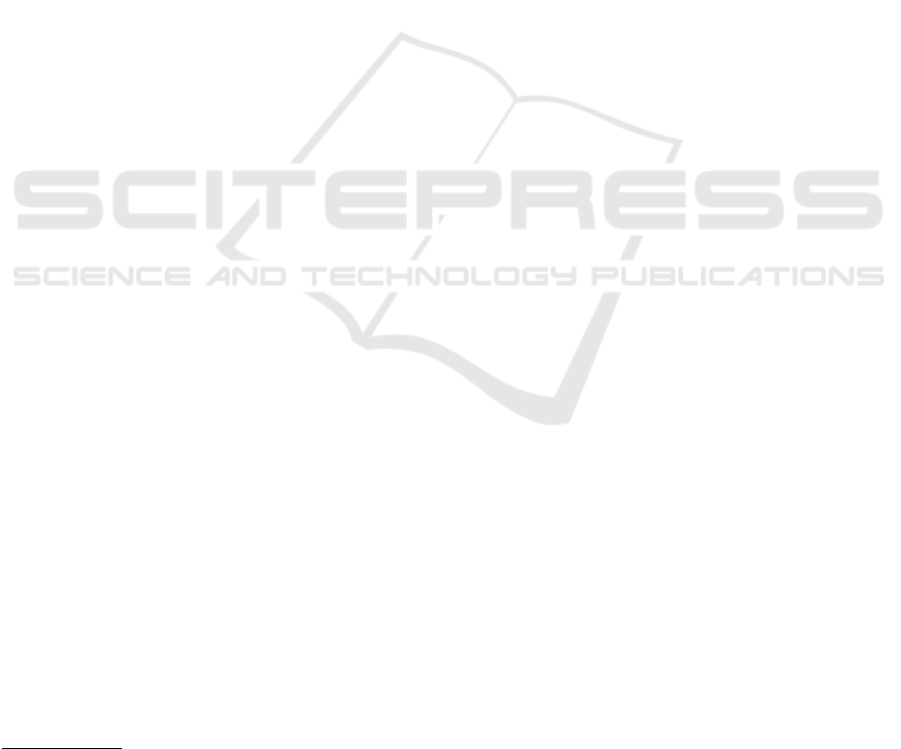
the National Food Security Act (NFSA’13), offering
highly subsidized food grains to 2.52 crore individu-
als in accordance with Article 13 of the NFSA. Effi-
cient monitoring and quick searching of ration card-
related information have become essential, necessitat-
ing the adoption of new technology for real-time data
retrieval. Elasticsearch, coupled with Kibana
2
as its
monitoring and visualization tool, emerges as a suit-
able solution, enabling faster and optimized perfor-
mance compared to traditional RDBMS systems.
The study aims to evaluate and analyze the real-
time performance of Elasticsearch compared to an
RDBMS system. The development of a web-based
application facilitates the easy retrieval of PDS-
related ration cards through Elasticsearch indexing.
Additionally, a dynamic dashboard incorporating Key
Performance Indicator (KPI)-based data visualization
using Kibana has been created. The proposed sys-
tem’s objectives include systematically recording and
maintaining ration cardholder details for Assam on
a unified website. Furthermore, the system aims to
expedite the generation of detailed reports for ration
cardholders whenever required.
2 RELATED WORK
Big data analysis serves various objectives, includ-
ing the classification of conversation topics to iden-
tify trending discussions. Given that the main aim of
this research is to devise a text search and big data
analysis system within the DevOps framework, it is
crucial to establish a clear definition. Wahaballa et
al.(Wahaballa et al., 2015) described this approach
as a software development methodology designed to
enhance communication, collaboration, and integra-
tion between development and operations teams, aim-
ing to address critical challenges such as the fear of
change and the risks associated with development.
Historically, the separation between teams dedi-
cated to swift feature delivery and those responsible
for ensuring system stability has led to discord and
decreased productivity. DevOps emerged to address
conflicts, offering swift and continuous assessment
through the facilitation and integration of collabora-
tion among development, operations, and teams re-
sponsible for quality assurance. But building, log-
ging, and monitoring tools are essential to automating
the DevOps process. Akshaya et al.(Akshaya et al.,
2015) introduces several monitoring tools commonly
2
Getting Started with the elastic stack
technology (2022) [Online]. Available
: https://www.elastic.co/guide/en/elastic-
stack/7.17/installing-elastic-stack.html
employed in DevOps practices. Some of them are
briefly described as follows.
• Splunk serves as a tool for analyzing and storing
big data, providing support for numerous related
applications and plugins.
• Loggly offers logging and log data management
through its cloud-based software-as-a-service
(SaaS) solutions. Unlike other similar tools fo-
cused on identifying system issues, Loggly em-
phasizes pinpointing the source of the problem.
• Graphite proves to be a scalable solution for stor-
ing data, generating graphs from time-lapse data,
and real-time monitoring of performance parame-
ters.
• Sumo Logic stands out as a cloud-based SaaS
security platform designed to process logs and
derive real-time insights, proving valuable for
promptly analyzing and addressing challenges
within the infrastructure.
• Cacti is designed as an application capable of stor-
ing system data and presenting real-time graphics
derived from it.
• Logstash, a component of the Elastic Stack, excels
in processing extensive volumes of log files and
extracting valuable insights from them.
• Kibana, an integral component within the Elas-
tic ecosystem, provides interactive visualization
of data on dashboards that users can customize ac-
cording to their needs.
Bangnasco et al.(Bagnasco et al., 2015) in their
work, describes ELK stack, comprised of Elastic-
search, Logstash, and Kibana, as a monitoring system
utilized for Infrastructure as a Service (IaaS) and sci-
entific applications in the cloud, leveraging the Elas-
ticsearch ecosystem. The monitoring system is used
to display data from a cloud-based system and store
it in a SQL database, then feed it to an Elasticsearch
node via the Logstash plugin. Each application pos-
sesses its unique Kibana dashboard for monitoring
and visualization. Nevertheless, the success of the
elastic stack-based solution prompted the team to con-
template the elimination of the SQL middleware layer
from the system. Zamfir et al.(Zamfir et al., 2019) in
their paper described the development of an Elastic-
search monitoring system that uses machine learning
techniques to effectively identify and stop errors at
scale. Takase et al. (Takase et al., 2017) in their work,
present a solution based on the strategy used by the
CERN cloud group. This solution comprises devel-
oping an Elasticsearch plugin to control data access
based on user identity, allowing individual users to
see personalized Kibana dashboards for monitoring
IC3Com 2024 - International Conference on Cognitive & Cloud Computing
6