN,
SVC, AdaBoost Classifier, LSTM, and XGB Classi-
fier, shows the dedication to tackling the challenges
involved in understanding the intricate dynamics of
water quality. The aforementioned models have been
thoroughly evaluated using a comprehensive dataset,
which includes a wide variety of water samples across
diverse rivers of India. The results of these evalu-
ations demonstrate the effectiveness of the models.
The implementation results depict that the AdaBoost
and XGB Classifier outperform with 100% accuracy.
Whereas the other models such as Logistic Regres-
sion, Decision Tree, Random Forest classifier, KNN,
SVC, and LSTM predict the water quality with an
accuracy of 97.24%, 99%, 99.50%, 97.49%, 97.99%
and 97.99% respectively.
In addition to that, various regional disparities pa-
rameters in the dataset are highlighted in this paper,
providing an insight into the intricate environmental
elements that affect water quality in different regions
of India. The water quality prediction results also give
an insight view of the different types of water quality
in the different regions of India. These predictions
can help in the perspective of farming areas to grow
different types of crops in suitable areas. Future work
may involve exploring more datasets and the effect of
the ensemble approach on ML models.
ACKNOWLEDGEMENTS
The authors wish to thank Mr. N. L. Chauhan for
guiding about water parameters. Thanks are also
to the management of Nirma University and Pandit
Deendayal Energy University for providing resources
to carry out research.
REFERENCES
Ewaid, S. H., Kadhum, S. A., Abed, S. A., and Salih, R. M.
(2019). Development and evaluation of irrigation wa-
ter quality guide using iwqg v. 1 software: A case
study of al-gharraf canal, southern iraq. Environmen-
tal technology & innovation, 13:224–232.
Ewuzie, U., Bolade, O. P., and Egbedina, A. O. (2022).
Application of deep learning and machine learn-
ing methods in water quality modeling and predic-
tion: a review. Current trends and advances in
computer-aided intelligent environmental data engi-
neering, pages 185–218.
Ibrahim, H., Yaseen, Z. M., Scholz, M., Ali, M., Gad, M.,
Elsayed, S., Khadr, M., Hussein, H., Ibrahim, H. H.,
Eid, M. H., et al. (2023). Evaluation and prediction of
groundwater quality for irrigation using an integrated
water quality indices, machine learning models and
gis approaches: A representative case study. Water,
15(4):694.
Khoi, D. N., Quan, N. T., Linh, D. Q., Nhi, P. T. T., and
Thuy, N. T. D. (2022). Using machine learning models
for predicting the water quality index in the la buong
river, vietnam. Water, 14(10):1552.
Meireles, A. C. M., Andrade, E. M. d., Chaves, L. C. G.,
Frischkorn, H., and Crisostomo, L. A. (2010). A new
proposal of the classification of irrigation water. Re-
vista Ci
ˆ
encia Agron
ˆ
omica, 41:349–357.
Modaresi, F. and Araghinejad, S. (2014). A comparative
assessment of support vector machines, probabilistic
neural networks, and k-nearest neighbor algorithms
for water quality classification. Water resources man-
agement, 28:4095–4111.
Sakaa, B., Elbeltagi, A., Boudibi, S., Chaffa
¨
ı, H., Islam, A.
R. M. T., Kulimushi, L. C., Choudhari, P., Hani, A.,
Brouziyne, Y., and Wong, Y. J. (2022). Water qual-
ity index modeling using random forest and improved
smo algorithm for support vector machine in saf-saf
river basin. Environmental Science and Pollution Re-
search, 29(32):48491–48508.
Sakizadeh, M. and Mirzaei, R. (2016). A comparative
study of performance of k-nearest neighbors and sup-
port vector machines for classification of groundwater.
Journal of Mining and Environment, 7(2):149–164.
Sharma, A., Jain, A., Gupta, P., and Chowdary, V. (2020).
Machine learning applications for precision agricul-
ture: A comprehensive review. IEEE Access, 9:4843–
4873.
Predictive Modeling of Water Quality in Indian Rivers: A Machine Learning Approach for Sustainable Resource Management
173